Optimal Testing For Crowd Workers
AAMAS '16: Proceedings of the 2016 International Conference on Autonomous Agents & Multiagent Systems(2016)
摘要
Requesters on crowdsourcing platforms, such as Amazon Mechanical Turk, routinely insert gold questions to verify that a worker is diligent and is providing high- quality answers. However, there is no clear understanding of when and how many gold questions to insert. Typically, requesters mix a flat 10{30% of gold questions into the task stream of every worker. This static policy is arbitrary and wastes valuable budget | the exact percentage is often chosen with little experimentation, and, more importantly, it does not adapt to individual workers, the current mixture of spamming vs. diligent workers, or the number of tasks workers perform before quitting.We formulate the problem of balancing between (1) testing workers to determine their accuracy and (2) actually getting work performed as a partially- observable Markov decision process (POMDP) and apply reinforcement learning to dynamically calculate the best policy. Evaluations on both synthetic data and with real Mechanical Turk workers show that our agent learns adaptive testing policies that produce up to 111% more reward than the non- adaptive policies used by most requesters. Furthermore, our method is fully automated, easy to apply, and runs mostly out of the box.
更多查看译文
关键词
Crowdsourcing,reinforcement learning
AI 理解论文
溯源树
样例
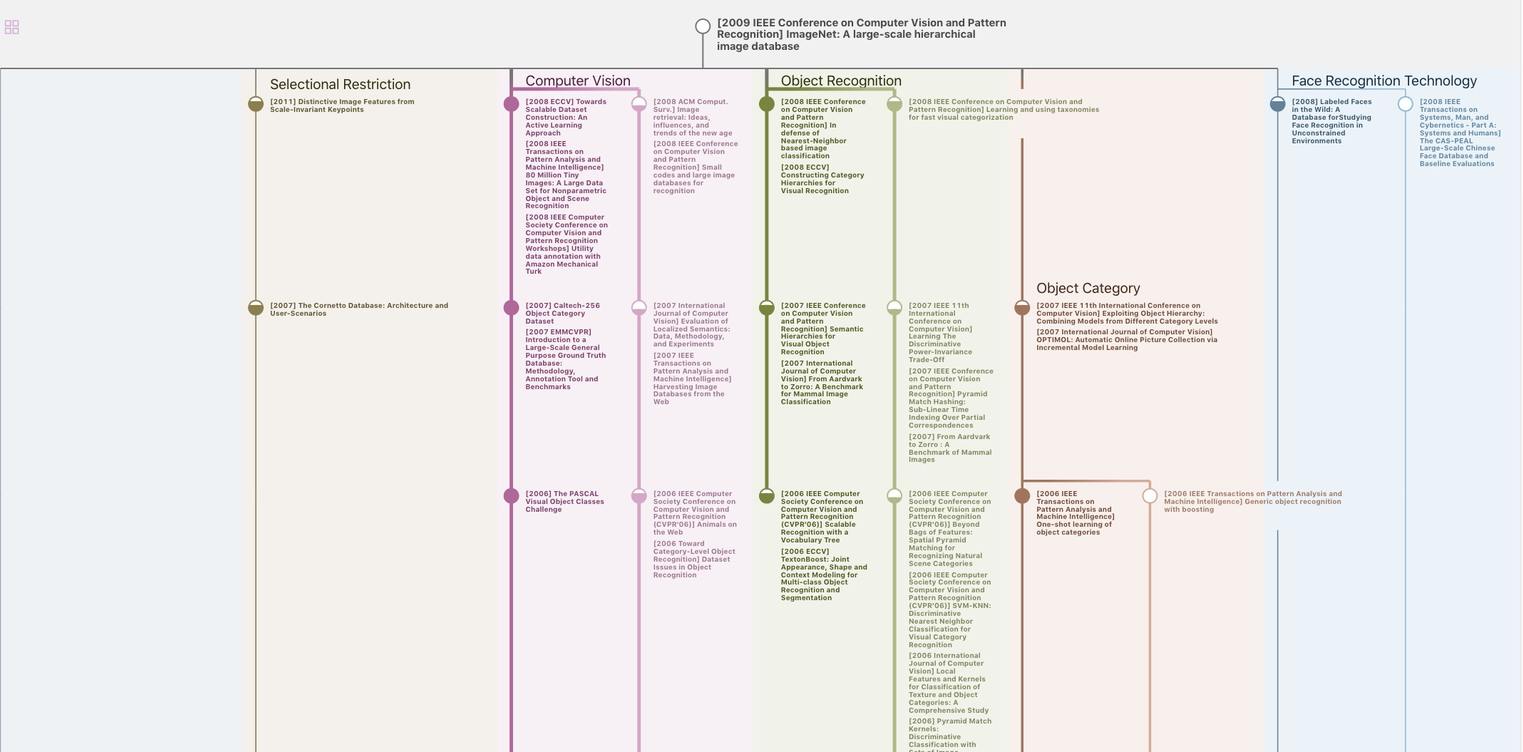
生成溯源树,研究论文发展脉络
Chat Paper
正在生成论文摘要