Elliptical Slice Sampling with Expectation Propagation.
UAI'16: Proceedings of the Thirty-Second Conference on Uncertainty in Artificial Intelligence(2016)
摘要
Markov Chain Monte Carlo techniques remain the gold standard for approximate Bayesian inference, but their practical issues — including onerous runtime and sensitivity to tuning parameters — often lead researchers to use faster but typically less accurate deterministic approximations. Here we couple the fast but biased deterministic approximation offered by expectation propagation with elliptical slice sampling, a state-of-the-art MCMC method. We extend our hybrid deterministic-MCMC method to include recycled samples and analytical slices, and we rigorously prove the validity of each enhancement. Taken together, we show that these advances provide an order of magnitude gain in efficiency beyond existing state-of-the-art sampling techniques in Bayesian classification and multivariate gaussian quadrature problems.
更多查看译文
AI 理解论文
溯源树
样例
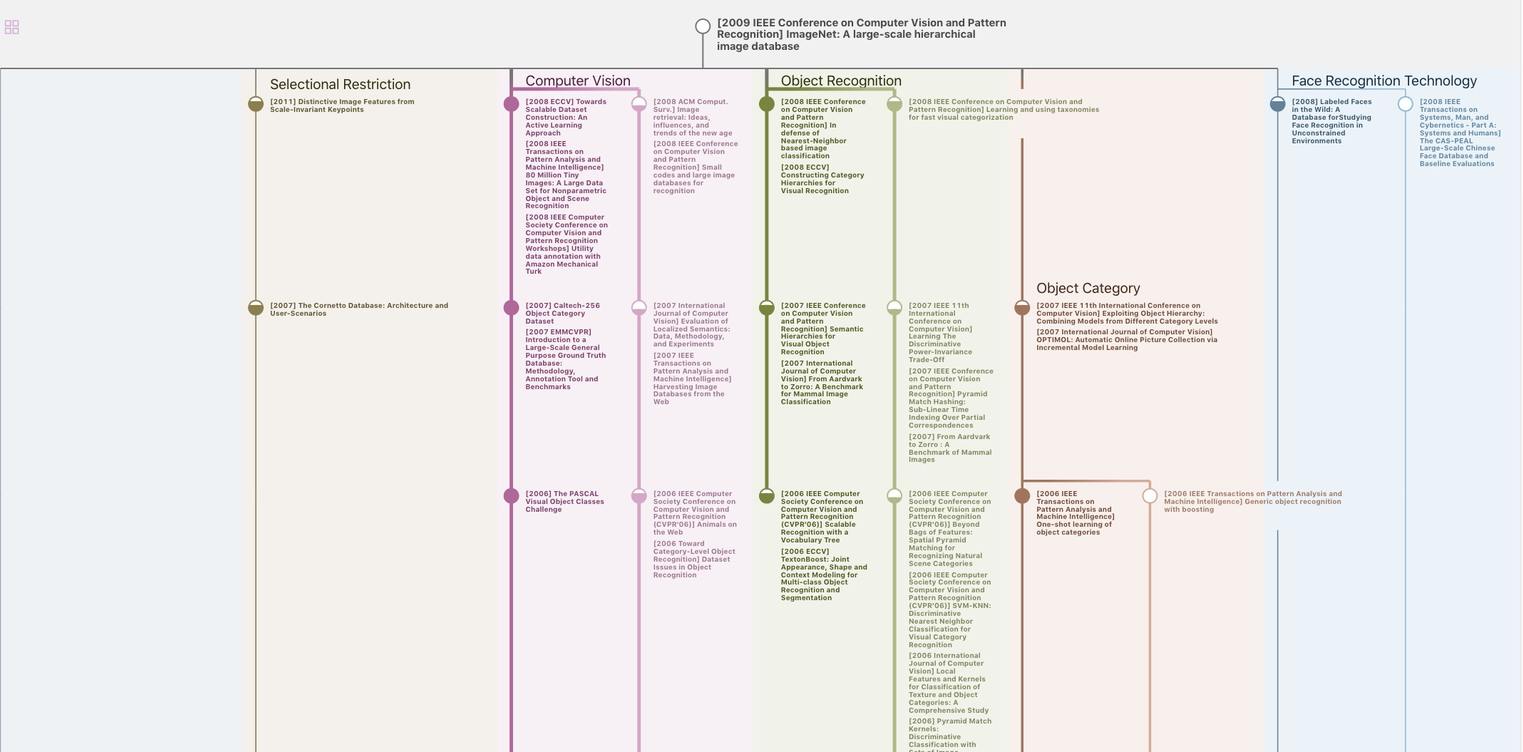
生成溯源树,研究论文发展脉络
Chat Paper
正在生成论文摘要