Global data association for the Probability Hypothesis Density filter using network flows
2016 IEEE International Conference on Robotics and Automation (ICRA)(2016)
摘要
The Probability Hypothesis Density (PHD) filter is an efficient formulation of multi-target state estimation that circumvents the combinatorial explosion of the multi-target posterior by operating on single-target space without maintaining target identities. In this paper, we propose a multi-target tracker based on the PHD filter that provides instantaneous state estimation and delayed decision on data association. For this purpose, we reformulate the PHD recursion in terms of single-target track hypotheses and solve a min-cost flow network for trajectory estimation where measurement likelihoods and transition probabilities are based on multi-target state estimates. In this manner, the presented approach combines global data association with efficient multi-target filtering. We evaluate the approach on a publicly available pedestrian tracking dataset to present state estimation and data association capabilities.
更多查看译文
关键词
pedestrian tracking dataset,transition probabilities,measurement likelihoods,PHD recursion,multitarget tracker,multitarget state estimation,network flows,probability hypothesis density filter,global data association
AI 理解论文
溯源树
样例
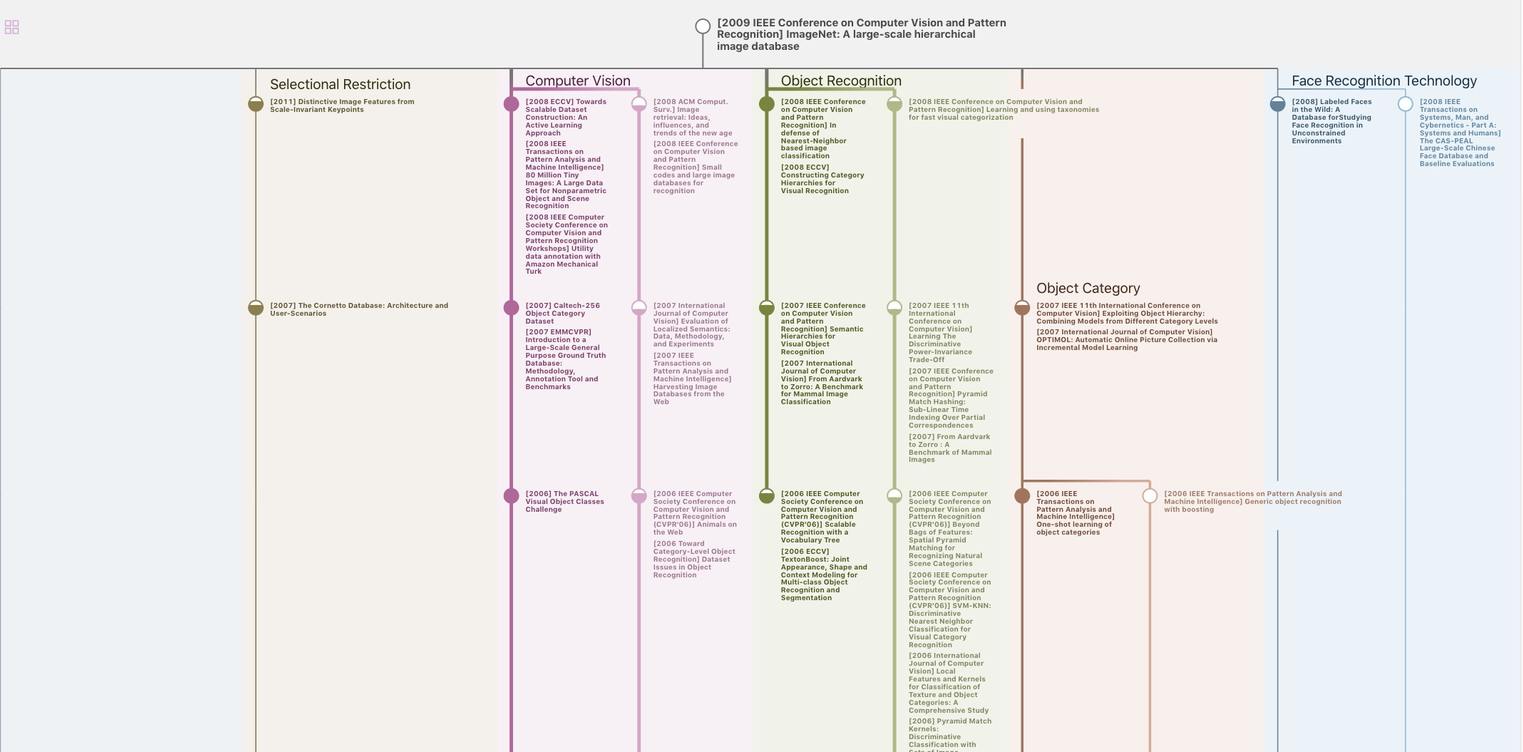
生成溯源树,研究论文发展脉络
Chat Paper
正在生成论文摘要