Partial Label Learning via Feature-Aware Disambiguation
KDD(2016)
摘要
Partial label learning deals with the problem where each training example is represented by a feature vector while associated with a set of candidate labels, among which only one label is valid. To learn from such ambiguous labeling information, the key is to try to disambiguate the candidate label sets of partial label training examples. Existing disambiguation strategies work by either identifying the ground-truth label iteratively or treating each candidate label equally. Nonetheless, the disambiguation process is generally conducted by focusing on manipulating the label space, and thus ignores making full use of potentially useful information from the feature space. In this paper, a novel two-stage approach is proposed to learning from partial label examples based on feature-aware disambiguation. In the first stage, the manifold structure of feature space is utilized to generate normalized labeling confidences over candidate label set. In the second stage, the predictive model is learned by performing regularized multi-output regression over the generated labeling confidences. Extensive experiments on artificial as well as real-world partial label data sets clearly validate the superiority of the proposed feature-aware disambiguation approach.
更多查看译文
关键词
weak supervision,partial label learning,disambiguation,manifold
AI 理解论文
溯源树
样例
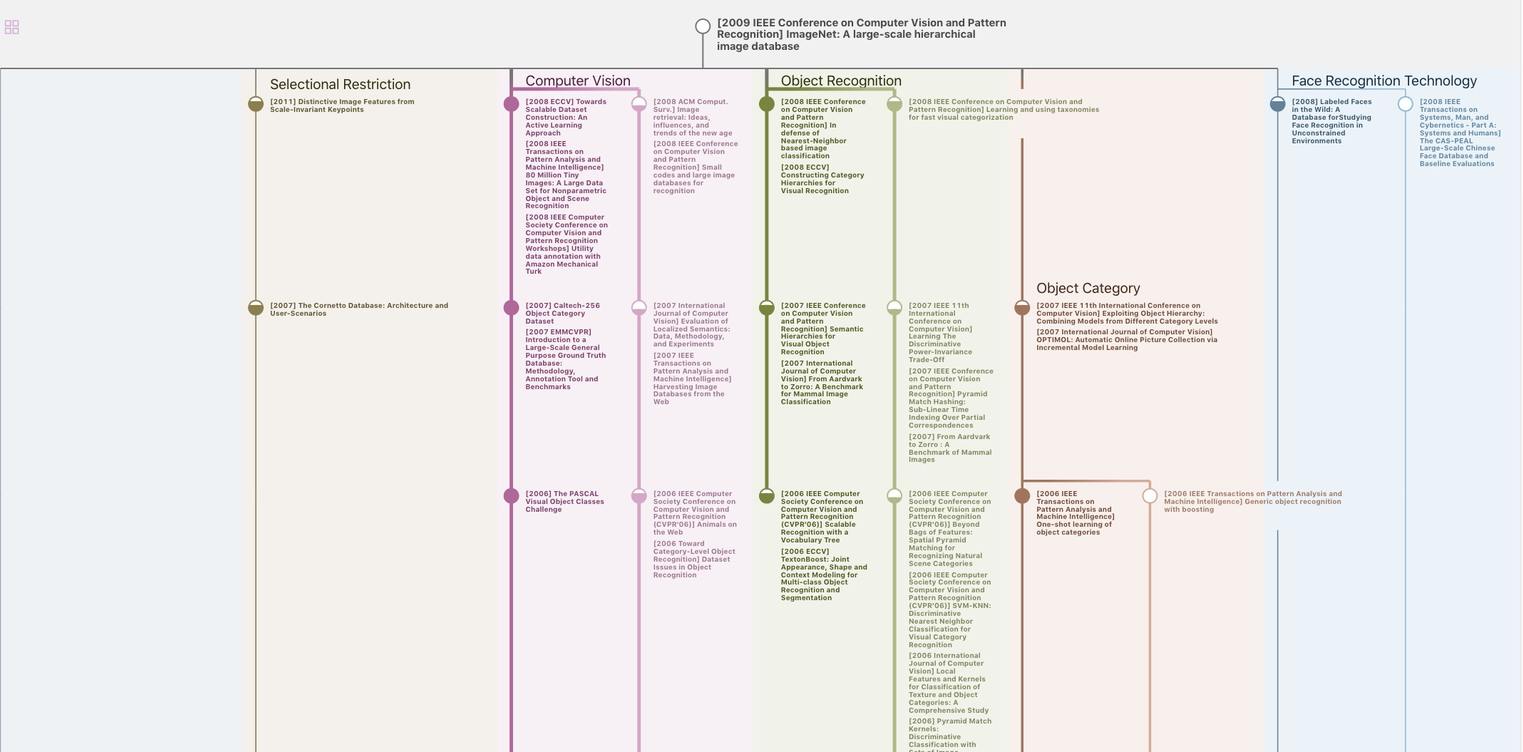
生成溯源树,研究论文发展脉络
Chat Paper
正在生成论文摘要