Inferring Population Genetic Parameters: Particle Filtering, HMM, Ripley's K-Function or Runs of Homozygosity?
ALGORITHMS IN BIOINFORMATICS(2016)
摘要
The coalescent with recombination is widely accepted as the key model to understand genetic diversity within a species. Many theoretical properties of the model are well understood, but formulating and implementing efficient inference methods remains a challenge. A major breakthrough has been to approximate the coalescent with recombination by a Markov chain along the sequences. Here we describe a new tool, RECJumper, for inference in the Markov approximated coalescent model. Previous methods are often based on a discretisation of the tree space and hidden Markov models. We avoid the discretisation by using particle filtering, and compare several proposal distributions. We also investigate runs of homozygosity, and introduce a new summary statistics from spatial statistics: Ripley's K-function. We find that (i) choosing an appropriate proposal distribution is crucial to obtain satisfactory behaviour in particle filtering, (ii) tree space discretisation in HMM-methodology is non-trivial and the choice can influence the results, and (iii) Ripley's K-function is a much more informative statistics than runs of homozygosity for recombination rate estimation.
更多查看译文
AI 理解论文
溯源树
样例
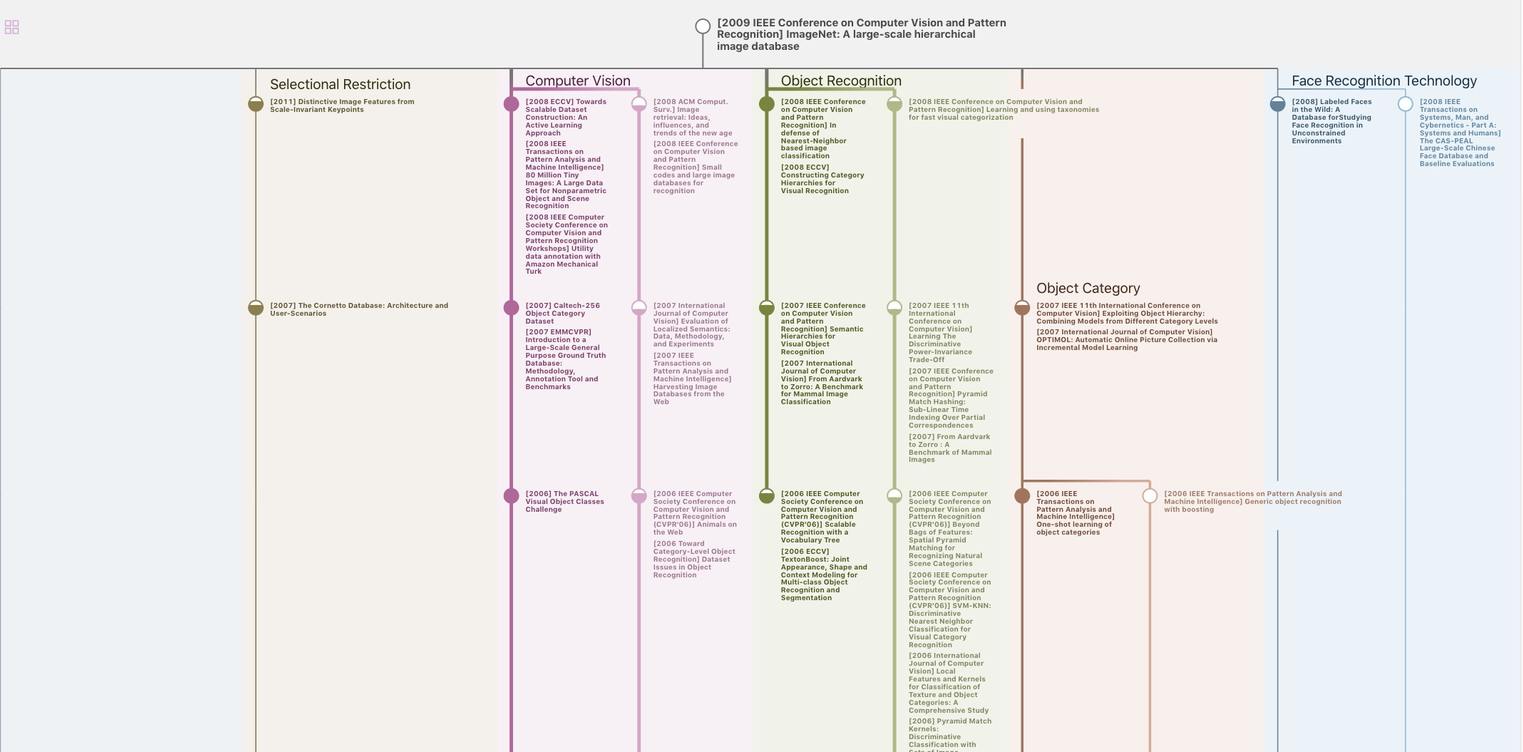
生成溯源树,研究论文发展脉络
Chat Paper
正在生成论文摘要