Bayesian Differential Privacy On Correlated Data
MOD(2015)
摘要
Differential privacy provides a rigorous standard for evaluating the privacy of perturbation algorithms. It has widely been regarded that differential privacy is a universal definition that deals with both independent and correlated data and a differentially private algorithm can protect privacy against arbitrary adversaries. However, recent research indicates that differential privacy may not guarantee privacy against arbitrary adversaries if the data are correlated.In this paper, we focus on the private perturbation algorithms on correlated data. We investigate the following three problems: (1) the influence of data correlations on privacy; (2) the influence of adversary prior knowledge on privacy; and (3) a general perturbation algorithm that is private for prior knowledge of any subset of tuples in the data when the data are correlated. We propose a Pufferfish definition of privacy, called Bayesian differential privacy, by which the privacy level of a probabilistic perturbation algorithm can be evaluated even when the data are correlated and when the prior knowledge is incomplete. We present a Gaussian correlation model to accurately describe the structure of data correlations and analyze the Bayesian differential privacy of the perturbation algorithm on the basis of this model. Our results show that privacy is poorest for an adversary who has the least prior knowledge. We further extend this model to a more general one that considers uncertain prior knowledge.
更多查看译文
关键词
Private data analysis,Differential privacy,Output perturbation,Gaussian Markov random field,Optimization
AI 理解论文
溯源树
样例
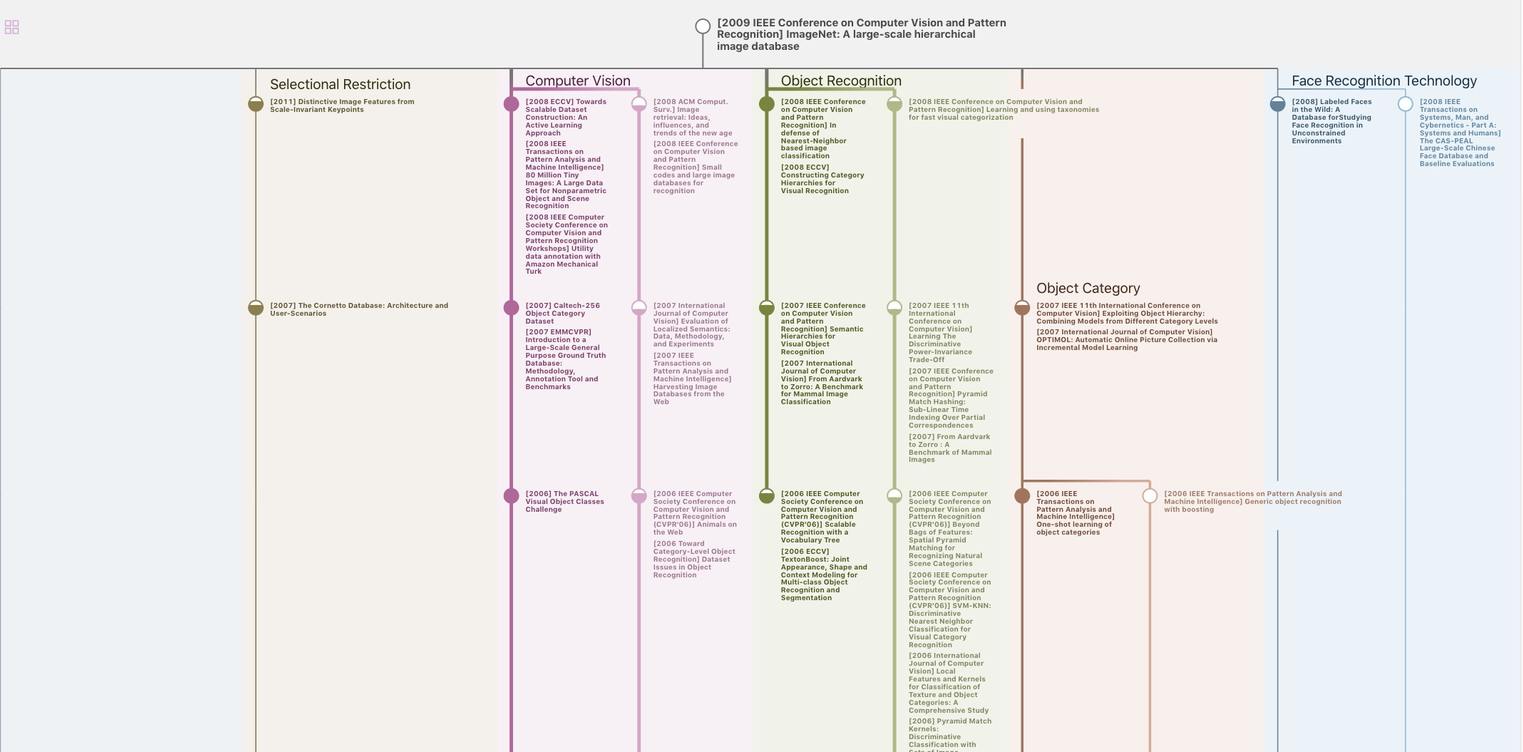
生成溯源树,研究论文发展脉络
Chat Paper
正在生成论文摘要