Stochastic Least Squares Learning For Deep Architectures
2015 International Joint Conference on Neural Networks (IJCNN)(2015)
摘要
In this paper, we present a novel way of pre-training deep architectures by using the stochastic least squares autoencoder (SLSA). The SLSA is based on the combination of stochastic least squares estimation and logistic sampling. The usefulness of the stochastic least squares approach coupled with the numerical trick of constraining the logistic sampling process is highlighted in this paper. This approach was tested and bench marked against other methods including Neural Nets (NN), Deep Belief Nets (DBN), and Stacked Denoising Autoencoder (SDAE) using the MNIST dataset. In addition, the SLSA architecture was also tested against established methods such as the Support Vector Machine (SVM), and the Naive Bayes Classifier (NB) on the Reuters-21578 and MNIST datasets. The experiments show the promise of SLSA as a pre-training step, in which stacked of SLSA yielded the lowest classification error and the highest F-measure scores on the MNIST and Reuters-21578 datasets respectively. Hence, this paper establishes the value of pre-training deep neural network, by using the SLSA.
更多查看译文
关键词
stochastic least squares learning,pretraining deep-architectures,stochastic least squares autoencoder,stochastic least squares estimation,logistic sampling process,neural nets,deep belief nets,stacked denoising autoencoder,NN,DBN,SDAE,MNIST dataset,support vector machine,SVM,naive Bayes classifier,NB,Reuters-21578 dataset,classification error,F-measure score,SLSA architecture
AI 理解论文
溯源树
样例
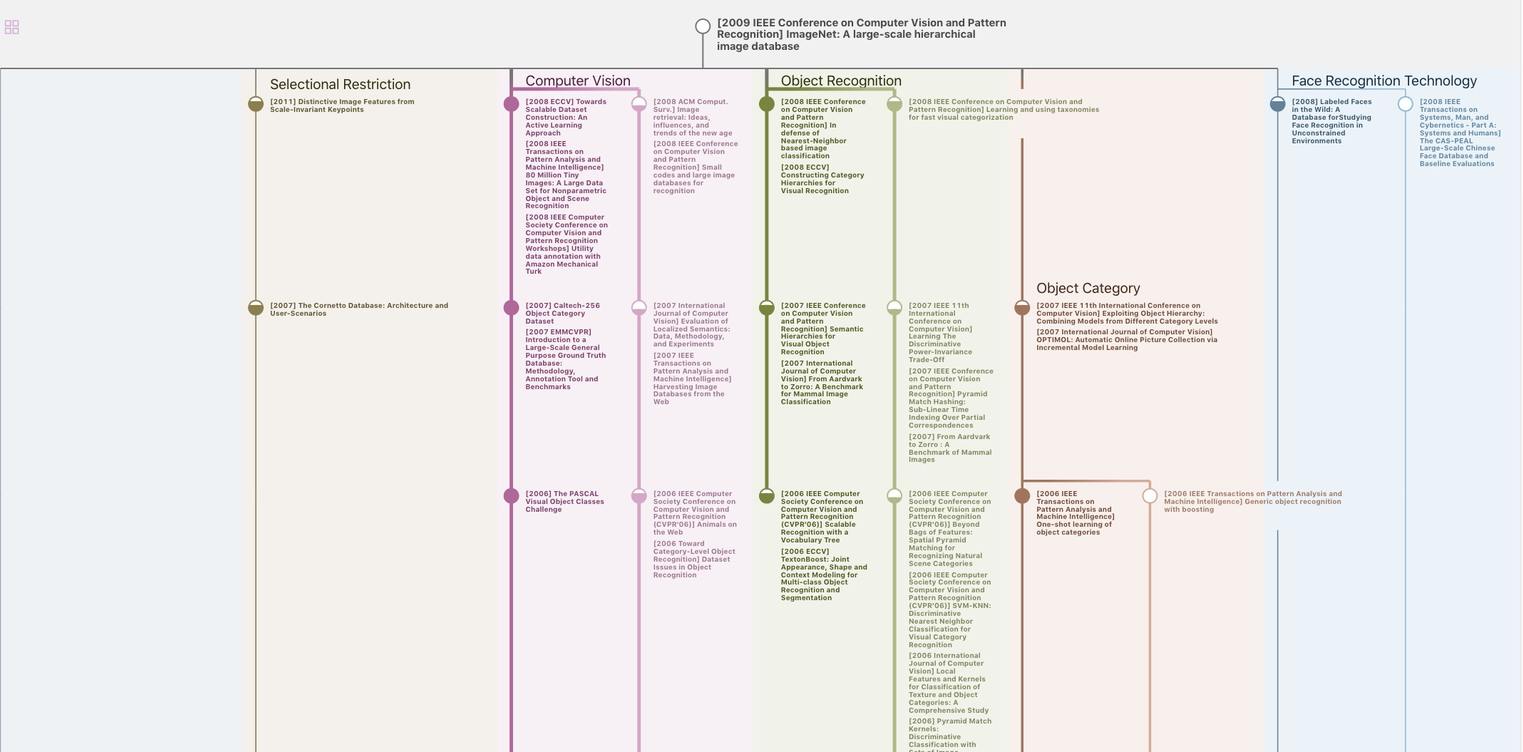
生成溯源树,研究论文发展脉络
Chat Paper
正在生成论文摘要