Multi-view non-negative matrix factorization by patch alignment framework with view consistency.
Neurocomputing(2016)
摘要
Multi-view non-negative matrix factorization (NMF) has been developed to learn the latent representation from multi-view non-negative data in recent years. To make the representation more meaningful, previous works mainly exploit either the consensus information or the complementary information from different views. However, the latent local geometric structure of each view is always ignored. In this paper, we develop a novel multi-view NMF by patch alignment framework with view consistency. Different from previous works, we take the local geometric structure of each view into consideration, and penalize the disagreement of different views at the same time. More specifically, given a data in each view, we construct a local patch utilizing locally linear embedding to preserve its local geometrical structure, and obtain the global representation under the whole alignment strategy. Meanwhile, for different views, we make the representations of views to approximate the latent representation shared by different views via considering the view consistency. We adopt the correntropy-induced metric to measure the reconstruction error and employ the half-quadratic technique to solve the optimization problem. The experimental results demonstrate the proposed method can achieve satisfactory performance compared with single-view methods and other existing multi-view NMF methods.
更多查看译文
关键词
Multi-view non-negative matrix factorization,Patch alignment framework,Geometric structure,Locally linear embedding,View consistency
AI 理解论文
溯源树
样例
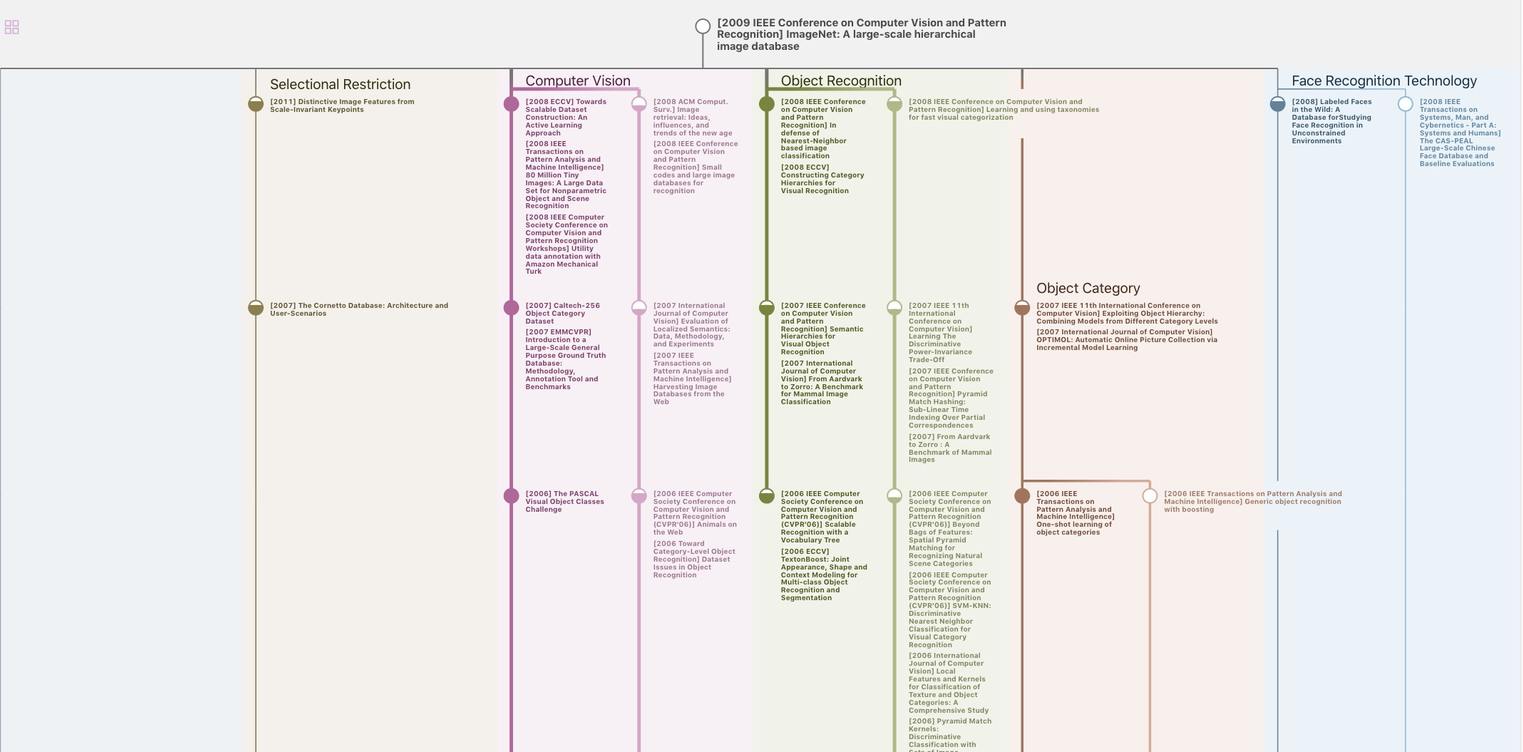
生成溯源树,研究论文发展脉络
Chat Paper
正在生成论文摘要