Feature Reduction of Multi-scale LBP for Texture Classification
2015 INTERNATIONAL CONFERENCE ON INTELLIGENT INFORMATION HIDING AND MULTIMEDIA SIGNAL PROCESSING (IIH-MSP)(2015)
Abstract
Local binary pattern (LBP) is a simple yet powerful texture descriptor modeling the relationship of pixels to their local neighborhood. By considering multiple neighborhood radii, multi-scale LBP (MS-LBP) is derived. For MS-LBP generation, different scales LBP histograms are first extracted separately, and then combined in concatenate or joint way, resulting in a one-dimensional or multi-dimensional histogram, respectively. Concatenate MS-LBP has low feature dimension but loses some important discriminative information, while joint MS-LBP performs well but suffers high feature dimension. In this work, based on the similarity between different scales patterns and the sparsity of joint MS-LBP histogram, a feature reduction method for joint MS-LBP is proposed. Experiments on Outex and CURet show that the proposed method and its extension have performance comparable to the original joint MS-LBP but have lower feature dimension.
MoreTranslated text
Key words
Local binary pattern (LBP), multi-scale LBP (MS-LBP), feature reduction, texture classification
AI Read Science
Must-Reading Tree
Example
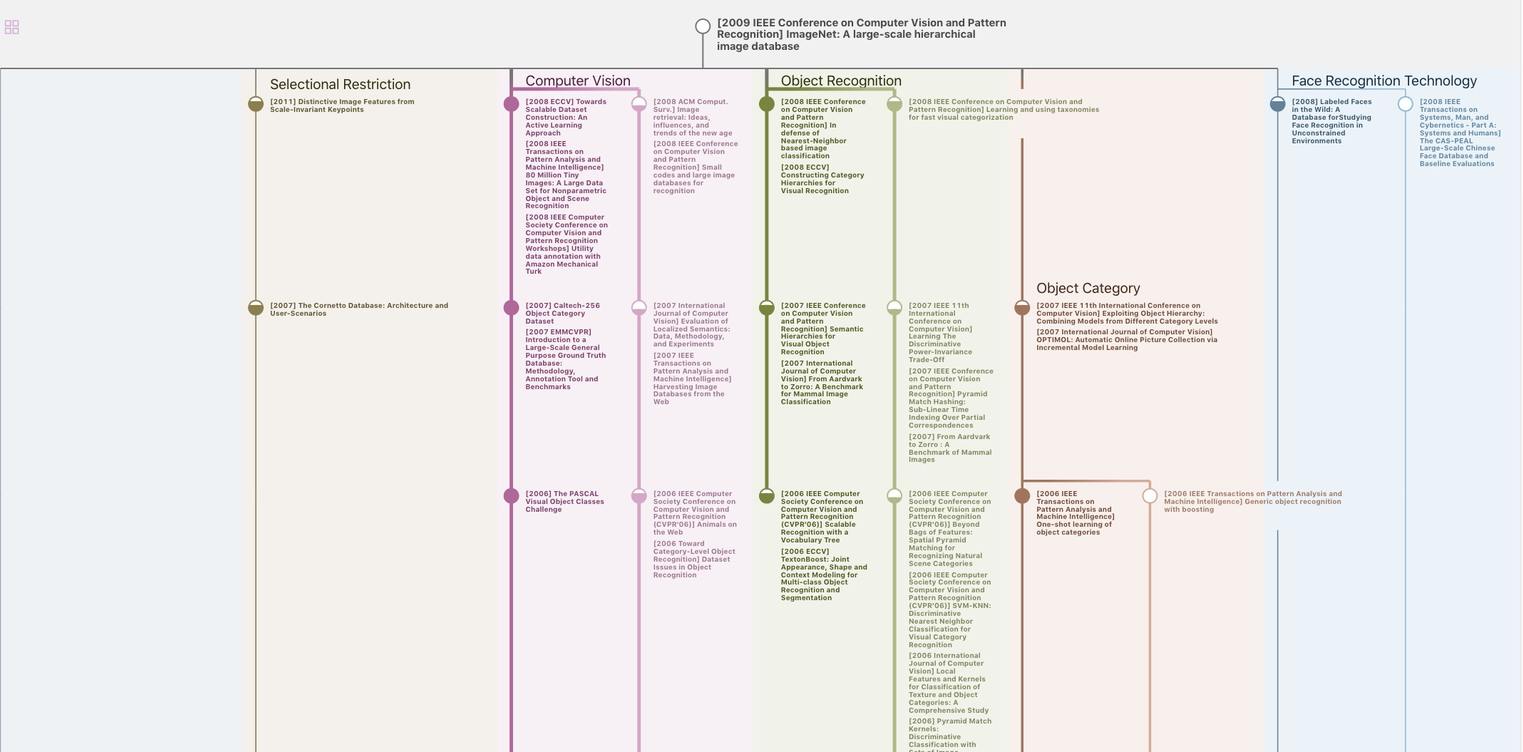
Generate MRT to find the research sequence of this paper
Chat Paper
Summary is being generated by the instructions you defined