Recurrent Neural Networks for Dialogue State Tracking.
ITAT(2016)
摘要
This paper discusses models for dialogue state tracking using recurrent neural networks (RNN). We present experiments on the standard dialogue state tracking (DST) dataset, DSTC2. On the one hand, RNN models became the state of the art models in DST, on the other hand, most state-of-the-art models are only turn-based and require dataset-specific preprocessing (e.g. DSTC2-specific) in order to achieve such results. We implemented two architectures which can be used in incremental settings and require almost no preprocessing. We compare their performance to the benchmarks on DSTC2 and discuss their properties. With only trivial preprocessing, the performance of our models is close to the state-of- the-art results.
更多查看译文
AI 理解论文
溯源树
样例
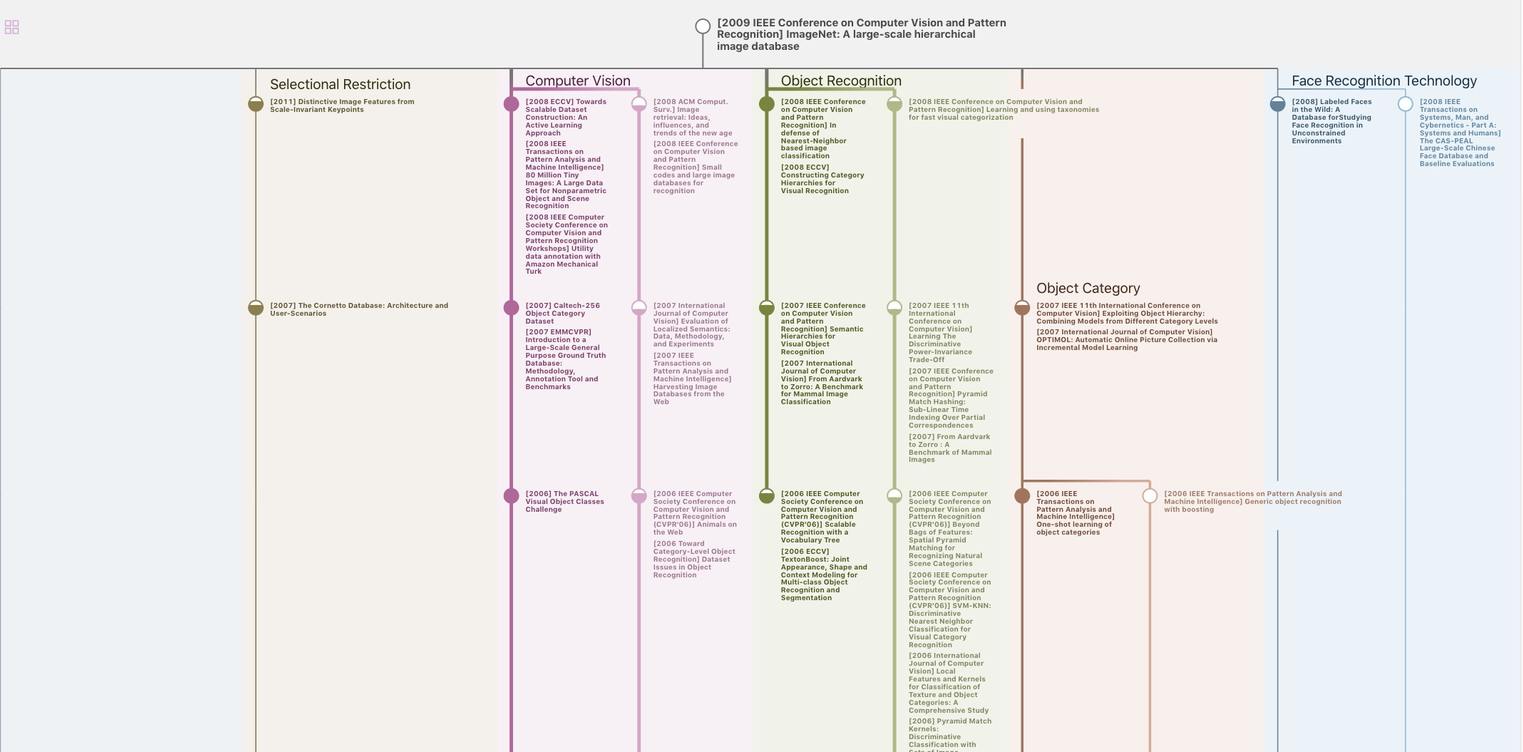
生成溯源树,研究论文发展脉络
Chat Paper
正在生成论文摘要