Improving Short Utterance Speaker Recognition by Modeling Speech Unit Classes.
IEEE/ACM Trans. Audio, Speech & Language Processing(2016)
摘要
Short utterance speaker recognition (SUSR) is highly challenging due to the limited enrollment and/or test data. We argue that the difficulty can be largely attributed to the mismatched prior distributions of the speech data used to train the universal background model (UBM) and those for enrollment and test. This paper presents a novel solution that distributes speech signals into a multitude of acoustic subregions that are defined by speech units, and models speakers within the subregions. To avoid data sparsity, a data-driven approach is proposed to cluster speech units into speech unit classes, based on which robust subregion models can be constructed. Further more, we propose a model synthesis approach based on maximum likelihood linear regression (MLLR) to deal with no-data speech unit classes. The experiments were conducted on a publicly available database SUD12. The results demonstrated that on a text-independent speaker recognition task where the test utterances are no longer than 2 seconds and mostly shorter than 0.5 seconds, the proposed sub-region modeling offered a 21.51% relative reduction in equal error rate (EER), compared with the standard GMM-UBM baseline. In addition, with the model synthesis approach, the performance can be greatly improved in scenarios where no enrollment data are available for some speech unit classes.
更多查看译文
关键词
Speech,Data models,Speaker recognition,Acoustics,Speech recognition,Speech processing,Adaptation models
AI 理解论文
溯源树
样例
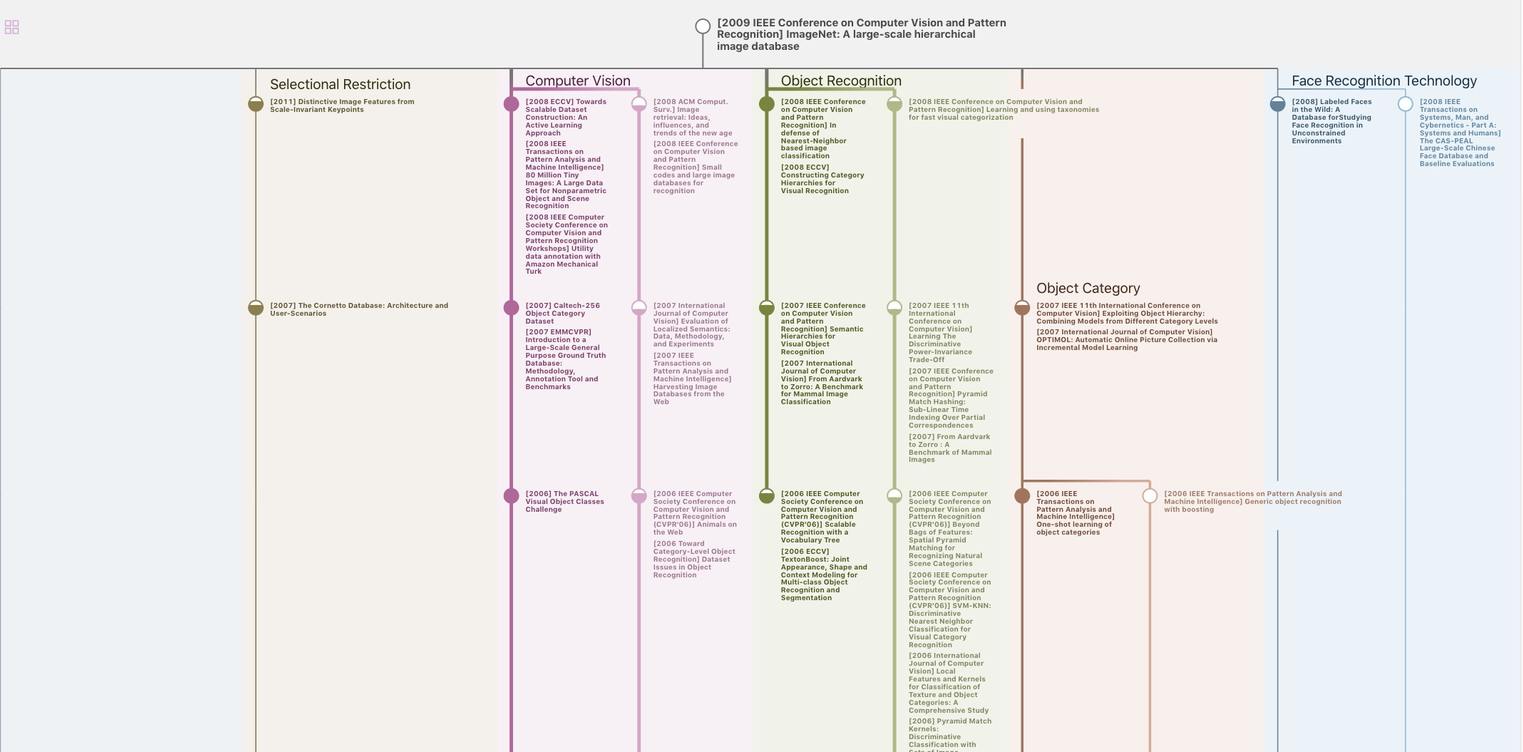
生成溯源树,研究论文发展脉络
Chat Paper
正在生成论文摘要