Evolutionary Semi-Supervised Ordinal Regression Using Weighted Kernel Fisher Discriminant Analysis
2015 IEEE Congress on Evolutionary Computation (CEC)(2015)
摘要
Ordinal regression has a wide range of applications, while it is intractable to be solved when lacking sufficient labeled data. In this paper, we propose an evolutionary semi-supervised kernel Fisher discriminant approach for ordinal regression. The proposed algorithm obtains the projection and thresholds by incorporating the unlabeled data with a weighting scheme, where the weights indicate the degrees of contributions to the class distribution by different training instances. The projection maps the original data to a one-dimensional space, and the thresholds are used for the prediction. The weights are computed with a label propagation method first. However, it is not always accurate. In order to further tune the weights to be more accurate, the differential evolution algorithm is applied here in this work. By a delicate weight update rule, the weights can be evolved indirectly. This tuning scheme makes the size of evolutionary individual just associated with the number of ranks rather than the number of instances. The experimental studies demonstrate that our algorithm can effectively use unlabeled data and yield satisfactory learning performance.
更多查看译文
关键词
evolutionary semisupervised ordinal regression,weighted kernel Fisher discriminant analysis,sufficient labeled data,evolutionary semisupervised kernel Fisher discriminant approach,weighting scheme,projection maps,one-dimensional space,label propagation method,differential evolution algorithm,weight update rule
AI 理解论文
溯源树
样例
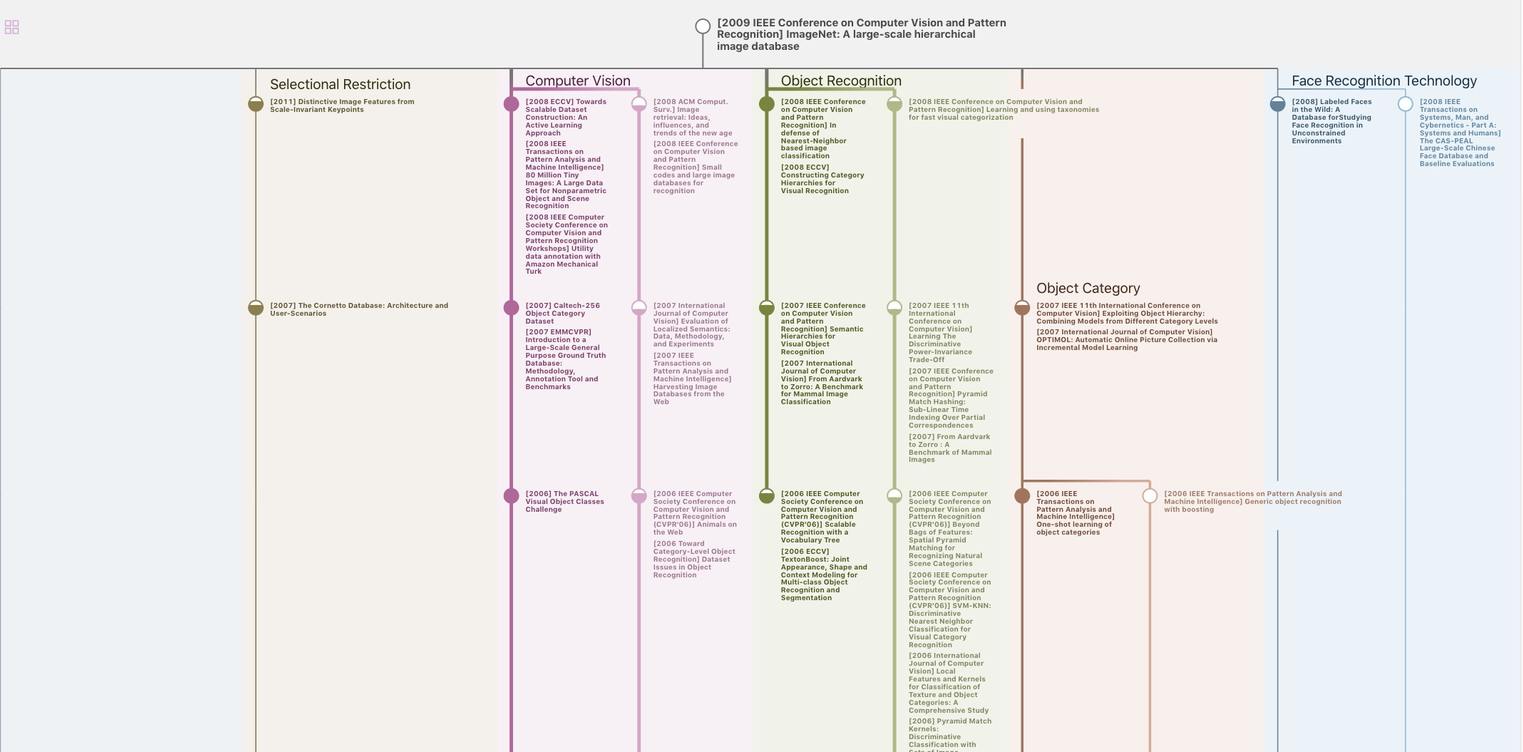
生成溯源树,研究论文发展脉络
Chat Paper
正在生成论文摘要