Learning To Respond With Deep Neural Networks For Retrieval-Based Human-Computer Conversation System
IR(2016)
摘要
To establish an automatic conversation system between humans and computers is regarded as one of the most hardcore problems in computer science, which involves interdisciplinary techniques in information retrieval, natural language processing, artificial intelligence, etc. The challenges lie in how to respond so as to maintain a relevant and continuous conversation with humans. Along with the prosperity of Web 2.0, we are now able to collect extremely massive conversational data, which are publicly available. It casts a great opportunity to launch automatic conversation systems. Owing to the diversity of Web resources, a retrieval-based conversation system will be able to find at least some responses from the massive repository for any user inputs. Given a human issued message, i.e., query, our system would provide a reply after adequate training and learning of how to respond. In this paper, we propose a retrieval-based conversation system with the deep learning-to-respond schema through a deep neural network framework driven by web data. The proposed model is general and unified for different conversation scenarios in open domain. We incorporate the impact of multiple data inputs, and formulate various features and factors with optimization into the deep learning framework. In the experiments, we investigate the effectiveness of the proposed deep neural network structures with better combinations of all different evidence. We demonstrate significant performance improvement against a series of standard and state-of-art baselines in terms of p@1, MAP, nDCG, and MRR for conversational purposes.
更多查看译文
关键词
Learning-to-respond,conversation system,contextual modeling,deep neural networks
AI 理解论文
溯源树
样例
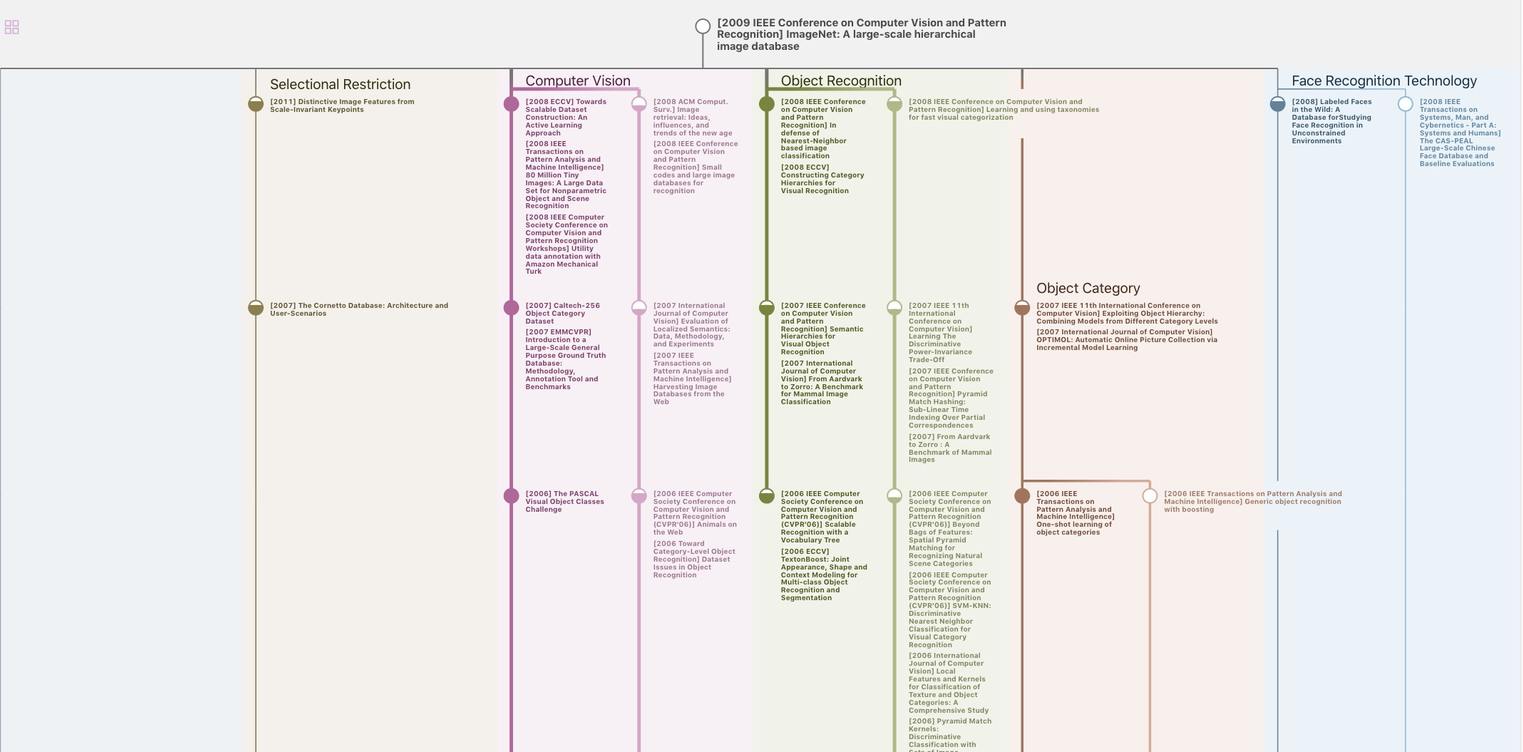
生成溯源树,研究论文发展脉络
Chat Paper
正在生成论文摘要