Energy Stable Model Reduction of Neurons by Nonnegative Discrete Empirical Interpolation.
SIAM JOURNAL ON SCIENTIFIC COMPUTING(2016)
摘要
The accurate and fast prediction of potential propagation in neuronal networks is of prime importance in neurosciences. This work develops a novel structure-preserving model reduction technique to address this problem based on Galerkin projection and nonnegative operator approximation. It is first shown that the corresponding reduced-order model is guaranteed to be energy stable, thanks to both the structure-preserving approach that constructs a distinct reduced-order basis for each cable in the network and the preservation of nonnegativity. Furthermore, a posteriori error estimates are provided, showing that the model reduction error can be bounded and controlled. Finally, the application to the model reduction of a large-scale neuronal network underlines the capability of the proposed approach to accurately predict the potential propagation in such networks while leading to important speedups.
更多查看译文
关键词
model reduction,nonnegative reduced basis,discrete empirical interpolation,Hodgkin-Huxley equation,summation-by-parts operators
AI 理解论文
溯源树
样例
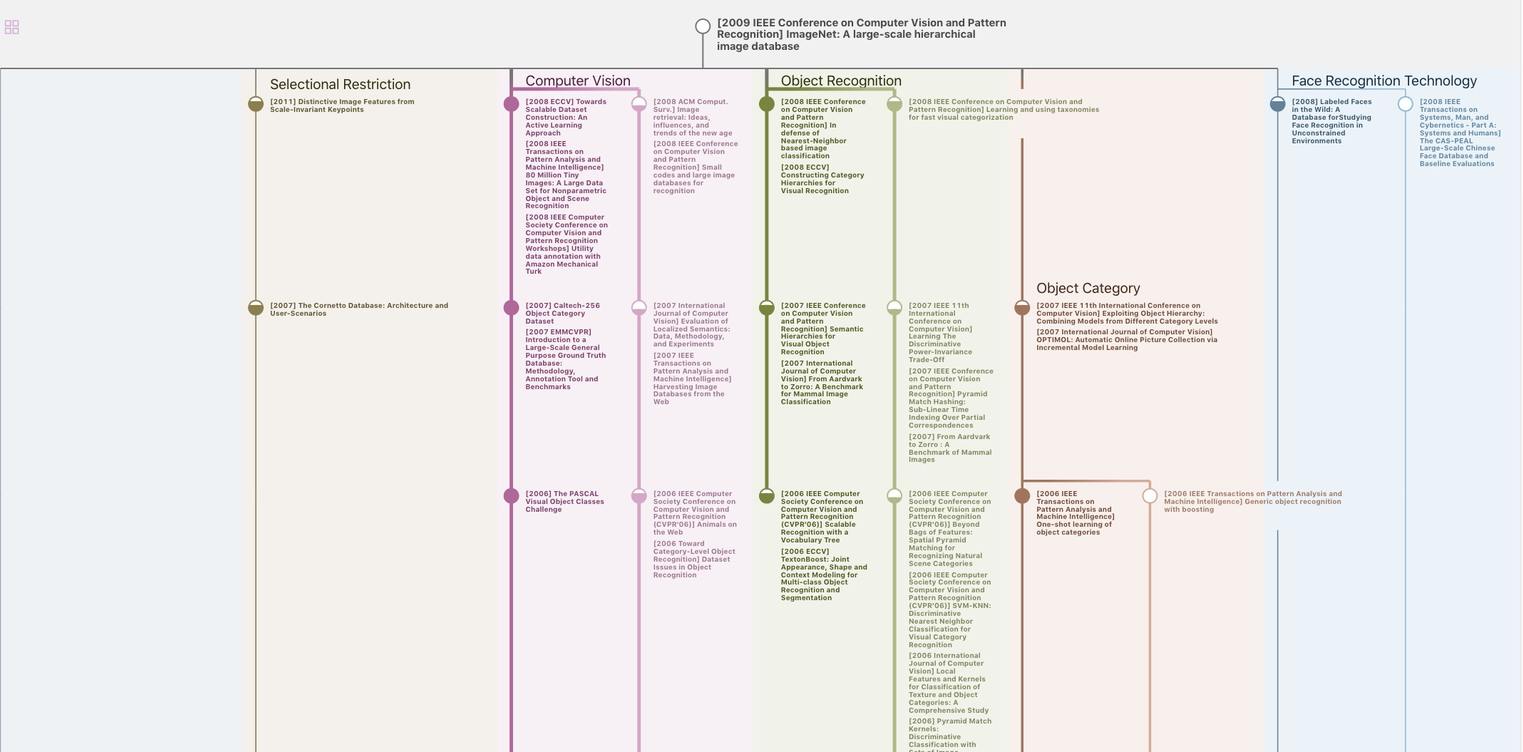
生成溯源树,研究论文发展脉络
Chat Paper
正在生成论文摘要