Robust Continuous-Discrete Extended Kalman Filter For Estimating Machine States With Model Uncertainties
2016 POWER SYSTEMS COMPUTATION CONFERENCE (PSCC)(2016)
摘要
Dynamic state estimation for synchronous generators is rapidly gaining importance due to its impact on wide-area control and stability of large scale power grids. However, the underlying uncertainties in the dynamic models of the generators may influence the estimation results. In this paper, a robust extended Kalman filter is developed for estimating machine states in the presence of model uncertainties. The proposed filter is based on minimizing the squared residual norm under the worst possible case, which indicates the uncertainties in the model should be bounded. The proposed algorithm is derived in two steps. First, the nonlinear dynamic equations of the machine model as well as the structured uncertainties are discretized and linearized. Second, by constructing the min-max optimization problem and solving it with considerable algebra, the time-and measurement-update expressions of the Kalman filter can be reformulated with modified parameters. The proposed filter is tested numerically based on a typical machine model and the results are presented.
更多查看译文
关键词
Dynamic state estimation,machine states,nonlinear state-space estimation,parametric uncertainty,robust extended Kalman filter
AI 理解论文
溯源树
样例
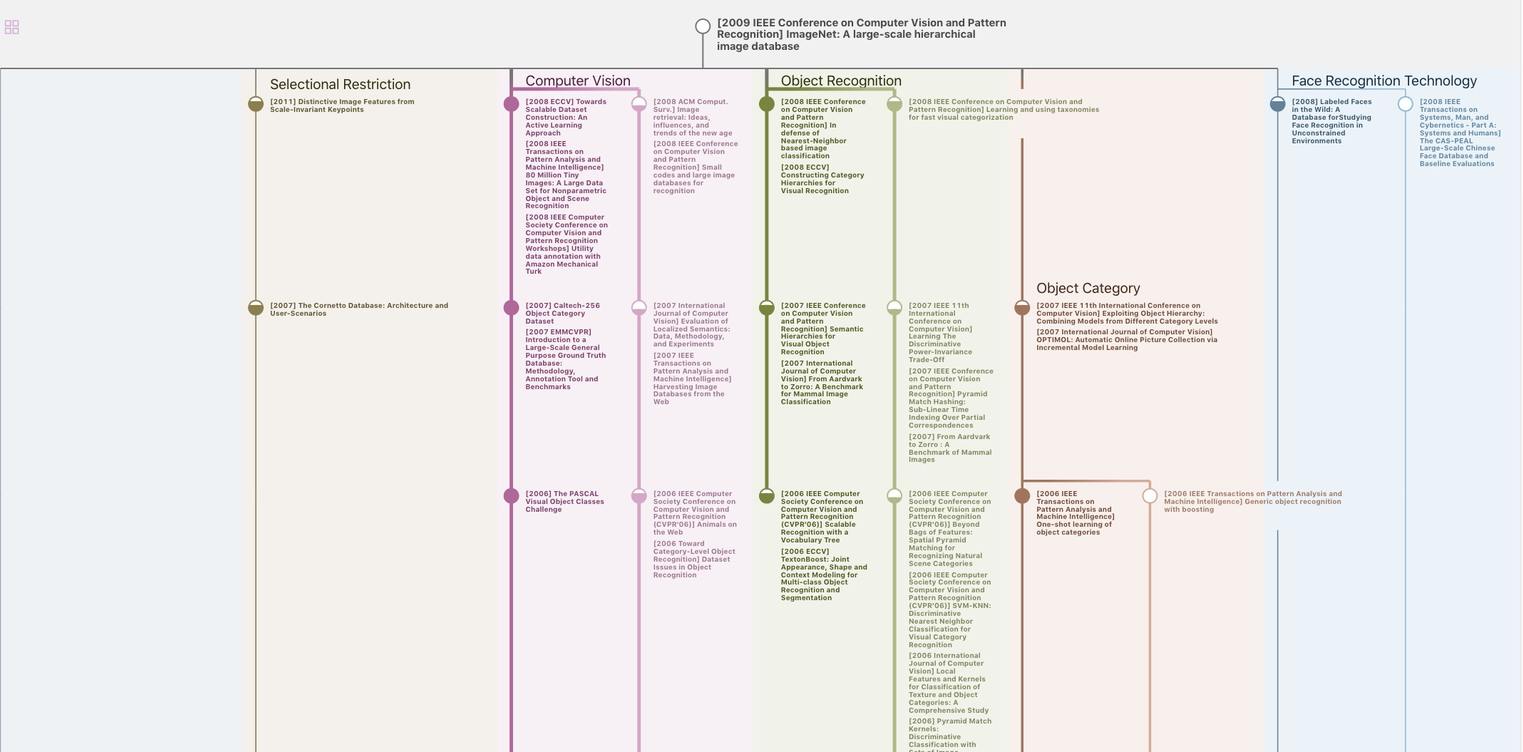
生成溯源树,研究论文发展脉络
Chat Paper
正在生成论文摘要