A General Distributed Dual Coordinate Optimization Framework for Regularized Loss Minimization.
Journal of Machine Learning Research(2017)
摘要
In modern large-scale machine learning applications, the training are often partitioned and stored on multiple machines. It is customary to employ the data approach, where the aggregated training loss is minimized without moving across machines. In this paper, we introduce a novel distributed dual formulation for regularized loss minimization problems that can directly handle parallelism under the distributed computing environment. This formulation allows us to systematically derive dual coordinate optimization procedures, which we refer to as Distributed Alternating Dual Maximization (DADM). The method extends earlier studies about distributed SDCA algorithms and has a rigorous theoretical analysis. Based on the new formulation, we also develop an accelerated DADM algorithm by generalizing the acceleration technique from the accelerated SDCA algorithm to the distributed setting. Our empirical studies show that our novel approach significantly improves the previous state-of-the-art distributed dual coordinate optimization algorithms.
更多查看译文
关键词
distributed optimization,stochastic dual coordinate ascent,acceleration,regularized loss minimization,computational complexity
AI 理解论文
溯源树
样例
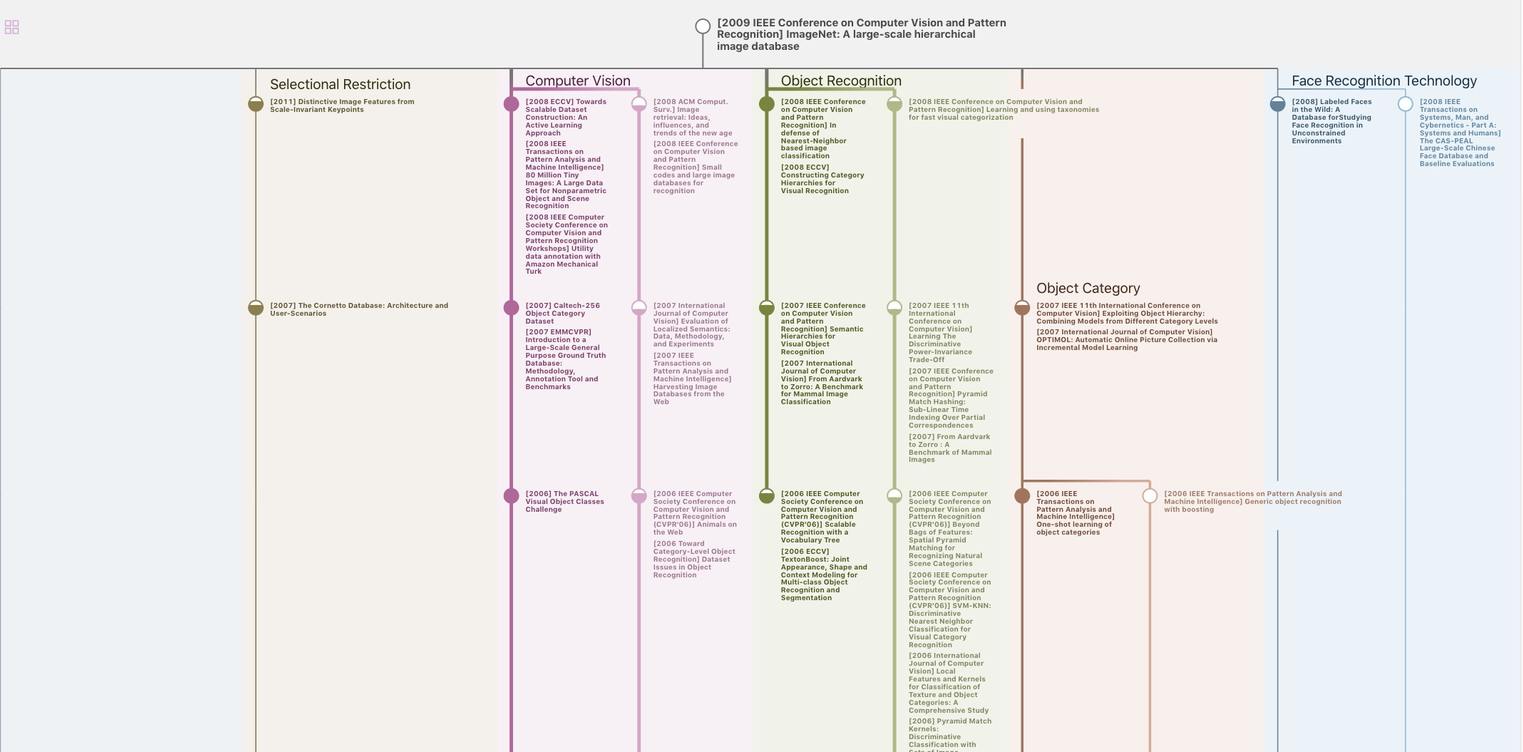
生成溯源树,研究论文发展脉络
Chat Paper
正在生成论文摘要