Graph feature selection for dementia diagnosis.
Neurocomputing(2016)
摘要
This paper proposes a graph feature selection method for dementia diagnosis, by adding the information inherent in the observations into a sparse multi-task learning framework. Specifically, this paper first defines two relations (i.e., the feature-feature relation and the sample-sample relation, respectively) based on the prior knowledge in the data. The feature-feature selection enforces the similarity relationship between features to be preserved in the coefficient matrix while the sample-sample relation is designed to preserve the relation between samples invariant in the predicted space. Then we embed these two kinds of relations into a multi-task learning framework (i.e., a least square loss function plus an l 2 -norm regularization term) to conduct feature selection. Furthermore, we feed the reduced data into Support Vector Machine (SVM) for conducting the identification of Alzheimer's Disease (AD). Finally, the experimental results on a subset of the Alzheimer's Disease Neuroimaging Initiative (ADNI) dataset showed the effectiveness of the proposed method in terms of classification accuracy, by comparing with the state-of-the-art methods, including k Nearest Neighbor (kNN), ridge regression, SVM, and so on.
更多查看译文
关键词
Dementia diagnosis,Feature selection,Manifold learning
AI 理解论文
溯源树
样例
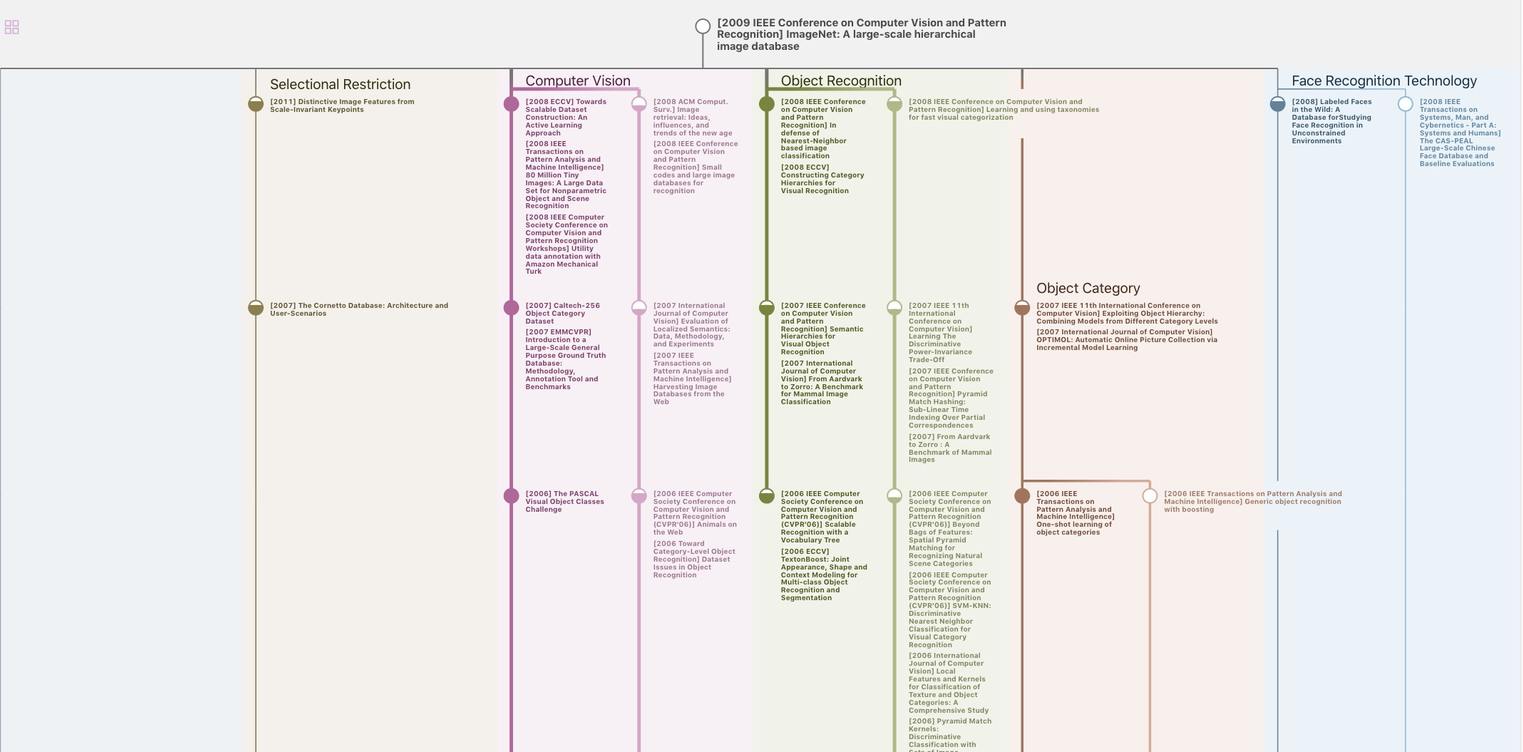
生成溯源树,研究论文发展脉络
Chat Paper
正在生成论文摘要