Cost-sensitive boosting algorithms: Do we really need them?
Machine Learning(2016)
摘要
We provide a unifying perspective for two decades of work on cost-sensitive Boosting algorithms. When analyzing the literature 1997–2016, we find 15 distinct cost-sensitive variants of the original algorithm; each of these has its own motivation and claims to superiority—so who should we believe? In this work we critique the Boosting literature using four theoretical frameworks: Bayesian decision theory, the functional gradient descent view, margin theory, and probabilistic modelling. Our finding is that only three algorithms are fully supported—and the probabilistic model view suggests that all require their outputs to be calibrated for best performance. Experiments on 18 datasets across 21 degrees of imbalance support the hypothesis—showing that once calibrated, they perform equivalently, and outperform all others. Our final recommendation—based on simplicity, flexibility and performance—is to use the original Adaboost algorithm with a shifted decision threshold and calibrated probability estimates.
更多查看译文
关键词
Boosting,Cost-sensitive,Class imbalance,Classifier calibration
AI 理解论文
溯源树
样例
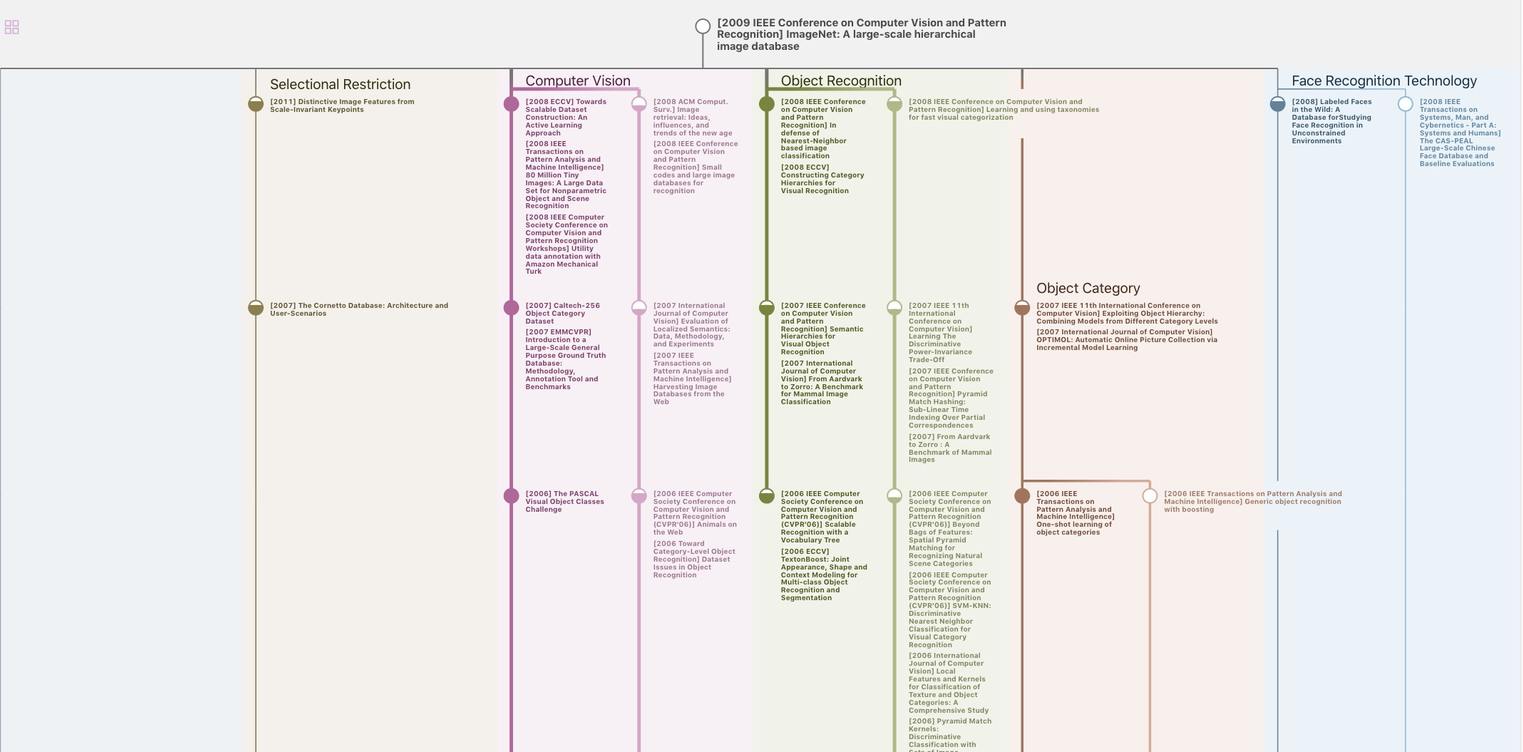
生成溯源树,研究论文发展脉络
Chat Paper
正在生成论文摘要