Semantic Word Embedding Neural Network Language Models For Automatic Speech Recognition
2016 IEEE International Conference on Acoustics, Speech and Signal Processing (ICASSP)(2016)
摘要
Semantic word embeddings have become increasingly important in natural language processing tasks over the last few years. This popularity is due to their ability to easily capture rich semantic information through a distributed representation and the availability of fast and scalable algorithms for learning them from large text corpora. State-of-the-art neural network language models (NNLMs) used in automatic speech recognition (ASR) and natural language processing also learn word embeddings optimized to model local N-gram dependencies given training text but are not optimized to capture semantic information. We hypothesize that semantic word embeddings provide diverse information compared to the word embeddings learned by NNLMs. We propose novel feedforward NNLM architectures that incorporate semantic word embeddings. We apply the resulting NNLMs to ASR on broadcast news and show improvements in both perplexity and word error rate.
更多查看译文
关键词
Word embeddings,neural networks,language modeling,automatic speech recognition,representation learning
AI 理解论文
溯源树
样例
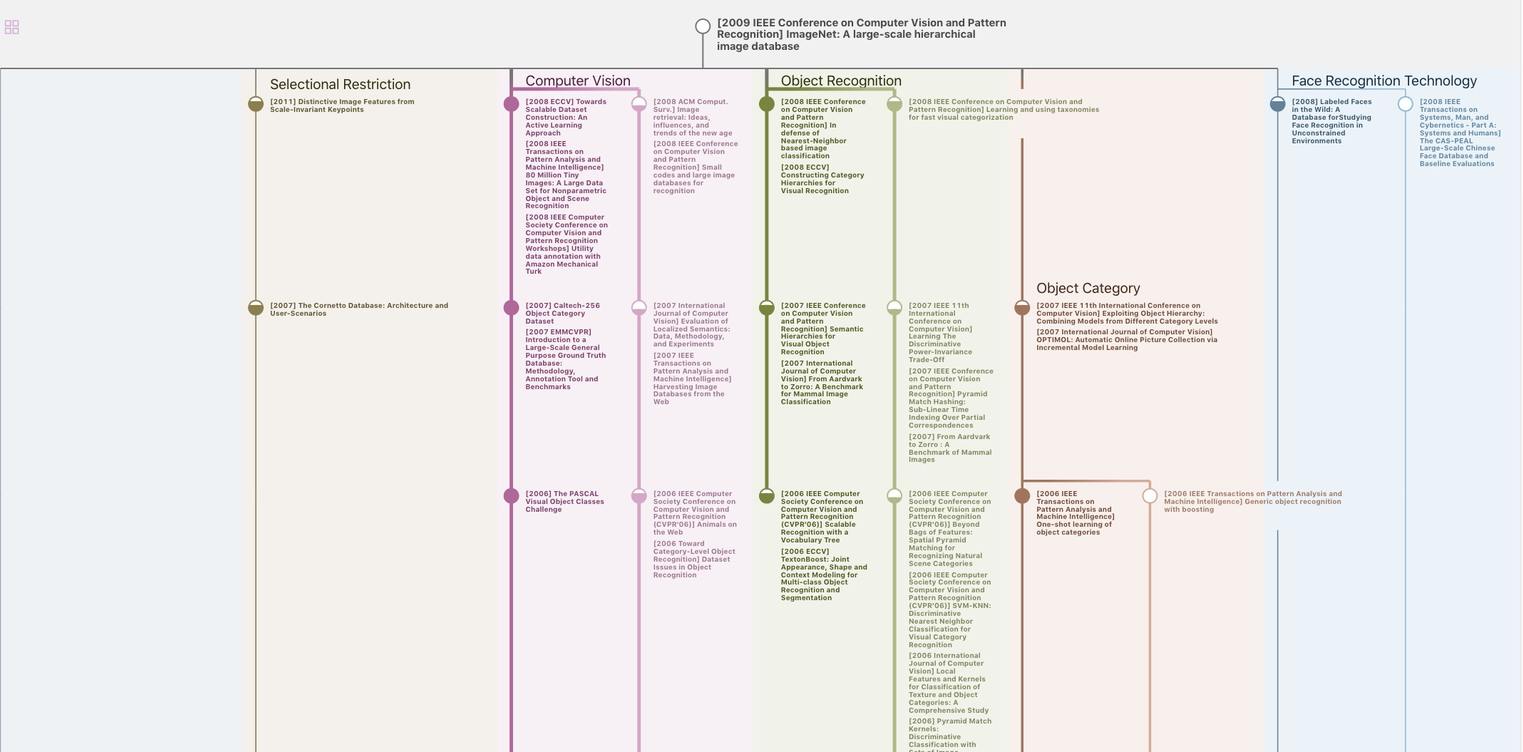
生成溯源树,研究论文发展脉络
Chat Paper
正在生成论文摘要