Self-Expressive Dictionary Learning for Dynamic 3D Reconstruction.
IEEE Transactions on Pattern Analysis and Machine Intelligence(2018)
摘要
We target the problem of sparse 3D reconstruction of dynamic objects observed by multiple unsynchronized video cameras with unknown temporal overlap. To this end, we develop a framework to recover the unknown structure without sequencing information across video sequences. Our proposed compressed sensing framework poses the estimation of 3D structure as the problem of dictionary learning, where the dictionary is defined as an aggregation of the temporally varying 3D structures. Given the smooth motion of dynamic objects, we observe any element in the dictionary can be well approximated by a sparse linear combination of other elements in the same dictionary (i.e., self-expression). Our formulation optimizes a biconvex cost function that leverages a compressed sensing formulation and enforces both structural dependency coherence across video streams, as well as motion smoothness across estimates from common video sources. We further analyze the reconstructability of our approach under different capture scenarios, and its comparison and relation to existing methods. Experimental results on large amounts of synthetic data as well as real imagery demonstrate the effectiveness of our approach.
更多查看译文
关键词
Dictionary learning,self-expression,unsynchronized videos,dynamic 3D reconstruction
AI 理解论文
溯源树
样例
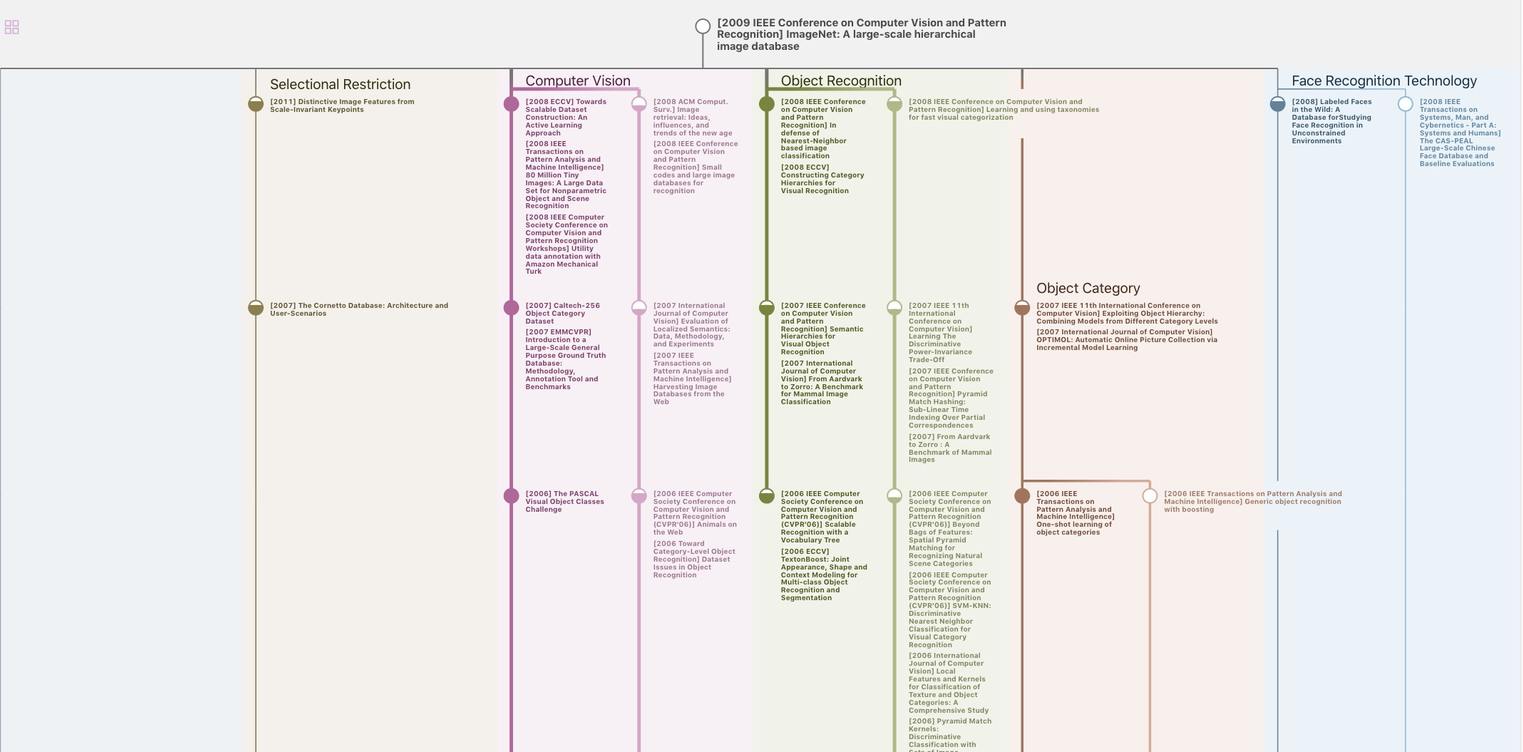
生成溯源树,研究论文发展脉络
Chat Paper
正在生成论文摘要