Higher-Order Block Term Decomposition for Spatially Folded fMRI Data
LVA/ICA(2017)
摘要
The growing use of neuroimaging technologies generates a massive amount of biomedical data that exhibit high dimensionality. Tensor-based analysis of brain imaging data has been proved quite effective in exploiting their multiway nature. The advantages of tensorial methods over matrix-based approaches have also been demonstrated in the context of functional magnetic resonance imaging (fMRI) data analysis. However, such methods can become ineffective in demanding scenarios, involving, e.g., strong noise and/or significant overlapping of activated regions. This paper aims at investigating the possible gains that can be obtained from a better exploitation of the spatial dimension, through a higher (than 3)-order tensor modeling of the fMRI signals. In this context, a higher-order Block Term Decomposition (BTD) is applied, for the first time in fMRI analysis. Its effectiveness in handling strong instances of noise is demonstrated via extensive simulation results.
更多查看译文
关键词
fMRI,Tensors,BTD,CPD,TPICA
AI 理解论文
溯源树
样例
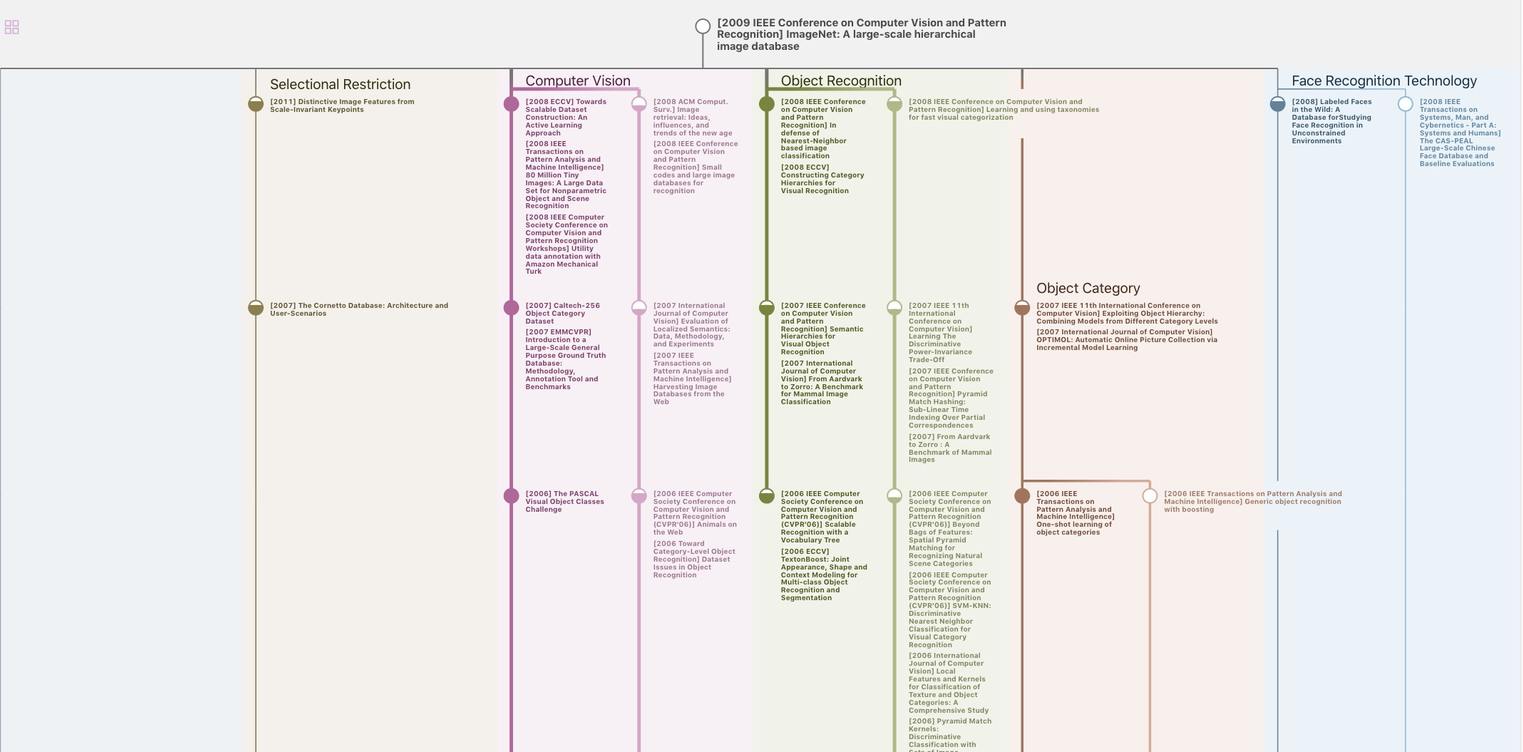
生成溯源树,研究论文发展脉络
Chat Paper
正在生成论文摘要