An approximate message passing approach for compressive hyperspectral imaging using a simultaneous low-rank and joint-sparsity prior
2016 8th Workshop on Hyperspectral Image and Signal Processing: Evolution in Remote Sensing (WHISPERS)(2016)
摘要
This paper considers a compressive sensing (CS) approach for hyperspectral data acquisition, which results in a practical compression ratio substantially higher than the state-of-the-art. Applying simultaneous low-rank and joint-sparse (L&S) model to the hyperspectral data, we propose a novel algorithm to joint reconstruction of hyperspectral data based on loopy belief propagation that enables the exploitation of both structured sparsity and amplitude correlations in the data. Experimental results with real hyperspectral datasets demonstrate that the proposed algorithm outperforms the state-of-the-art CS-based solutions with substantial reductions in reconstruction error.
更多查看译文
关键词
Compressive hyperspectral imaging,low-rank,joint-sparse,compressive sensing,approximate message passing
AI 理解论文
溯源树
样例
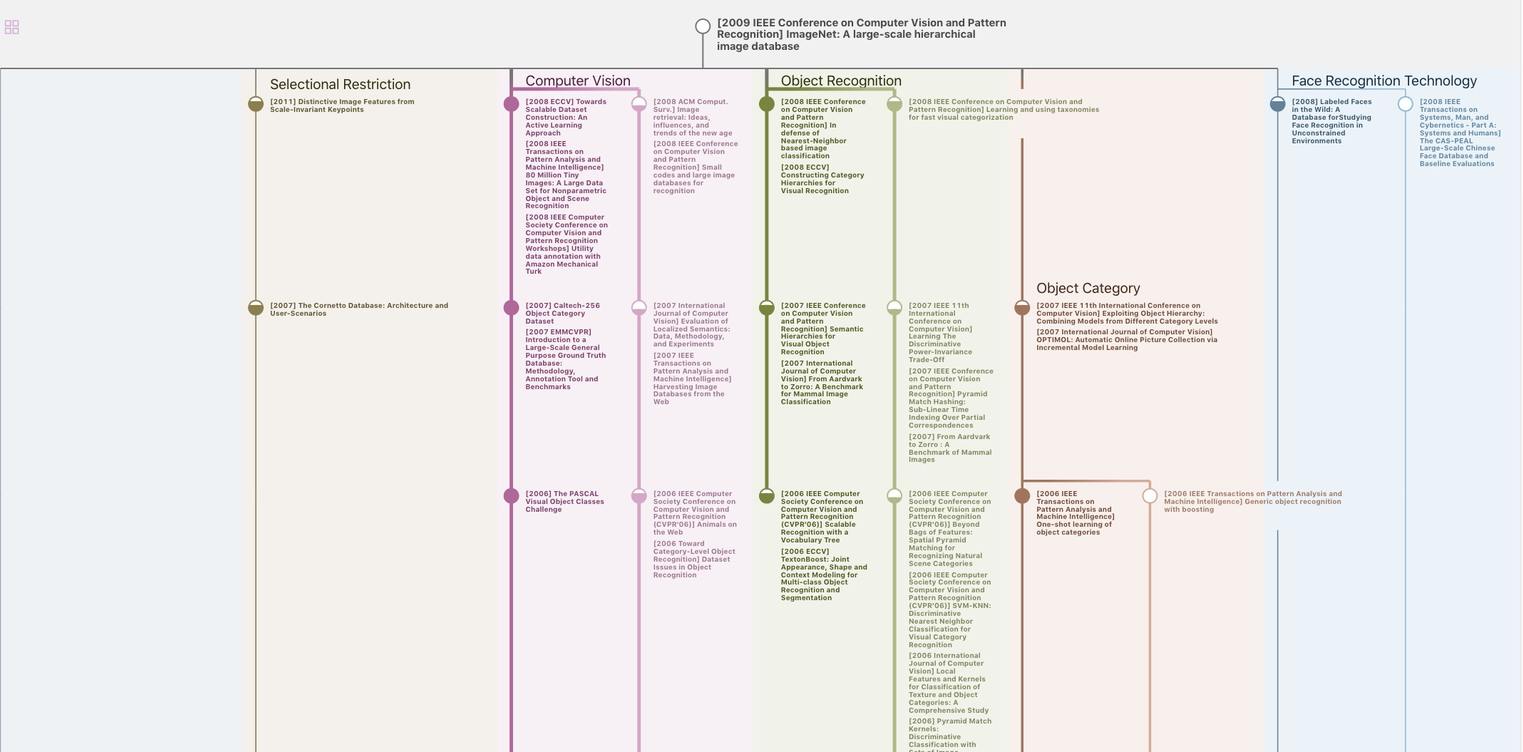
生成溯源树,研究论文发展脉络
Chat Paper
正在生成论文摘要