An adaptive penalty multi-pitch estimator with self-regularization.
Signal Processing(2016)
摘要
This work treats multi-pitch estimation, and in particular the common misclassification issue wherein the pitch at half the true fundamental frequency, the sub-octave, is chosen instead of the true pitch. Extending on current group LASSO-based methods for pitch estimation, this work introduces an adaptive total variation penalty, which enforces both group- and block sparsity, as well as deals with errors due to sub-octaves. Also presented is a scheme for signal adaptive dictionary construction and automatic selection of the regularization parameters. Used together with this scheme, the proposed method is shown to yield accurate pitch estimates when evaluated on synthetic speech data. The method is shown to perform as good as, or better than, current state-of-the-art sparse methods while requiring fewer tuning parameters than these, as well as several conventional pitch estimation methods, even when these are given oracle model orders. When evaluated on a set of ten musical pieces, the method shows promising results for separating multi-pitch signals. HighlightsWe propose a multi-pitch estimator based on a regularized least-squares' problem.The method automatically estimates the number of sources and their model orders.The proposed method incorporates a signal adaptive self-regularization scheme.The proposed method is shown to yield accurate pitch frequency estimates.
更多查看译文
关键词
Multi-pitch estimation,Block sparsity,Adaptive sparse penalty,Self-regularization,ADMM
AI 理解论文
溯源树
样例
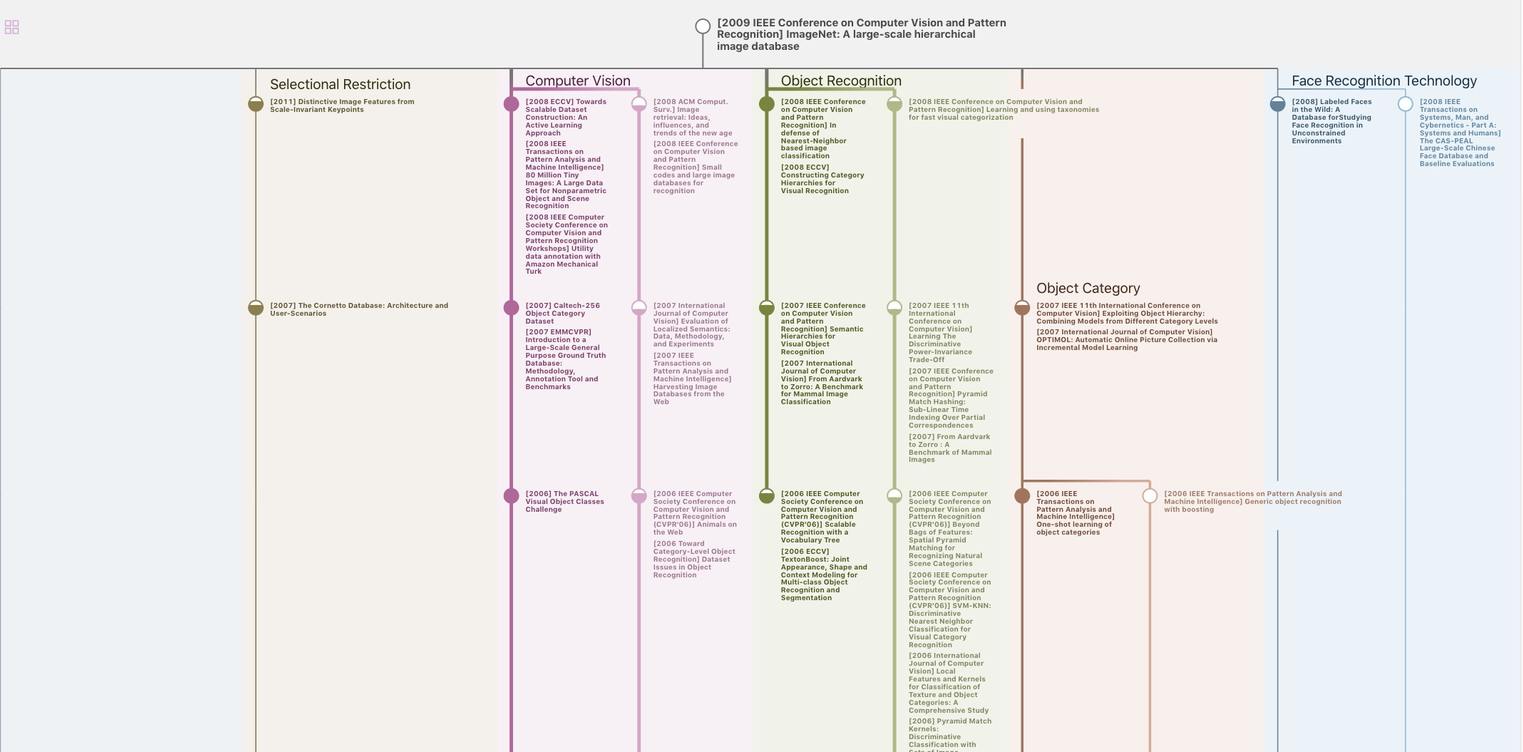
生成溯源树,研究论文发展脉络
Chat Paper
正在生成论文摘要