Basic Statistics of SIFT Features for Texture Analysis.
Bildverarbeitung für die Medizin(2016)
摘要
This paper presents an evaluation of two methods using Scale Invariant Feature Transform (SIFT) for texture analysis, in order to classify malaria parasites versus leukocytes in thick blood smears. In the first approach, the texture is represented by basic statistics of SIFT features like the mean value and standard deviation. The second technique is a vector-quantization approach called bag of visual words, which uses k-means clustering for creating one feature vector per image. Here, the number of clusters is the crucial variable, which is analyzed in this contribution. Furthermore, we compare two different ways to choose the SIFT keypoints. The default SIFT keypoint detector is performed, or a dense grid of equally distributed points in the image determines the location of the keypoints. The results show that grid keypoints yield the best performance and classification in general is possible with both methods using SIFT.
更多查看译文
关键词
Feature Vector, Visual Word, Scale Invariant Feature Transform, Thick Blood Smear, Spatial Pyramid Match
AI 理解论文
溯源树
样例
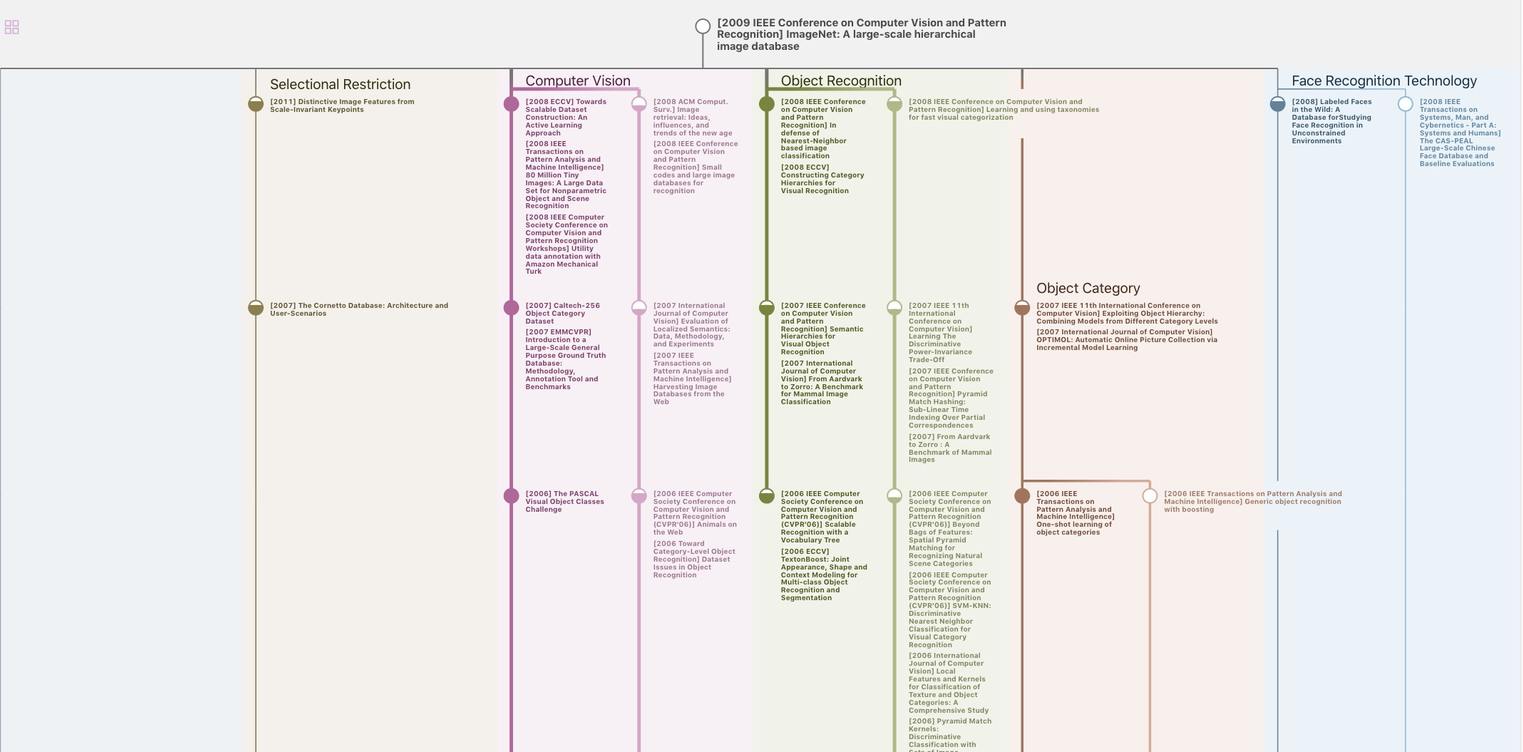
生成溯源树,研究论文发展脉络
Chat Paper
正在生成论文摘要