The Orthogonally Partitioned Em Algorithm: Extending The Em Algorithm For Algorithmic Stability And Bias Correction Due To Imperfect Data
INTERNATIONAL JOURNAL OF BIOSTATISTICS(2016)
摘要
We propose an extension of the EM algorithm that exploits the common assumption of unique parameterization, corrects for biases due to missing data and measurement error, converges for the specified model when standard implementation of the EM algorithm has a low probability of convergence, and reduces a potentially complex algorithm into a sequence of smaller, simpler, self-contained EM algorithms. We use the theory surrounding the EM algorithm to derive the theoretical results of our proposal, showing that an optimal solution over the parameter space is obtained. A simulation study is used to explore the finite sample properties of the proposed extension when there is missing data and measurement error. We observe that partitioning the EM algorithm into simpler steps may provide better bias reduction in the estimation of model parameters. The ability to breakdown a complicated problem in to a series of simpler, more accessible problems will permit a broader implementation of the EM algorithm, permit the use of software packages that now implement and/or automate the EM algorithm, and make the EM algorithm more accessible to a wider and more general audience.
更多查看译文
关键词
EM algorithm, missing data, measurement error, imperfect data, bias correction
AI 理解论文
溯源树
样例
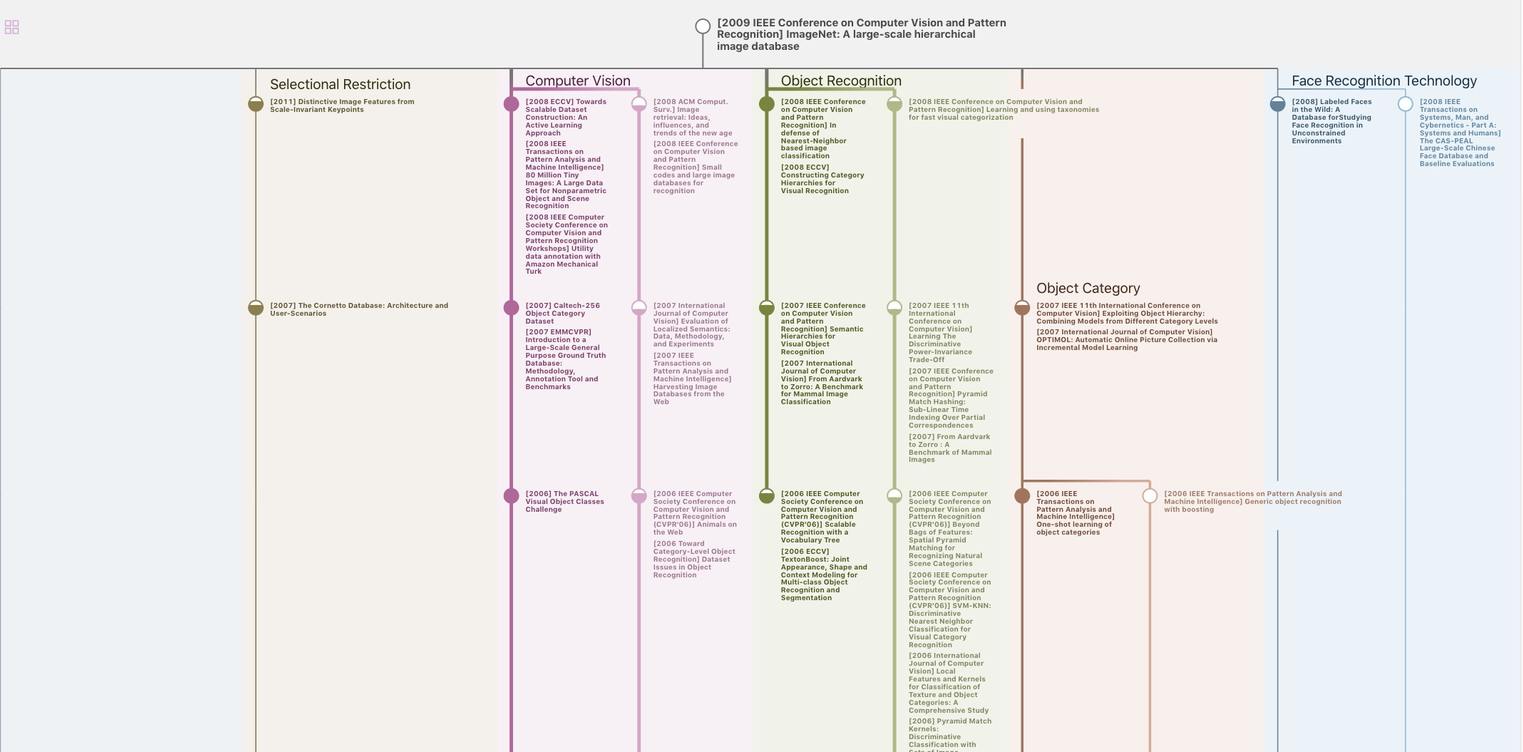
生成溯源树,研究论文发展脉络
Chat Paper
正在生成论文摘要