Joint semantic similarity assessment with raw corpus and structured ontology for semantic-oriented service discovery
Personal and Ubiquitous Computing(2016)
摘要
Semantic-oriented service matching is one of the challenges in automatic Web service discovery. Service users may search for Web services using keywords and receive the matching services in terms of their functional profiles. A number of approaches to computing the semantic similarity between words have been developed to enhance the precision of matchmaking, which can be classified into ontology-based and corpus-based approaches. The ontology-based approaches commonly use the differentiated concept information provided by a large ontology for measuring lexical similarity with word sense disambiguation. Nevertheless, most of the ontologies are domain-special and limited to lexical coverage, which have a limited applicability. On the other hand, corpus-based approaches rely on the distributional statistics of context to represent per word as a vector and measure the distance of word vectors. However, the polysemous problem may lead to a low computational accuracy. In this paper, in order to augment the semantic information content in word vectors, we propose a multiple semantic fusion (MSF) model to generate sense-specific vector per word. In this model, various semantic properties of the general-purpose ontology WordNet are integrated to fine-tune the distributed word representations learned from corpus, in terms of vector combination strategies. The retrofitted word vectors are modeled as semantic vectors for estimating semantic similarity. The MSF model-based similarity measure is validated against other similarity measures on multiple benchmark datasets. Experimental results of word similarity evaluation indicate that our computational method can obtain higher correlation coefficient with human judgment in most cases. Moreover, the proposed similarity measure is demonstrated to improve the performance of Web service matchmaking based on a single semantic resource. Accordingly, our findings provide a new method and perspective to understand and represent lexical semantics.
更多查看译文
关键词
Joint semantic similarity assessment,Feature fusion,Low-dimensional vector space,WordNet,Service discovery
AI 理解论文
溯源树
样例
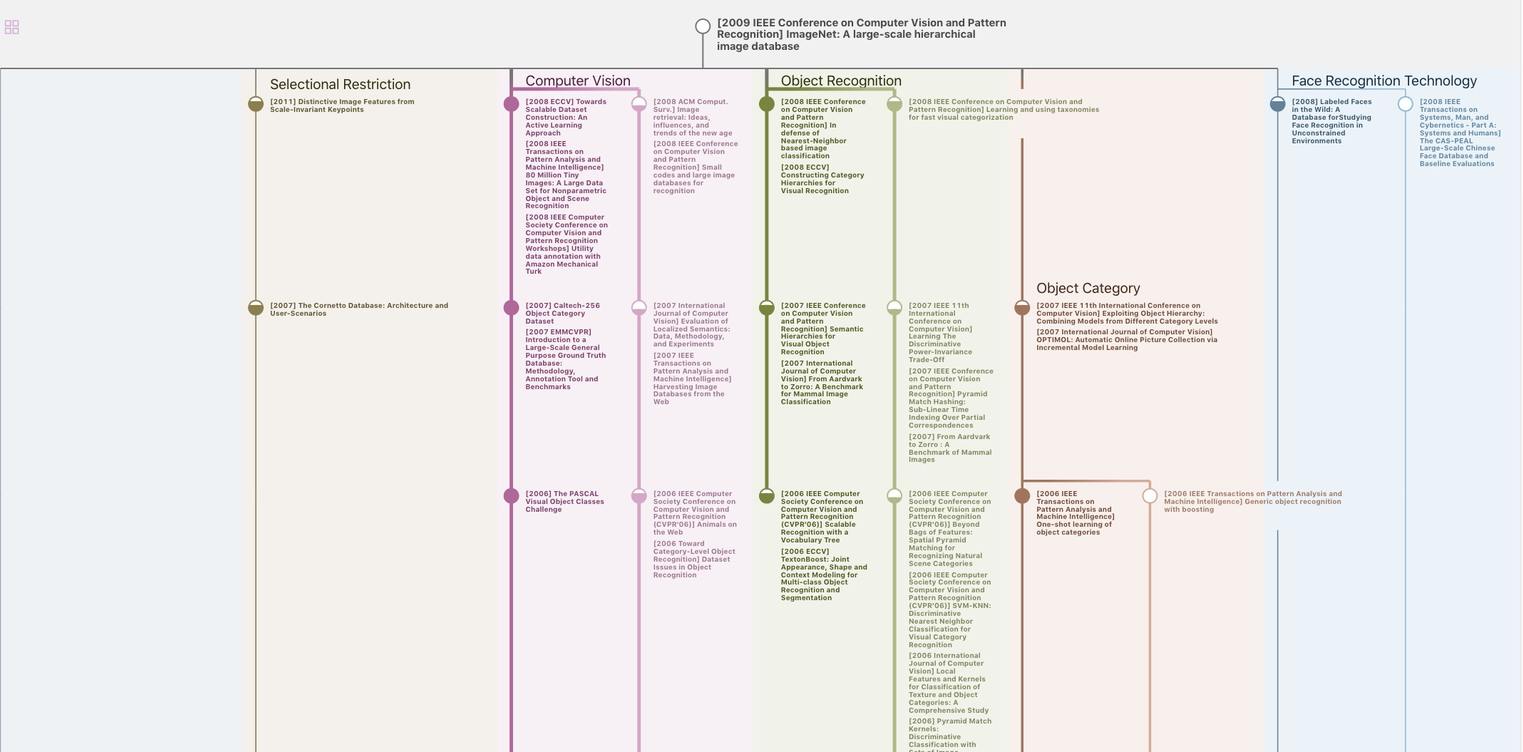
生成溯源树,研究论文发展脉络
Chat Paper
正在生成论文摘要