Increasing Grid Flexibility Through Improved Electricity Demand Prediction in Nicaragua
2015 IEEE 14th International Conference on Machine Learning and Applications (ICMLA)(2015)
摘要
Renewable energy provides an increasingly significant contribution to power production around the globe. The variable and uncertain nature of certain renewable energy sources, however, requires increased grid flexibility to reliably match electricity supply with demand. On average, wind energy accounts for 20% of Nicaragua's total generation, and can produce up to 50% within a given hour. Under the renewable energy regime fuel-oil generators are the main source of grid flexibility. Information-driven flexibility, such as improved demand prediction, can be used to reduce the need of fuel-oil based flexibility without affecting reliability. This paper evaluates and compares the use of multiple linear regression (MLR) and support vector regression (SVR) in their ability to minimize electricity demand forecast error in Nicaragua. We find SVR reduces the mean absolute percent error of prediction to 3.8%, compared with MLR (7.7%). SVR further performs a prediction with 21% less error than the current prediction mechanism employed by the utility. Finally, we discuss how improved prediction algorithms can be used to reduce Nicaragua's dependency on fuel-oil for flexibility, while also reducing costs for the utility.
更多查看译文
关键词
grid flexibility,STLF,SVR,MLR,Nicaragua
AI 理解论文
溯源树
样例
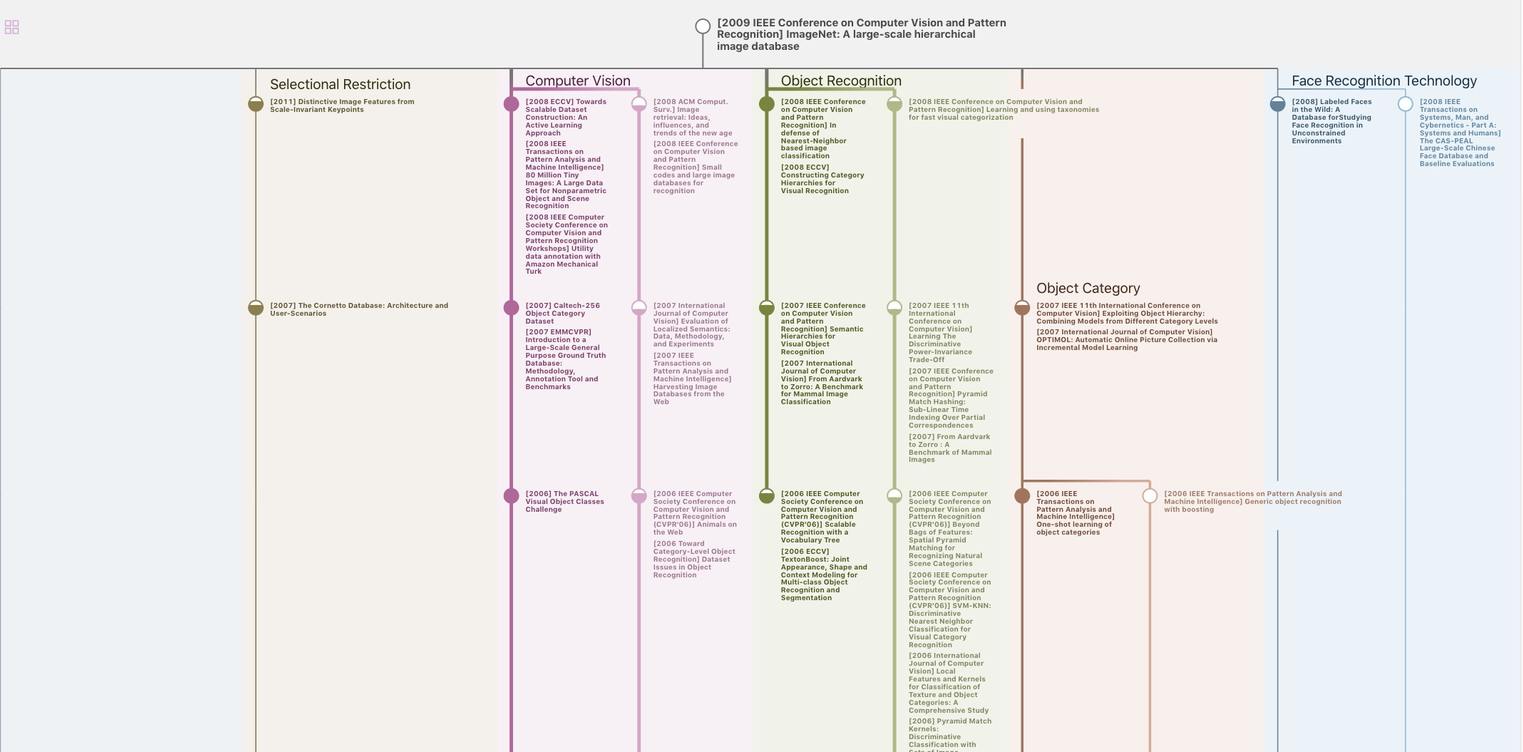
生成溯源树,研究论文发展脉络
Chat Paper
正在生成论文摘要