Active Learning For Dependency Parsing With Partial Annotation
PROCEEDINGS OF THE 54TH ANNUAL MEETING OF THE ASSOCIATION FOR COMPUTATIONAL LINGUISTICS, VOL 1(2016)
摘要
Different from traditional active learning based on sentence-wise full annotation (FA), this paper proposes active learning with dependency-wise partial annotation (PA) as a finer-grained unit for dependency parsing. At each iteration, we select a few most uncertain words from an unlabeled data pool, manually annotate their syntactic heads, and add the partial trees into labeled data for parser retraining. Compared with sentence-wise FA, dependency-wise PA gives us more flexibility in task selection and avoids wasting time on annotating trivial tasks in a sentence. Our work makes the following contributions. First, we are the first to apply a probabilistic model to active learning for dependency parsing, which can 1) provide tree probabilities and dependency marginal probabilities as principled uncertainty metrics, and 2) directly learn parameters from PA based on a forest-based training objective. Second, we propose and compare several uncertainty metrics through simulation experiments on both Chinese and English. Finally, we conduct human annotation experiments to compare FA and PA on real annotation time and quality.
更多查看译文
AI 理解论文
溯源树
样例
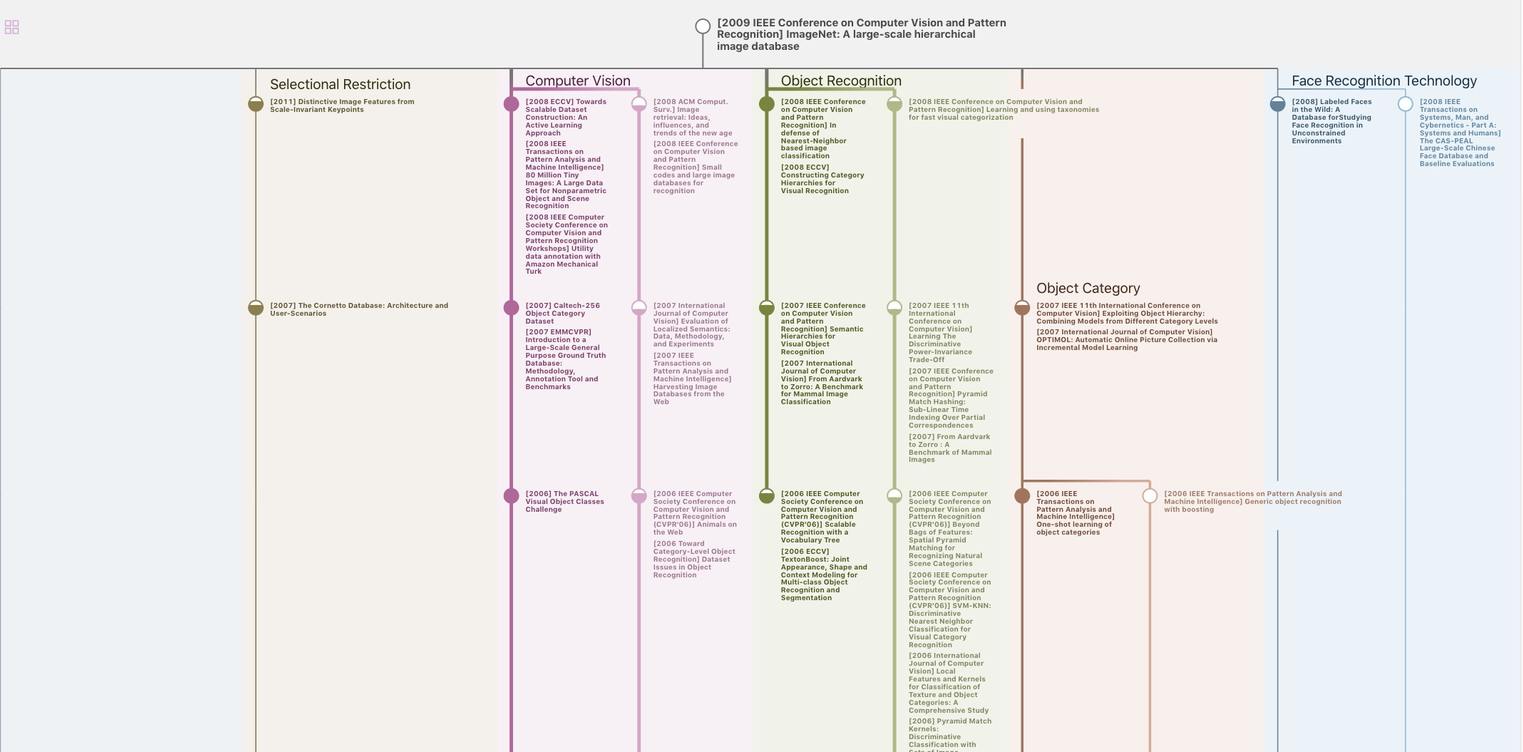
生成溯源树,研究论文发展脉络
Chat Paper
正在生成论文摘要