EigenTransitions with Hypothesis Testing: The Anatomy of Urban Mobility.
ICWSM(2016)
摘要
Identifying the patterns in urban mobility is important for a variety of tasks such as transportation planning, urban re- source allocation, emergency planning etc. This is evident from the large body of research on the topic, which has ex- ploded with the vast amount of geo-tagged user-generated content from online social media. However, most of the ex- isting work focuses on a specific setting, taking a statistical approach to describe and model the observed patterns. On the contrary in this work we introduce EigenTransitions, a spectrum-based, generic framework for analyzing spatio- temporal mobility datasets. EigenTransitions capture the anatomy of the aggregate and/or individuals’ mobility as a compact set of latent mobility patterns. Using a large cor- pus of geo-tagged content collected from Twitter, we utilize EigenTransitions to analyze the structure of urban mo- bility. In particular, we identify the EigenTransitions of a flow network between urban areas and derive hypothesis testing framework to evaluate urban mobility from both tem- poral and demographic perspectives. We further show how EigenTransitions not only identify latent mobility pat- terns, but also have the potential to support applications such as mobility prediction and inter-city comparisons. In partic- ular, by identifying neighbors with similar latent mobility patterns and incorporating their historical transition behav- iors, we proposed an EigenTransitions-based k-nearest neighbor algorithm, which can significantly improve the per- formance of individual mobility prediction. The proposed method is especially effective in “cold-start” scenarios where traditional methods are known to perform poorly.
更多查看译文
AI 理解论文
溯源树
样例
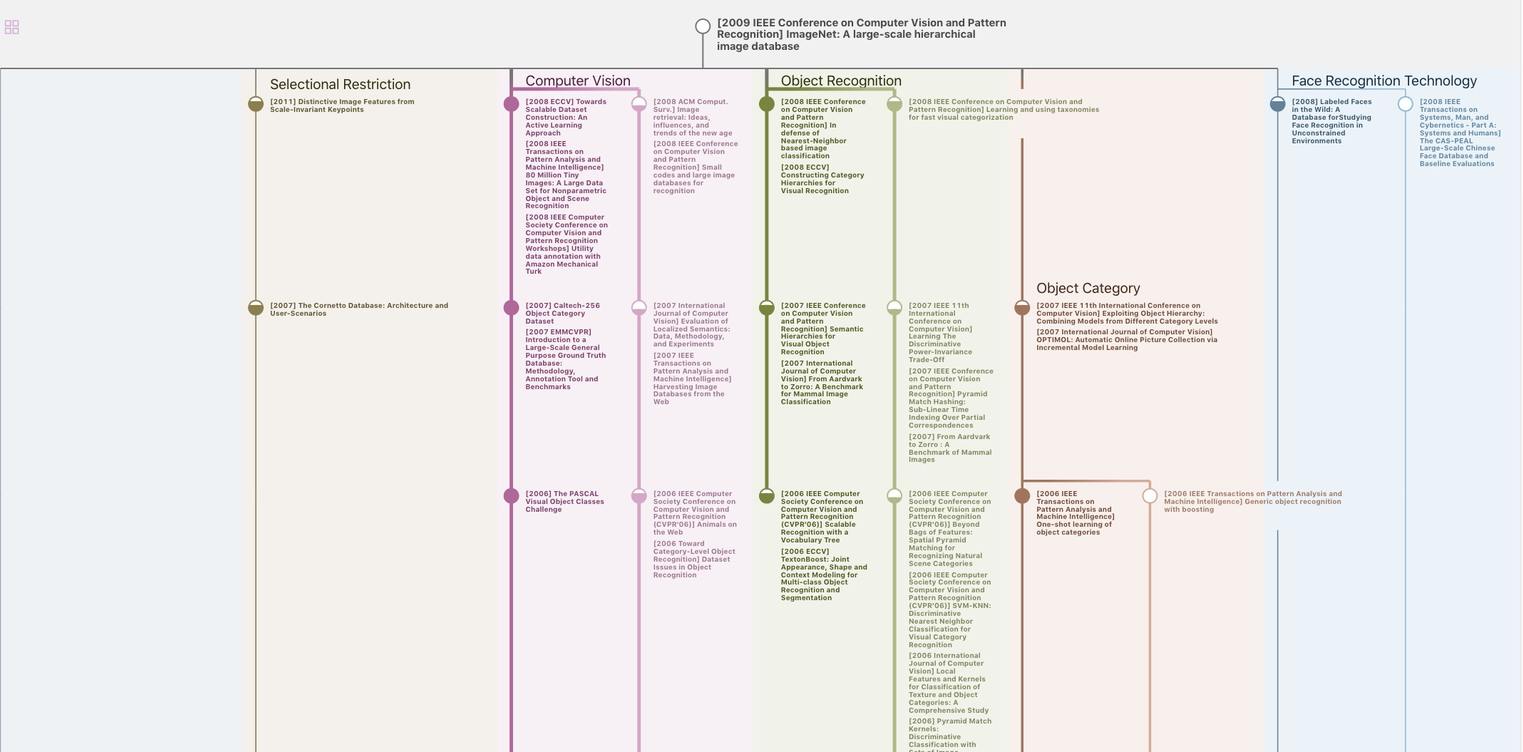
生成溯源树,研究论文发展脉络
Chat Paper
正在生成论文摘要