Learning Multivariate Log-concave Distributions.
COLT(2017)
摘要
We study the problem of estimating multivariate log-concave probability density functions. We prove the first sample complexity upper bound for learning log-concave densities on $mathbb{R}^d$, for all $d geq 1$. Prior to our work, no upper bound on the sample complexity of this learning problem was known for the case of $du003e3$. In more detail, we give an estimator that, for any $d ge 1$ and $epsilonu003e0$, draws $tilde{O}_d left( (1/epsilon)^{(d+5)/2} right)$ samples from an unknown target log-concave density on $mathbb{R}^d$, and outputs a hypothesis that (with high probability) is $epsilon$-close to the target, in total variation distance. Our upper bound on the sample complexity comes close to the known lower bound of $Omega_d left( (1/epsilon)^{(d+1)/2} right)$ for this problem.
更多查看译文
AI 理解论文
溯源树
样例
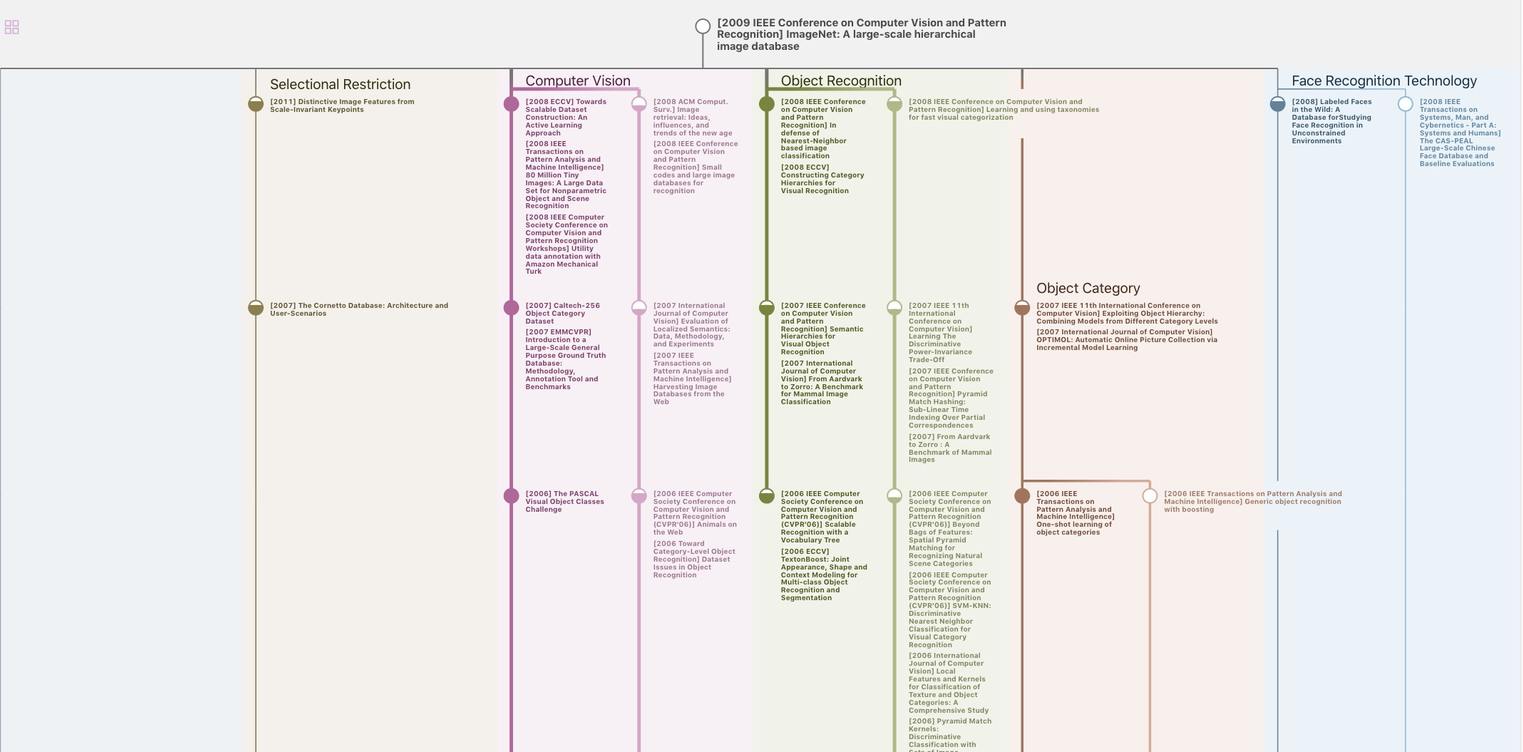
生成溯源树,研究论文发展脉络
Chat Paper
正在生成论文摘要