Gaussian Process Domain Experts for Model Adaptation in Facial Behavior Analysis
2016 IEEE Conference on Computer Vision and Pattern Recognition Workshops (CVPRW)(2016)
摘要
We present a novel approach for supervised domain adaptation that is based upon the probabilistic framework of Gaussian processes (GPs). Specifically, we introduce domain-specific GPs as local experts for facial expression classification from face images. The adaptation of the classifier is facilitated in probabilistic fashion by conditioning the target expert on multiple source experts. Furthermore, in contrast to existing adaptation approaches, we also learn a target expert from available target data solely. Then, a single and confident classifier is obtained by combining the predictions from multiple experts based on their confidence. Learning of the model is efficient and requires no retraining/reweighting of the source classifiers. We evaluate the proposed approach on two publicly available datasets for multi-class (MultiPIE) and multi-label (DISFA) facial expression classification. To this end, we perform adaptation of two contextual factors: 'where' (view) and 'who' (subject). We show in our experiments that the proposed approach consistently outperforms both source and target classifiers, while using as few as 30 target examples. It also outperforms the state-of-the-art approaches for supervised domain adaptation.
更多查看译文
关键词
Gaussian process domain experts,model adaptation,facial behavior analysis,supervised domain adaptation,probabilistic framework,domain-specific GPs,multiclass facial expression classification,face images,confident classifier,multilabel facial expression classification,DISFA,multiPIE
AI 理解论文
溯源树
样例
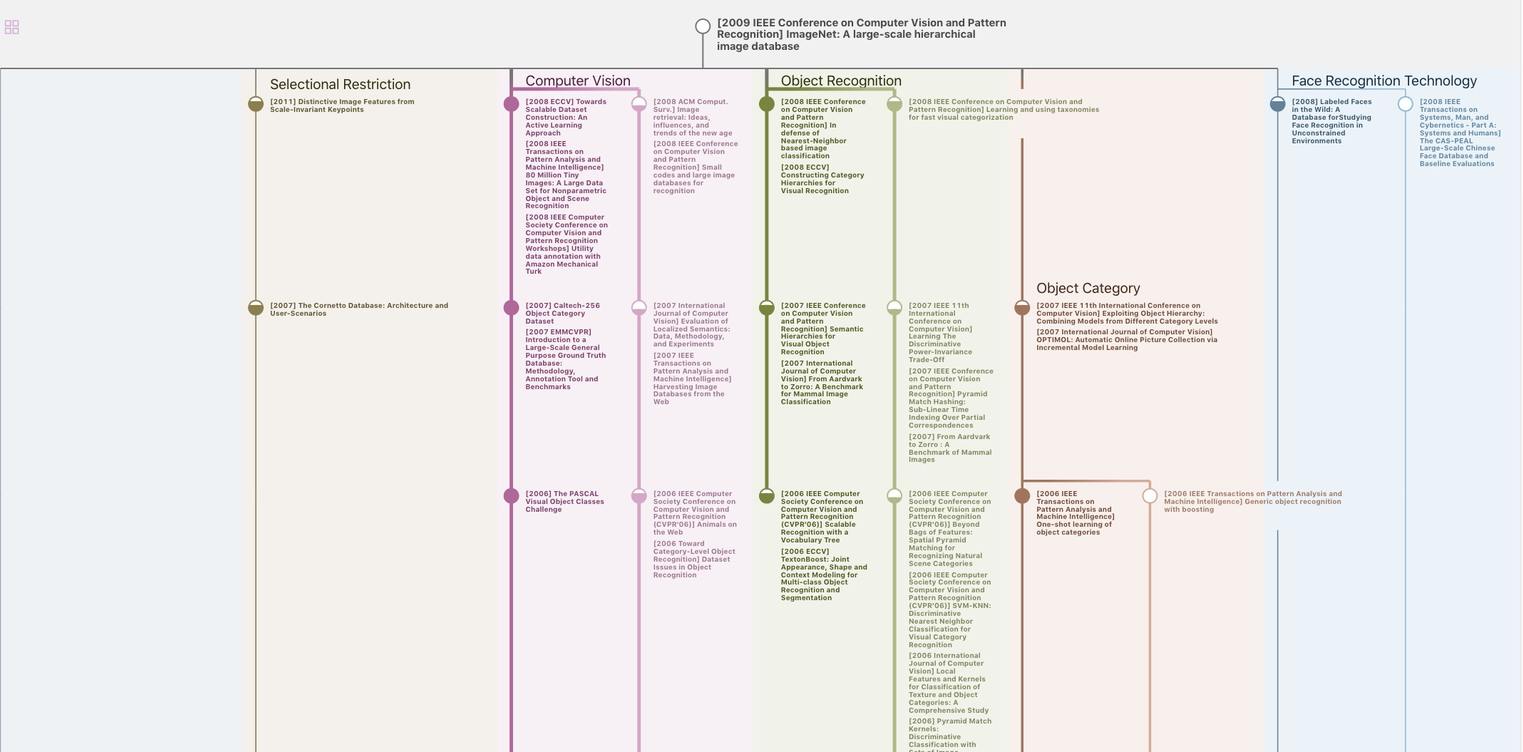
生成溯源树,研究论文发展脉络
Chat Paper
正在生成论文摘要