Performance characterization of self-calibrating protocols for wearable EEG applications
International Conference on Pervasive Technologies Related to Assistive Environments(2015)
摘要
Therapeutic Neurofeedback (NFB) using real-time electroencephalography (EEG) data works by reinforcing desired brainwave patterns. Although EEG is a well-established diagnostic tool and EEG-NFB shows great promise for enhancing cognitive performance and treating neurological disorders, proof of its efficacy has been limited. Here we characterize a novel Self-Calibrating Protocol (SCP) method coupled to five standard machine learning algorithms to classify brain states corresponding to the experience of \"pain\" or \"no pain\". Our results indicate that commercially available, wearable EEG sensors provide sufficient data fidelity to robustly differentiate the two \"perceptually opposite\" brain states. Crucially, use of SCP allows us for the first time to bypass the pitfalls associated with trying to force an individual's brain wave patterns to match \"normed\" target patterns obtained over population averages. These are necessary steps towards personalized NFB therapies and bespoke Brain-Computer Interfaces and brain training suitable to a wide variety of individual needs.
更多查看译文
关键词
EEG, neurofeedback, biofeedback, self-calibrating protocols, wearable sensors
AI 理解论文
溯源树
样例
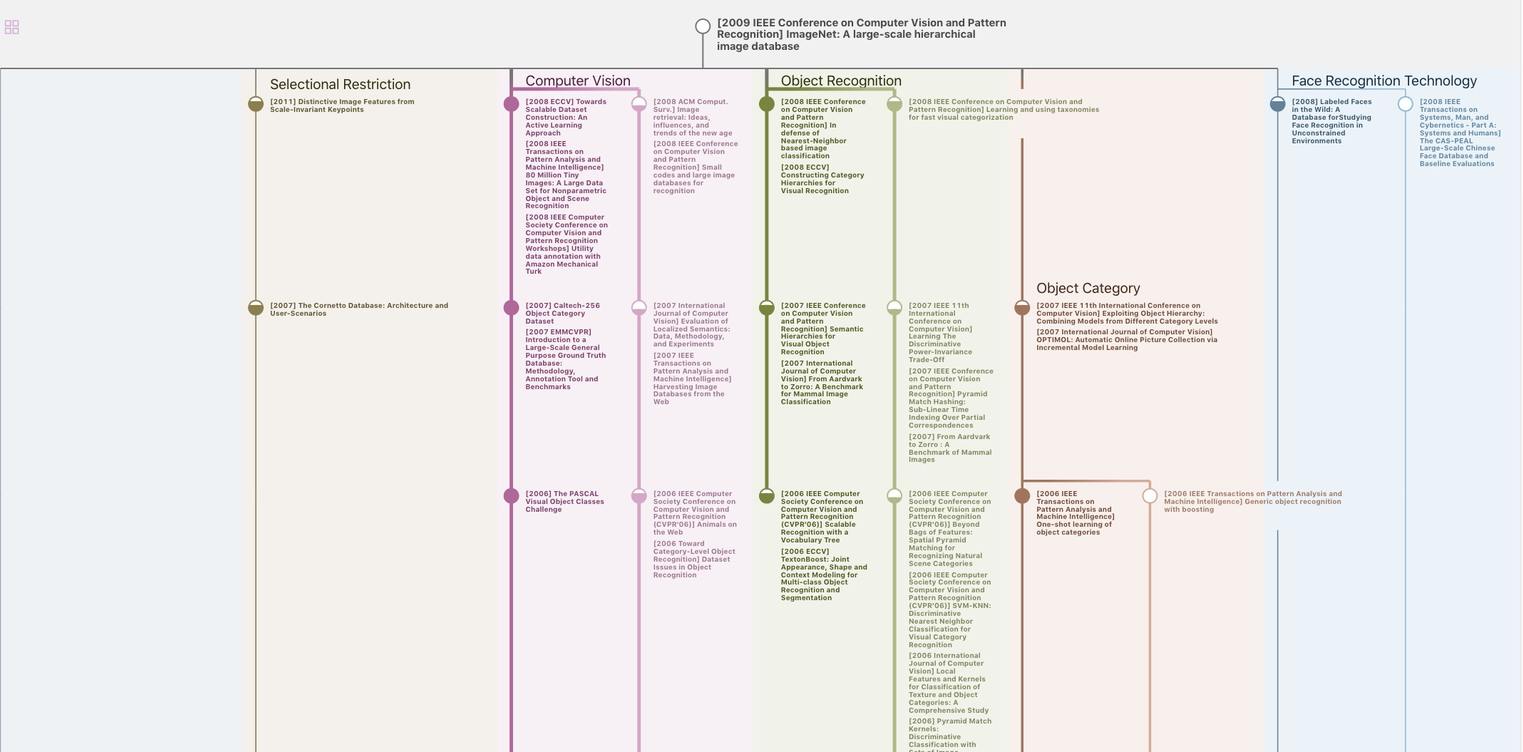
生成溯源树,研究论文发展脉络
Chat Paper
正在生成论文摘要