Bayesian Hyperparameter Optimization for Ensemble Learning
UAI'16: Proceedings of the Thirty-Second Conference on Uncertainty in Artificial Intelligence(2016)
摘要
In this paper, we bridge the gap between hyperparameter optimization and ensemble learning by performing Bayesian optimization of an ensemble with regards to its hyperparameters. Our method consists in building a fixed-size ensemble, optimizing the configuration of one classifier of the ensemble at each iteration of the hyperparameter optimization algorithm, taking into consideration the interaction with the other models when evaluating potential performances. We also consider the case where the ensemble is to be reconstructed at the end of the hyperparameter optimization phase, through a greedy selection over the pool of models generated during the optimization. We study the performance of our proposed method on three different hyperparameter spaces, showing that our approach is better than both the best single model and a greedy ensemble construction over the models produced by a standard Bayesian optimization.
更多查看译文
关键词
bayesian hyperparameter optimization,learning
AI 理解论文
溯源树
样例
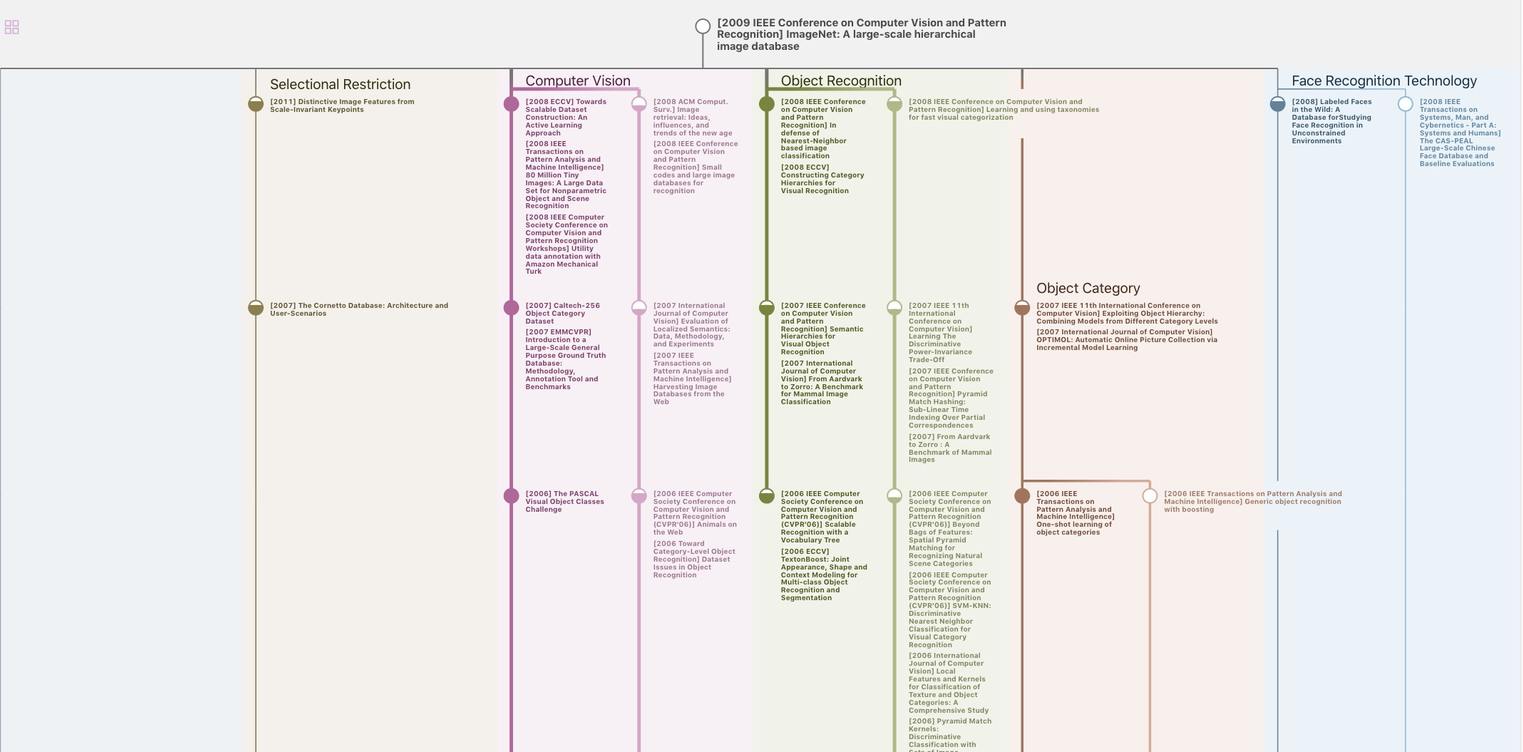
生成溯源树,研究论文发展脉络
Chat Paper
正在生成论文摘要