Biases in Automated Music Playlist Generation: A Comparison of Next-Track Recommending Techniques
UMAP(2016)
摘要
Playlist generation is a special form of music recommendation where the problem is to create a sequence of tracks to be played next, given a number of seed tracks. In academia, the evaluation of playlisting techniques is often done by assessing with the help of information retrieval measures if an algorithm is capable of selecting those tracks that also a human would pick next. Such approaches however cannot capture other factors, e.g., the homogeneity of the tracks that can determine the quality perception of playlists. In this work, we report the results of a multi-metric comparison of different academic approaches and a commercial playlisting service. Our results show that all tested techniques generate playlists with certain biases, e.g., towards very popular tracks, and often create playlists continuations that are quite different from those that are created by real users.
更多查看译文
AI 理解论文
溯源树
样例
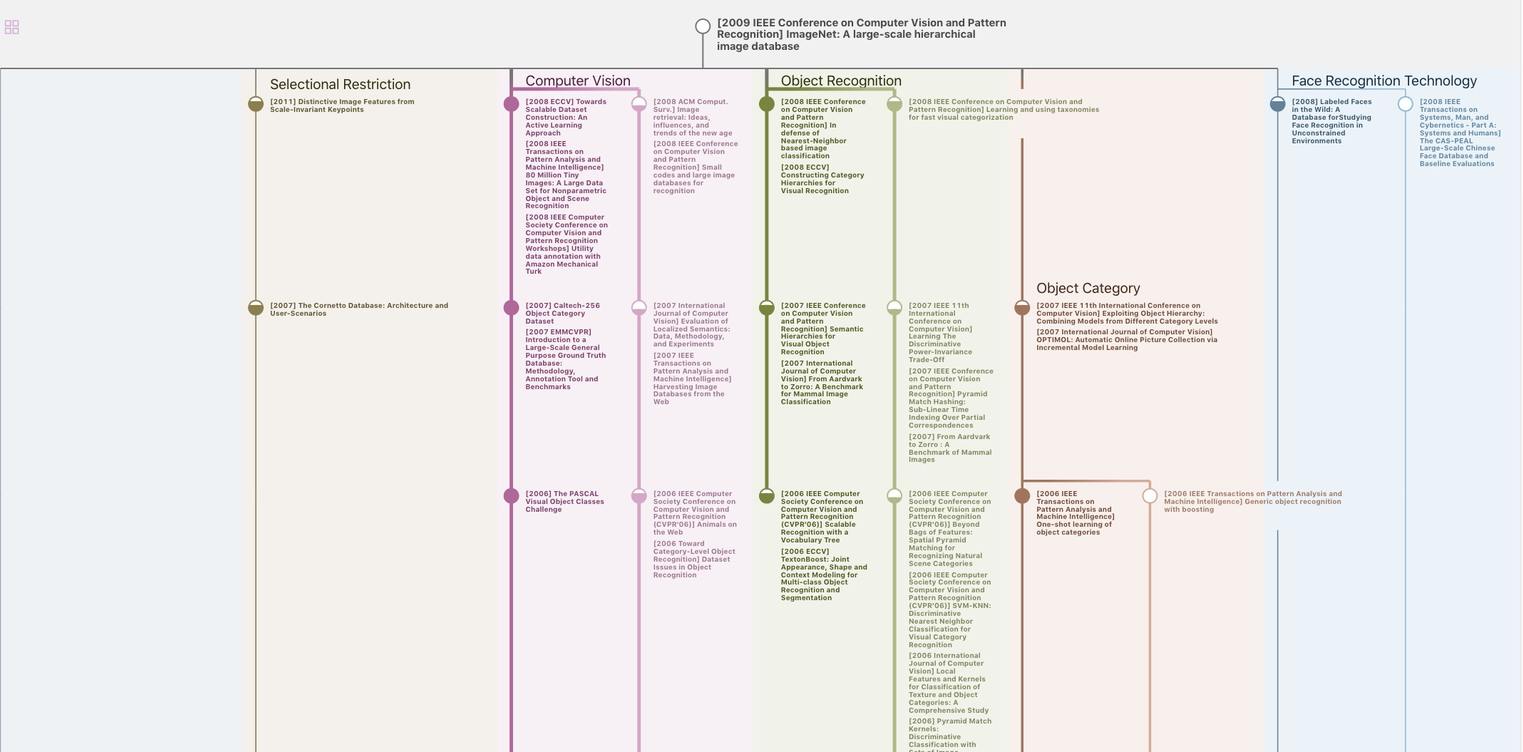
生成溯源树,研究论文发展脉络
Chat Paper
正在生成论文摘要