Hyperspectral Image Classification With Polynomial Laplacian Embedding
INTELLIGENT COMPUTING METHODOLOGIES, ICIC 2016, PT III(2016)
摘要
Hyperspectral remote sensing has drawn great interests in earth observation, since contiguous spectrum can provide rich information of ground objects. However, such numerous bands also pose great challenges to efficient processing of hyperspectral image (HSI). Manifold learning, as a promising tool for nonlinear dimensionality reduction, has been widely used in feature extraction of HSI data to find meaningful representations of original spectrum. Nevertheless, lack of explicit and nonlinear mapping is still a limitation. In the paper, we propose a novel manifold learning based method for the classification of ground targets in HSI data, named as Polynomial Laplacian Embedding (PLAE). We first encode spatial information into spectral signal to obtain a fused representation. Then we model the local geometry of high-dimensional HSI data with graph Laplacian, and we introduce a nonlinear and explicit polynomial mapping to find a compact low-dimensional feature space, in which efficient classification of ground targets can be achieved. Experiments conducted on benchmark data sets demonstrate that high classification accuracy can be obtained by using the features extracted by PLAE.
更多查看译文
关键词
Supervised learning,Manifold learning,Feature extraction,Hyperspectral image classification,Graph laplacian
AI 理解论文
溯源树
样例
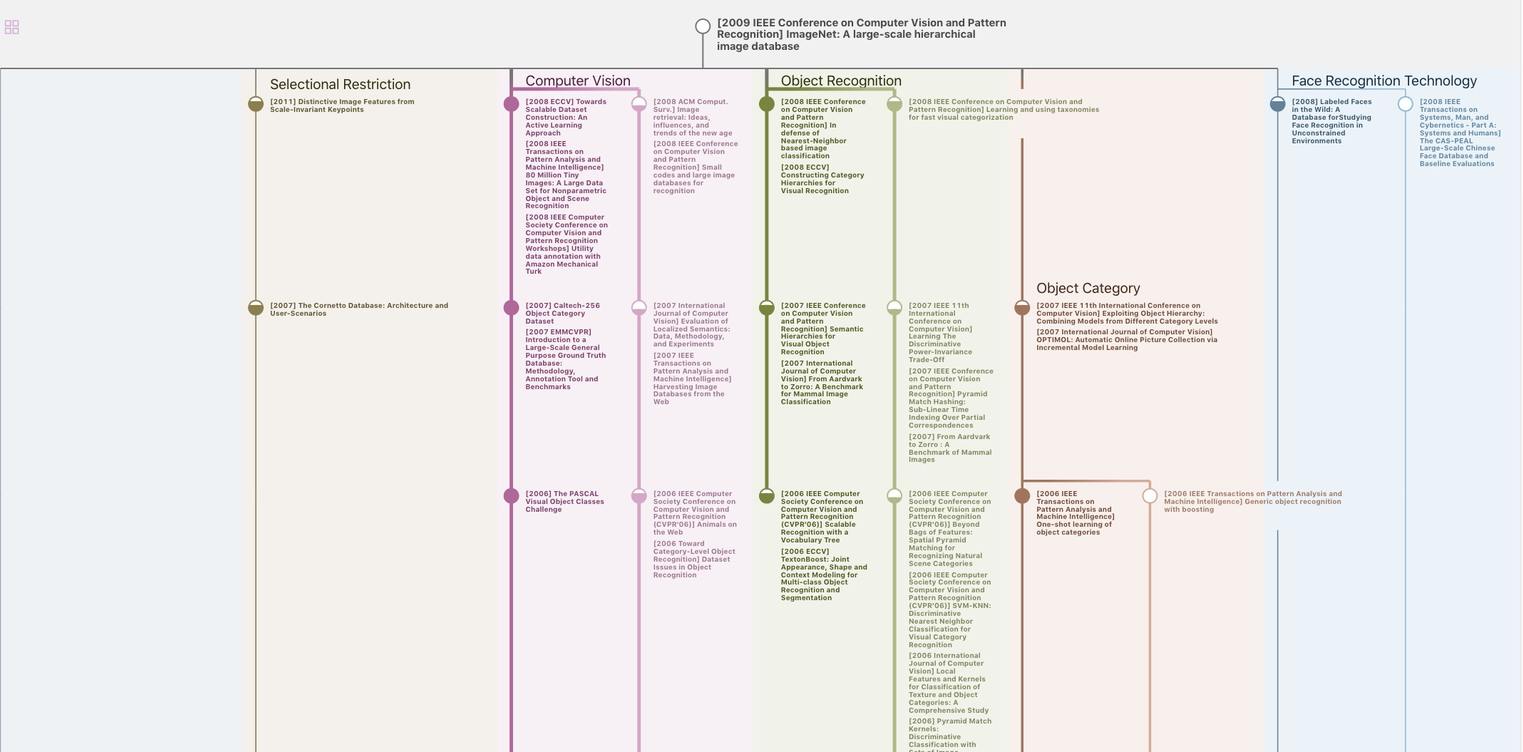
生成溯源树,研究论文发展脉络
Chat Paper
正在生成论文摘要