Characterizing and Optimizing Cellular Network Performance During Crowded Events.
IEEE/ACM Trans. Netw.(2016)
摘要
During crowded events, cellular networks face voice and data traffic volumes that are often orders of magnitude higher than what they face during routine days. Despite the use of portable base stations for temporarily increasing communication capacity and free Wi-Fi access points for offloading Internet traffic from cellular base stations, crowded events still present significant challenges for cellular network operators looking to reduce dropped call events and improve Internet speeds. For an effective cellular network design, management, and optimization, it is crucial to understand how cellular network performance degrades during crowded events, what causes this degradation, and how practical mitigation schemes would perform in real-life crowded events. This paper makes a first step toward this end by characterizing the operational performance of a tier-1 cellular network in the U.S. during two high-profile crowded events in 2012. We illustrate how the changes in population distribution, user behavior, and application workload during crowded events result in significant voice and data performance degradation, including more than two orders of magnitude increase in connection failures. Our findings suggest two mechanisms that can improve performance without resorting to costly infrastructure changes: radio resource allocation tuning and opportunistic connection sharing. Using trace-driven simulations, we show that more aggressive release of radio resources via 1-2 s shorter radio resource control timeouts as compared with routine days helps to achieve better tradeoff between wasted radio resources, energy consumption, and delay during crowded events, and opportunistic connection sharing can reduce connection failures by 95% when employed by a small number of devices in each cell sector.
更多查看译文
关键词
Energy consumption,Internet,IEEE 802.11 Standard,Performance evaluation,Resource management,Base stations,Tuning
AI 理解论文
溯源树
样例
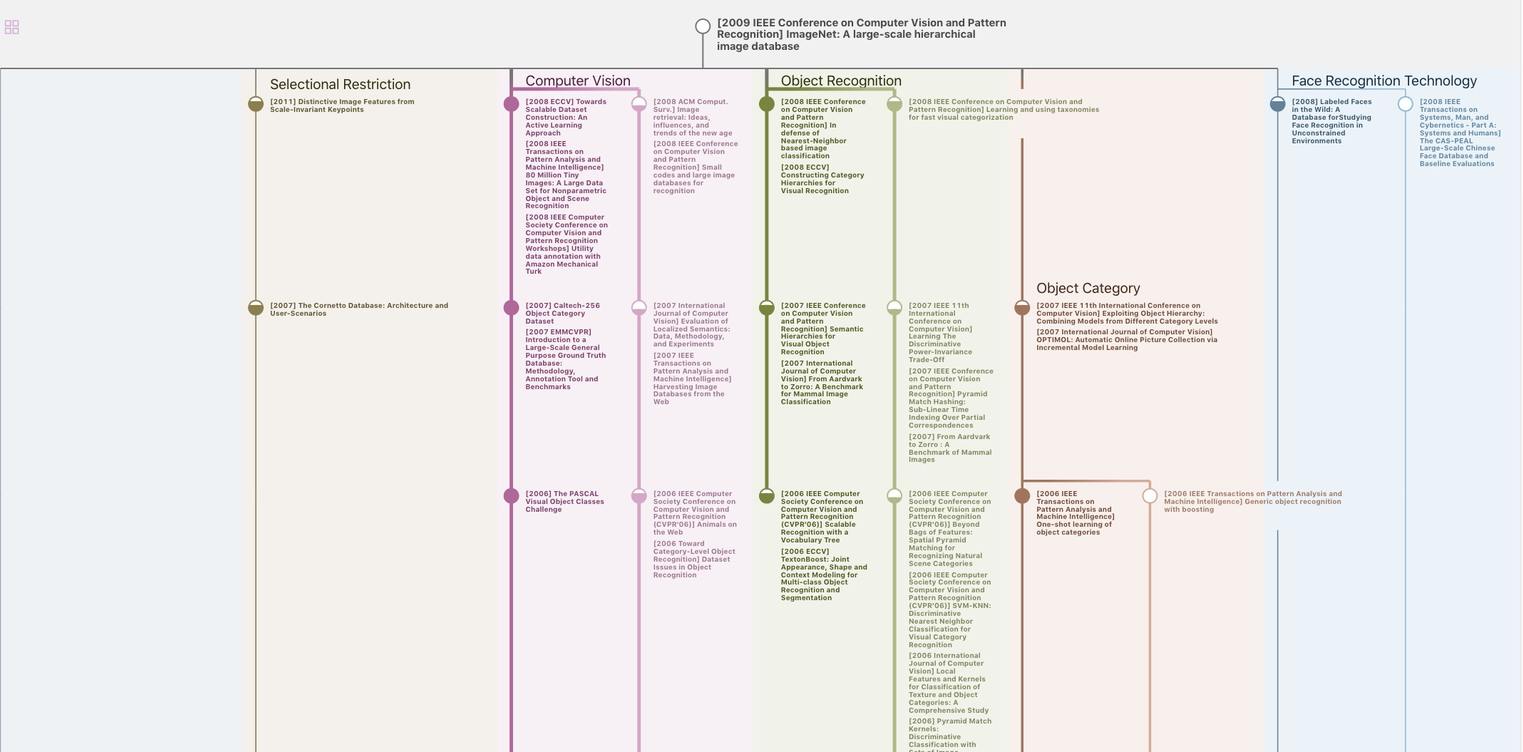
生成溯源树,研究论文发展脉络
Chat Paper
正在生成论文摘要