Multiway Histogram Intersection For Multi-Target Tracking
2015 18TH INTERNATIONAL CONFERENCE ON INFORMATION FUSION (FUSION)(2015)
摘要
In recent studies of multi-target tracking, high-order association and its corresponding high-order affinity (or similarity) is often preferred over pairwise comparisons to capture high-order discriminative information. A naturally raised challenge is to calculate affinity (or similarity) among more than two target candidates. When target appearance is represented by histograms, such as the popular SIFT and HOG descriptors, pairwise matching measurements, such as Histogram Intersection (HI) etc. are often combined to fit the high-order request in an ad hoc way. However, such combinations may be ineffective and inefficient.In this paper, we address the pairwise matching issue by proposing a novel multi-histogram similarity named Multiway Histogram Intersection (MHI). MHI naturally extends HI by summing over the "min" value of all histograms in each bin. MHI applies to any number of histograms, fits the request of multi-target tracking better and requires less time than previously used affinities. To demonstrate its superiority, we integrate MHI into a recently proposed rank-1-tensor-approximation multi-tracking framework and apply it to vehicle tracking in wide aerial video surveillance. The advantage of using MHI is clearly supported by the experimental results against six common approaches on two public benchmark datasets.
更多查看译文
关键词
multiway histogram intersection,feature comparison,tensor,multi-target tracking,WAMI
AI 理解论文
溯源树
样例
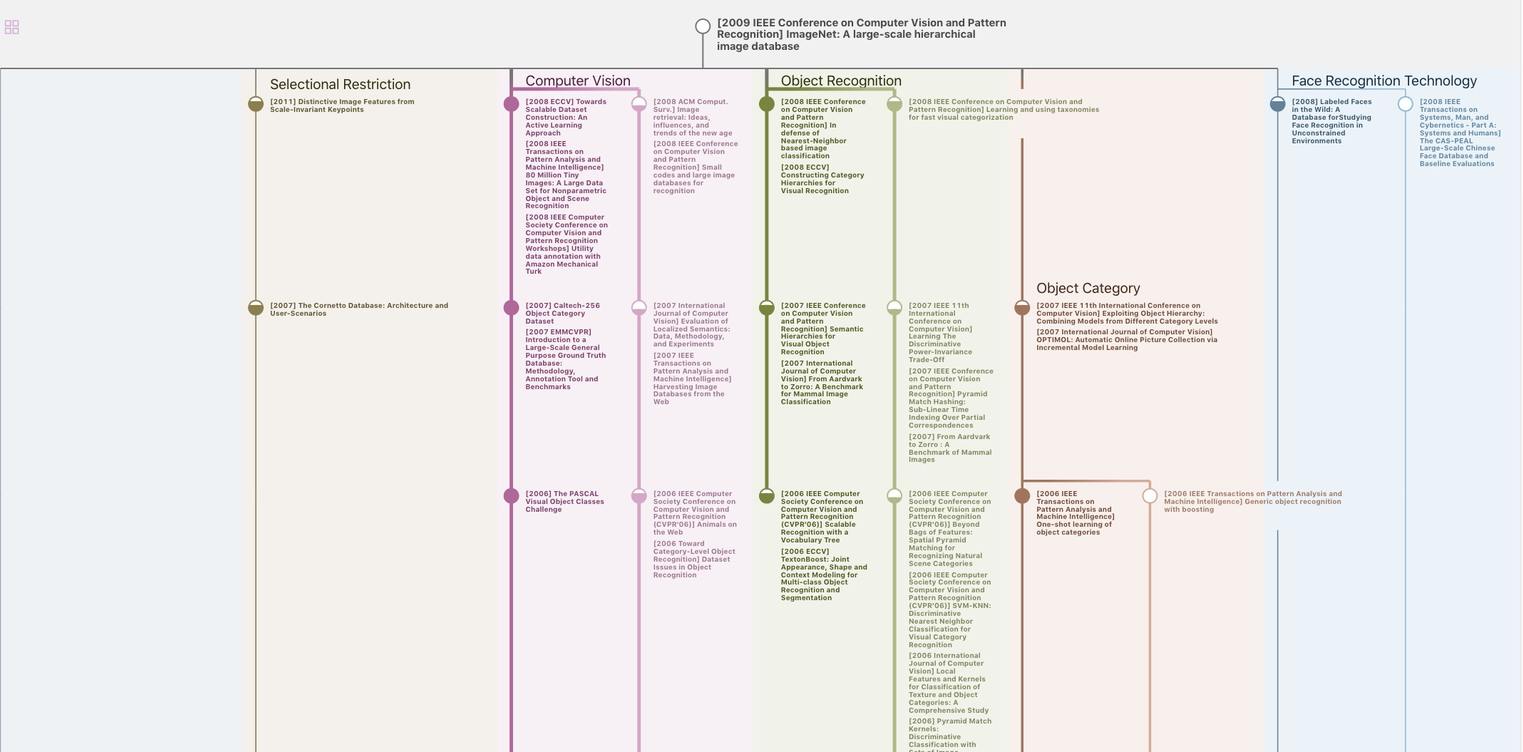
生成溯源树,研究论文发展脉络
Chat Paper
正在生成论文摘要