Entropy clustering approach for improving forecasting in DDoS attacks
ICNSC(2015)
摘要
Volume anomaly such as distributed denial-of-service (DDoS) has been around for ages but with advancement in technologies, they have become stronger, shorter and weapon of choice for attackers. Digital forensic analysis of intrusions using alerts generated by existing intrusion detection system (IDS) faces major challenges, especially for IDS deployed in large networks. In this paper, the concept of automatically sifting through a huge volume of alerts to distinguish the different stages of a DDoS attack is developed. The proposed novel framework is purpose-built to analyze multiple logs from the network for proactive forecast and timely detection of DDoS attacks, through a combined approach of Shannon-entropy concept and clustering algorithm of relevant feature variables. Experimental studies on a cyber-range simulation dataset from the project industrial partners show that the technique is able to distinguish precursor alerts for DDoS attacks, as well as the attack itself with a very low false positive rate (FPR) of 22.5%. Application of this technique greatly assists security experts in network analysis to combat DDoS attacks.
更多查看译文
关键词
alert management, distributed denial-of-service (DDoS) detection, k-means clustering analysis, network security, online anomaly detection, Shannon entropy
AI 理解论文
溯源树
样例
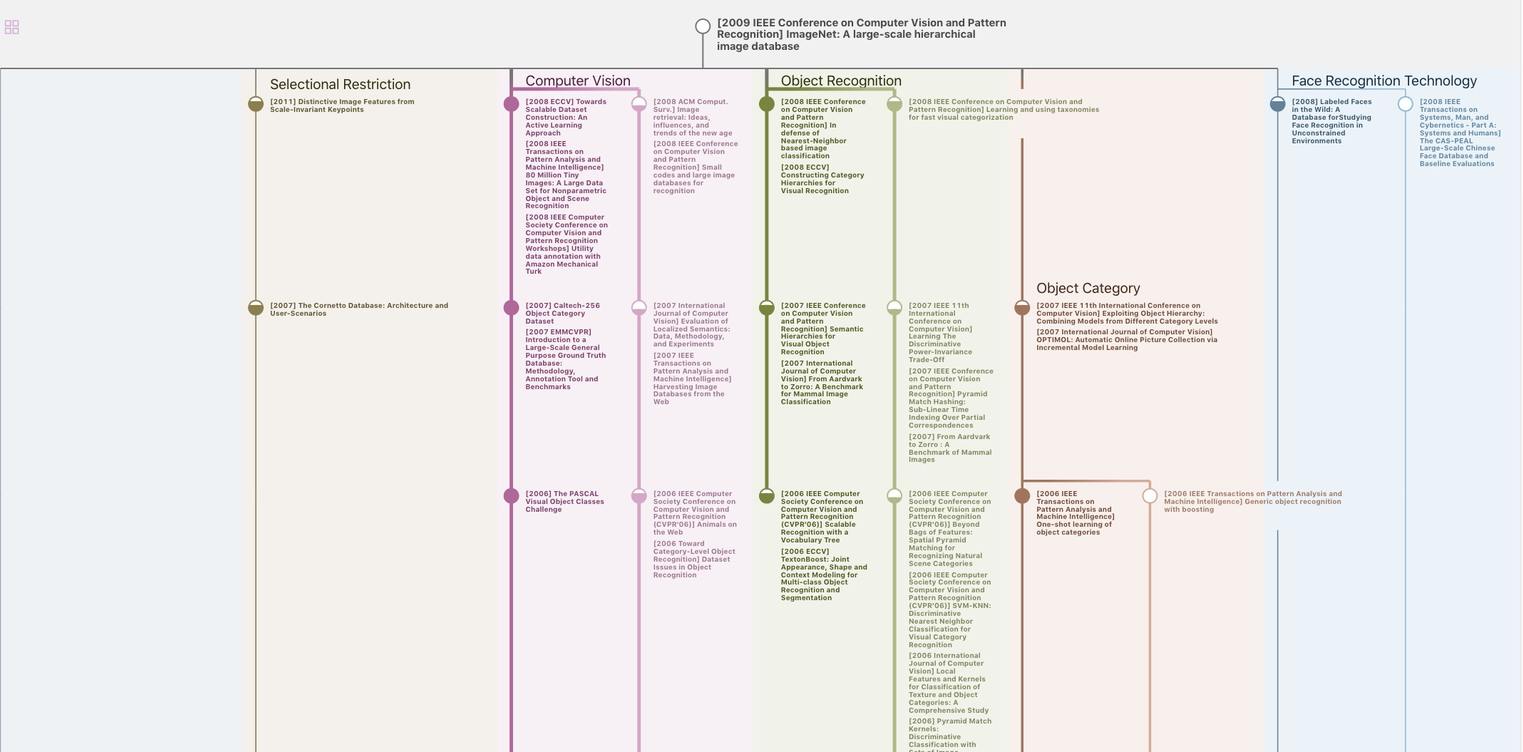
生成溯源树,研究论文发展脉络
Chat Paper
正在生成论文摘要