Adiabatic Persistent Contrastive Divergence Learning
arXiv (Cornell University)(2016)
摘要
This paper studies the problem of parameter learning in probabilistic graphical models having latent variables, where the standard approach is the expectation maximization algorithm alternating expectation (E) and maximization (M) steps. However, both E and M steps are computationally intractable for high dimensional data, while the substitution of one step to a faster surrogate for combating against intractability can often cause failure in convergence. We propose a new learning algorithm which is computationally efficient and provably ensures convergence to a correct optimum. Its key idea is to run only a few cycles of Markov Chains (MC) in both E and M steps. Such an idea of running incomplete MC has been well studied only for M step in the literature, called Contrastive Divergence (CD) learning. While such known CD-based schemes find approximated gradients of the log-likelihood via the mean-field approach in E step, our proposed algorithm does exact ones via MC algorithms in both steps due to the multi-time-scale stochastic approximation theory. Despite its theoretical guarantee in convergence, the proposed scheme might suffer from the slow mixing of MC in E step. To tackle it, we also propose a hybrid approach applying both mean-field and MC approximation in E step, where the hybrid approach outperforms the bare mean-field CD scheme in our experiments on real-world datasets.
更多查看译文
关键词
adiabatic persistent contrastive divergence learning,parameter learning,graphical models,latent variables,expectation maximization algorithm,APCD,Markov chains,multitime-scale stochastic approximation theory,mean-field approximations,MC approximations
AI 理解论文
溯源树
样例
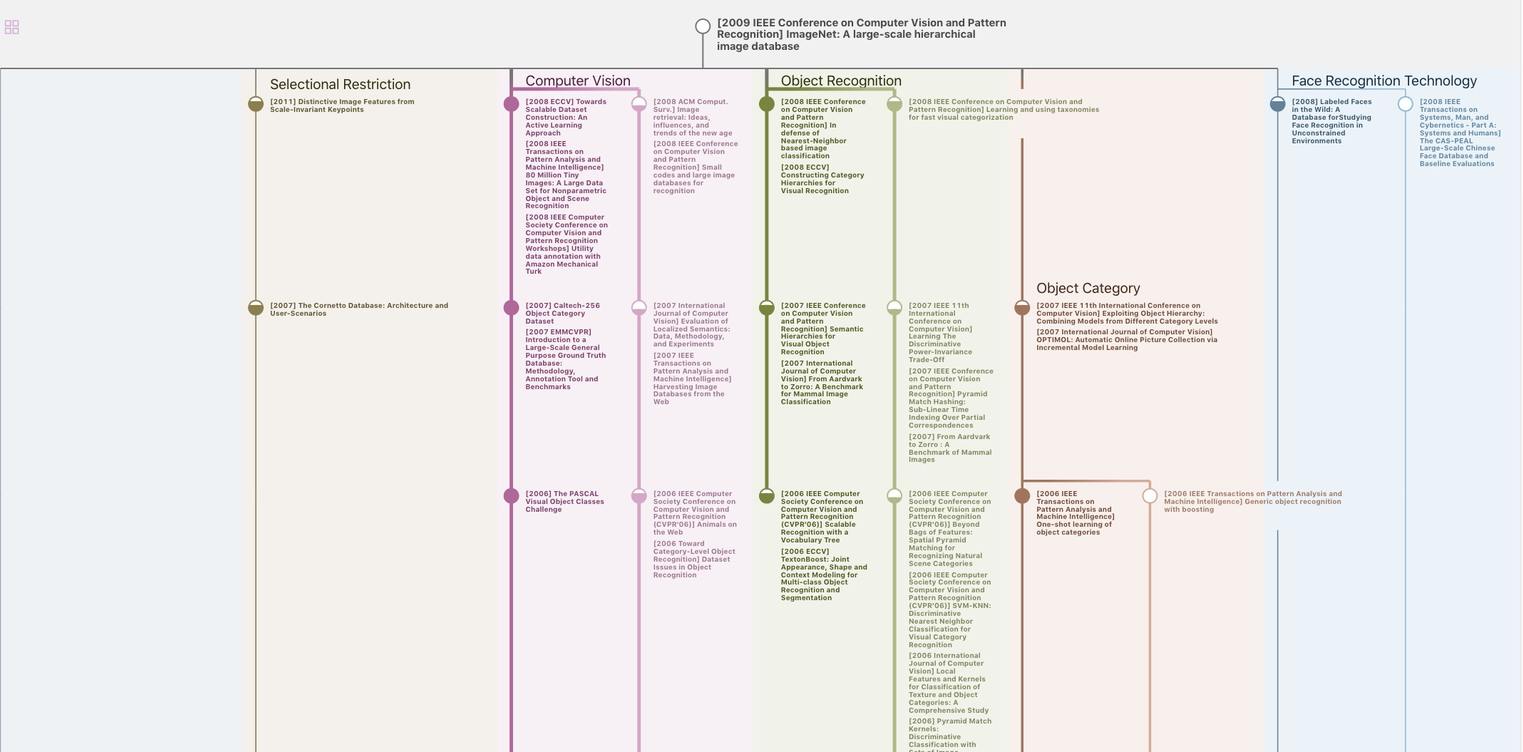
生成溯源树,研究论文发展脉络
Chat Paper
正在生成论文摘要