AutoMOMML: Automatic Multi-objective Modeling with Machine Learning
HIGH PERFORMANCE COMPUTING(2016)
摘要
In recent years, automatic data-driven modeling with machine learning (ML) has received considerable attention as an alternative to analytical modeling for many modeling tasks. While ad hoc adoption of ML approaches has obtained success, the real potential for automation in data-driven modeling has yet to be achieved. We propose AutoMOMML, an end-to-end, ML-based framework to build predictive models for objectives such as performance, and power. The framework adopts statistical approaches to reduce the modeling complexity and automatically identifies and configures the most suitable learning algorithm to model the required objectives based on hardware and application signatures. The experimental results using hardware counters as application signatures show that the median prediction error of performance, processor power, and DRAM power models are 13 %, 2.3 %, and 8 %, respectively.
更多查看译文
关键词
Training Point,Input Selection,Good Parameter Setting,Absolute Prediction Error,Hardware Counter
AI 理解论文
溯源树
样例
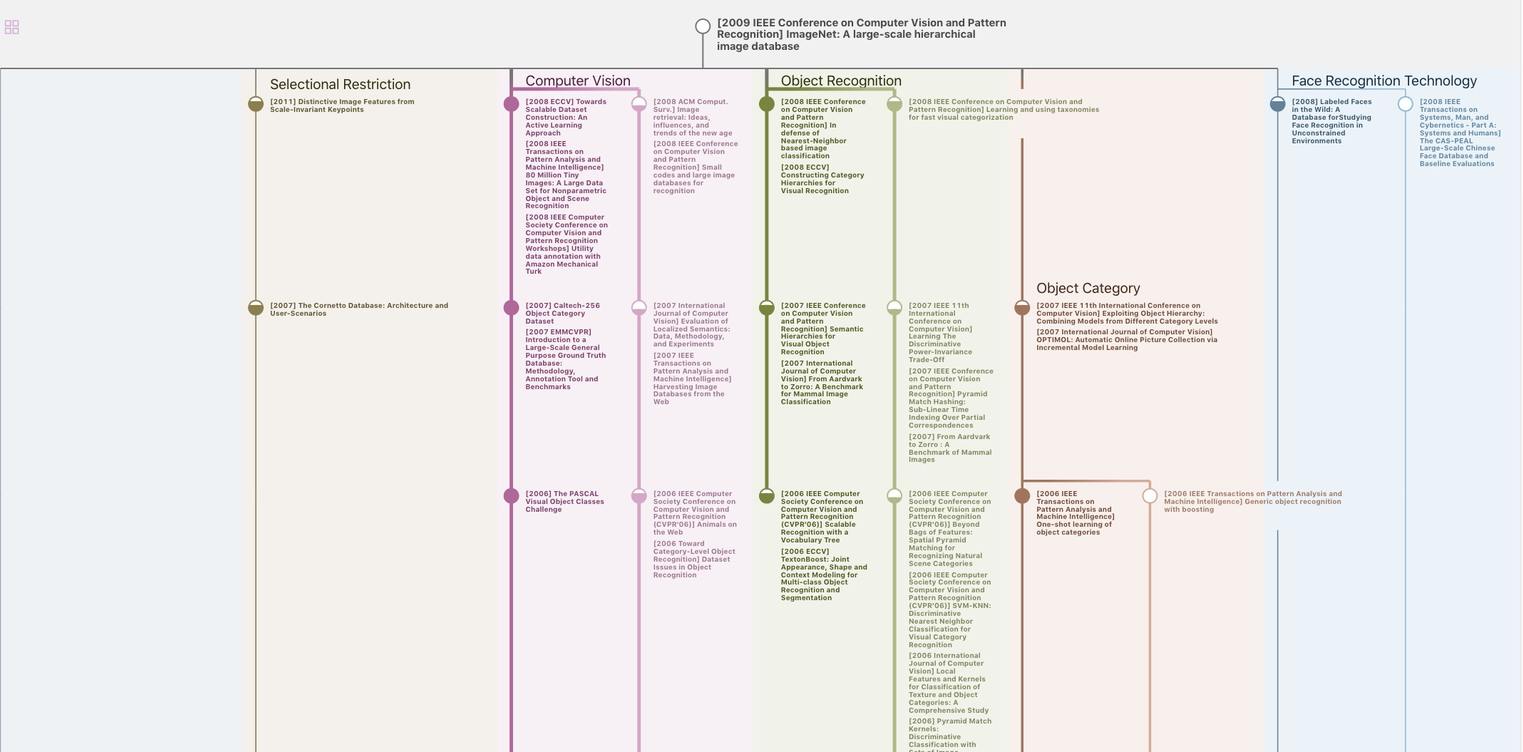
生成溯源树,研究论文发展脉络
Chat Paper
正在生成论文摘要