Monte-Carlo Tree Search for Simulation-based Strategy Analysis
FDG(2019)
摘要
Games are often designed to shape player behavior in a desired way; however, it can be unclear how design decisions affect the space of behaviors in a game. Designers usually explore this space through human playtesting, which can be time-consuming and of limited effectiveness in exhausting the space of possible behaviors. In this paper, we propose the use of automated planning agents to simulate humans of varying skill levels to generate game playthroughs. Metrics can then be gathered from these playthroughs to evaluate the current game design and identify its potential flaws. We demonstrate this technique in two games: the popular word game Scrabble and a collectible card game of our own design named Cardonomicon. Using these case studies, we show how using simulated agents to model humans of varying skill levels allows us to extract metrics to describe game balance (in the case of Scrabble) and highlight potential design flaws (in the case of Cardonomicon).
更多查看译文
关键词
Real-Time Strategy Games,Behavior Trees,Player Modeling,General Game Playing,Game Culture
AI 理解论文
溯源树
样例
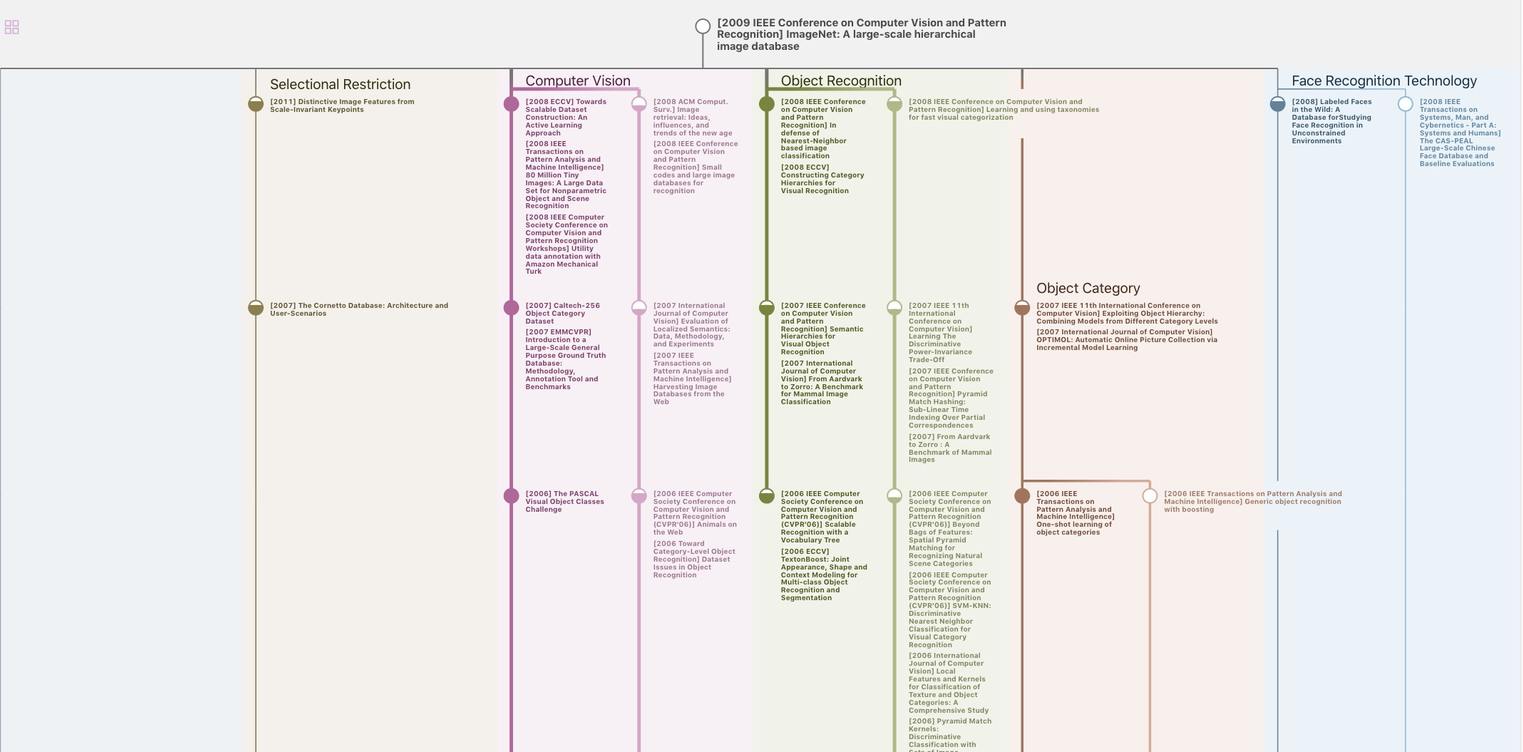
生成溯源树,研究论文发展脉络
Chat Paper
正在生成论文摘要