Instance Selection For Efficient And Reliable Camera Calibration
2016 IEEE International Conference on Robotics and Automation (ICRA)(2016)
摘要
The popularity of cameras for perception is enabled in part by powerful intrinsic calibration routines, commonly requiring a user to manually collect images of a known calibration target. The manual nature of this process produces training data that is unevenly spread in the camera relative pose space. If we desire camera parameters that perform well on average over the entire relative pose space, training on such a dataset results in poor performance. To address this, we show that reasoning about the training data distribution to select a more uniformly-spread subset of images produces more accurate and stable calibrations with fewer images. Our approach can be used easily with most camera calibration algorithms. We demonstrate in large-scale physical experiments the effect of non-uniform training data and show that our approach outperforms baselines in reprojection error and parameter variance.
更多查看译文
关键词
instance selection,camera calibration,training data distribution,large-scale physical experiments,nonuniform training data,reprojection error,parameter variance
AI 理解论文
溯源树
样例
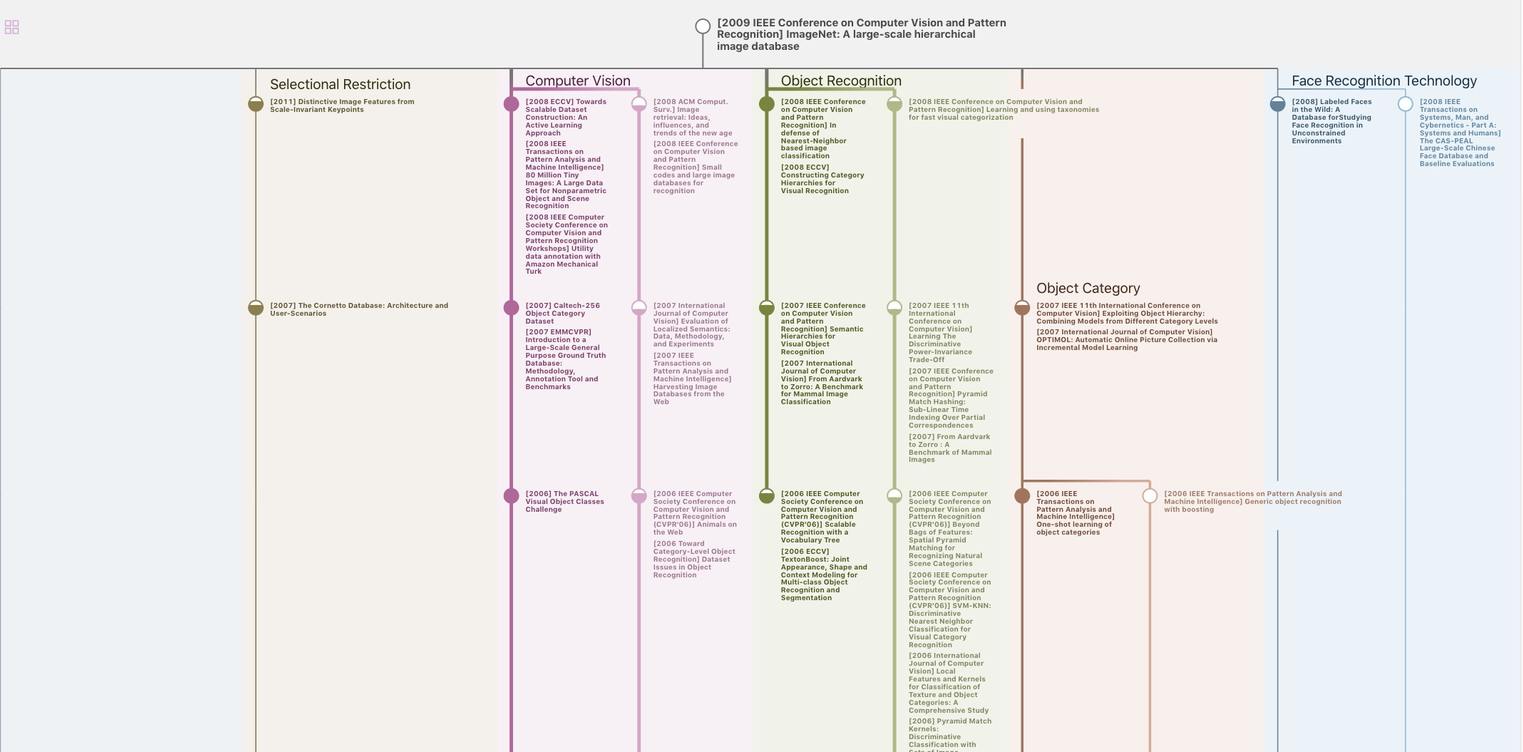
生成溯源树,研究论文发展脉络
Chat Paper
正在生成论文摘要