Human activity recognition with smartphone sensors using deep learning neural networks.
Expert Syst. Appl.(2016)
摘要
This paper proposes a deep convolutional neural network for HAR using smartphone sensors.Experiments show that the proposed method derives relevant and more complex features.The method achieved an almost perfect classification on moving activities.It outperforms other state-of-the-art data mining techniques in HAR. Human activities are inherently translation invariant and hierarchical. Human activity recognition (HAR), a field that has garnered a lot of attention in recent years due to its high demand in various application domains, makes use of time-series sensor data to infer activities. In this paper, a deep convolutional neural network (convnet) is proposed to perform efficient and effective HAR using smartphone sensors by exploiting the inherent characteristics of activities and 1D time-series signals, at the same time providing a way to automatically and data-adaptively extract robust features from raw data. Experiments show that convnets indeed derive relevant and more complex features with every additional layer, although difference of feature complexity level decreases with every additional layer. A wider time span of temporal local correlation can be exploited (1×9-1×14) and a low pooling size (1×2-1×3) is shown to be beneficial. Convnets also achieved an almost perfect classification on moving activities, especially very similar ones which were previously perceived to be very difficult to classify. Lastly, convnets outperform other state-of-the-art data mining techniques in HAR for the benchmark dataset collected from 30 volunteer subjects, achieving an overall performance of 94.79% on the test set with raw sensor data, and 95.75% with additional information of temporal fast Fourier transform of the HAR data set.
更多查看译文
关键词
Human activity recognition,Deep learning,Convolutional neural network,Smartphone,Sensors
AI 理解论文
溯源树
样例
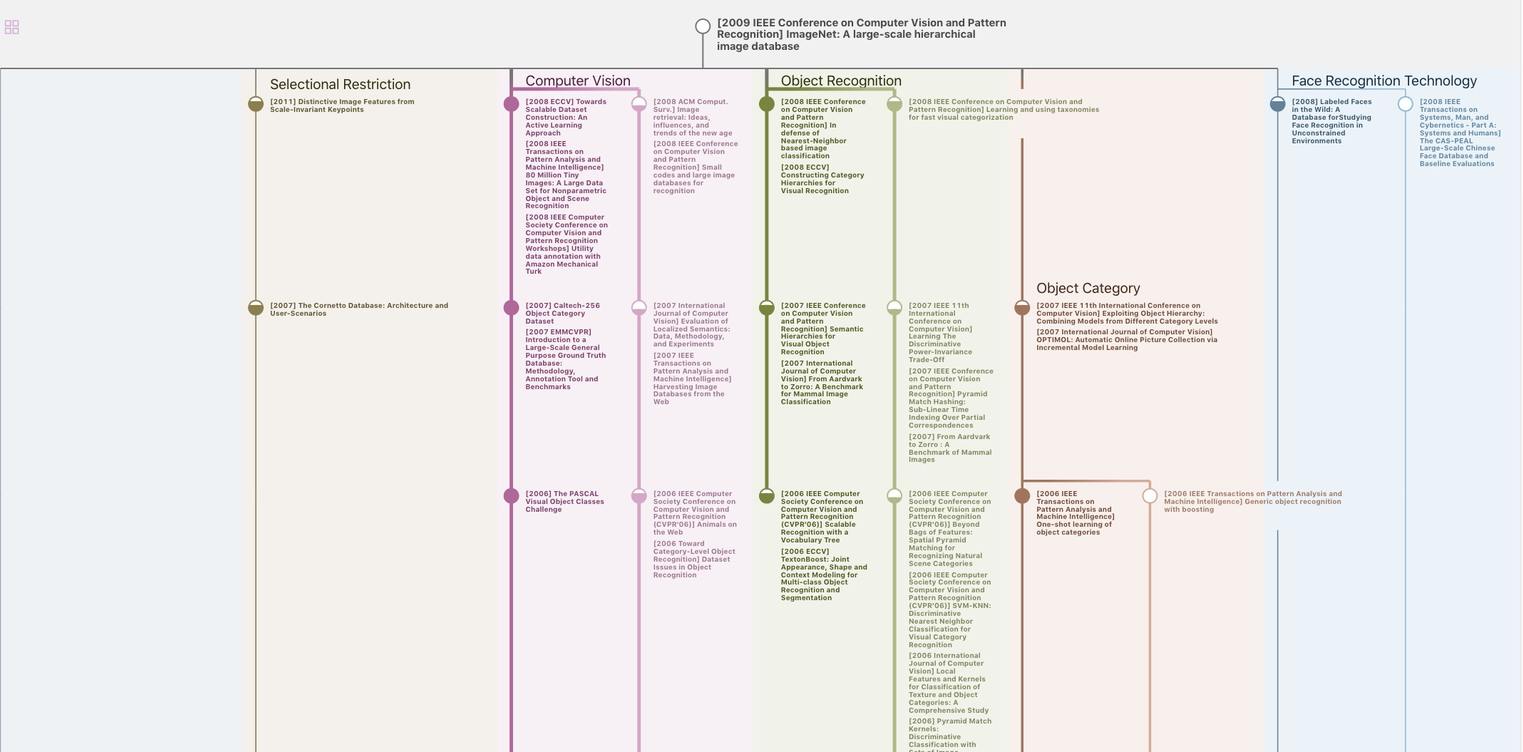
生成溯源树,研究论文发展脉络
Chat Paper
正在生成论文摘要