Network of Experts for Large-Scale Image Categorization
COMPUTER VISION - ECCV 2016, PT VII(2016)
摘要
We present a tree-structured network architecture for large-scale image classification. The trunk of the network contains convolutional layers optimized over all classes. At a given depth, the trunk splits into separate branches, each dedicated to discriminate a different subset of classes. Each branch acts as an expert classifying a set of categories that are difficult to tell apart, while the trunk provides common knowledge to all experts in the form of shared features. The training of our "network of experts" is completely end-to-end: the partition of categories into disjoint subsets is learned simultaneously with the parameters of the network trunk and the experts are trained jointly by minimizing a single learning objective over all classes. The proposed structure can be built from any existing convolutional neural network (CNN). We demonstrate its generality by adapting 3 popular CNNs for image categorization into the form of networks of experts. Our experiments on CIFAR100 and ImageNet show that in each case our method yields a substantial improvement in accuracy over the base CNN, and gives the best reported result on CIFAR100. Finally, the improvement in accuracy comes at little additional cost: compared to the base network, the training time of our model is about 1.5X and the number of parameters is comparable or in some cases even lower.
更多查看译文
关键词
Deep learning,Convolutional networks,Image classification
AI 理解论文
溯源树
样例
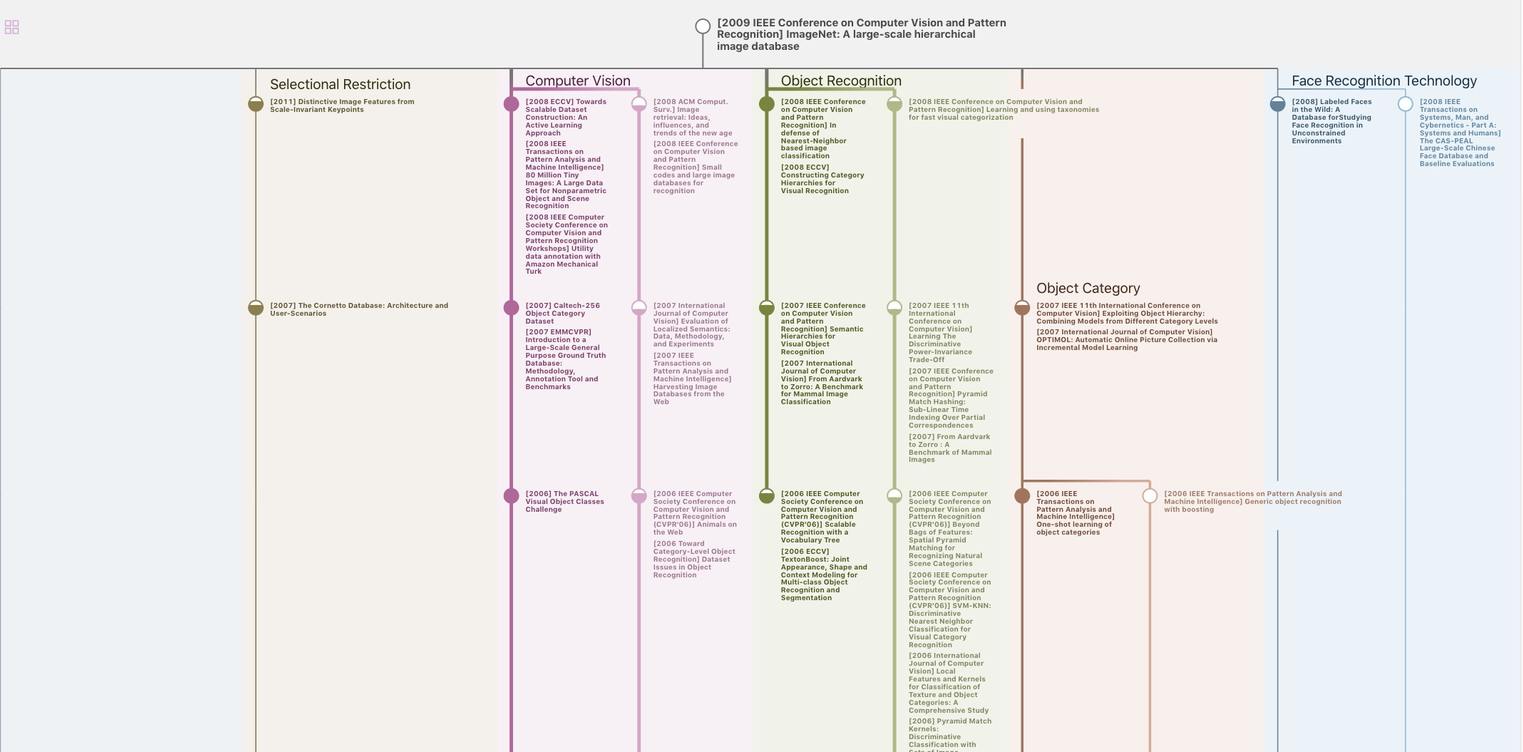
生成溯源树,研究论文发展脉络
Chat Paper
正在生成论文摘要