Learning to Represent Knowledge Graphs with Gaussian Embedding.
CIKM(2015)
摘要
ABSTRACTThe representation of a knowledge graph (KG) in a latent space recently has attracted more and more attention. To this end, some proposed models (e.g., TransE) embed entities and relations of a KG into a "point" vector space by optimizing a global loss function which ensures the scores of positive triplets are higher than negative ones. We notice that these models always regard all entities and relations in a same manner and ignore their (un)certainties. In fact, different entities and relations may contain different certainties, which makes identical certainty insufficient for modeling. Therefore, this paper switches to density-based embedding and propose KG2E for explicitly modeling the certainty of entities and relations, which learn the representations of KGs in the space of multi-dimensional Gaussian distributions. Each entity/relation is represented by a Gaussian distribution, where the mean denotes its position and the covariance (currently with diagonal covariance) can properly represent its certainty. In addition, compared with the symmetric measures used in point-based methods, we employ the KL-divergence for scoring triplets, which is a natural asymmetry function for effectively modeling multiple types of relations. We have conducted extensive experiments on link prediction and triplet classification with multiple benchmark datasets (WordNet and Freebase). Our experimental results demonstrate that our method can effectively model the (un)certainties of entities and relations in a KG, and it significantly outperforms state-of-the-art methods (including TransH and TransR).
更多查看译文
AI 理解论文
溯源树
样例
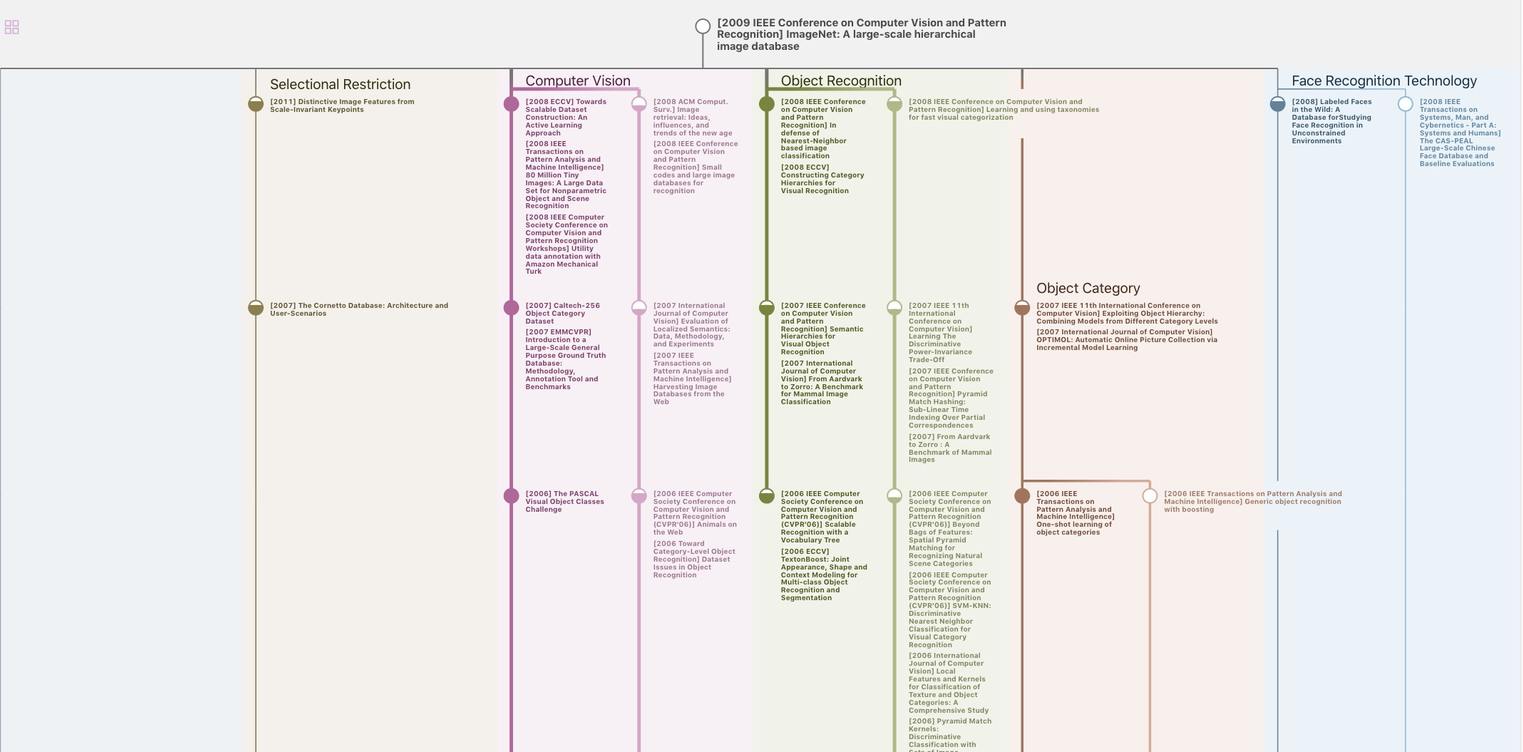
生成溯源树,研究论文发展脉络
Chat Paper
正在生成论文摘要