Sign constraints on feature weights improve a joint model of word segmentation and phonology.
HLT-NAACL(2015)
摘要
This paper describes a joint model of word segmentation and phonological alternations, which takes unsegmented utterances as input and infers word segmentations and underlying phonological representations. The model is a Maximum Entropy or log-linear model, which can express a probabilistic version of Opti- mality Theory (OT; Prince and Smolensky (2004)), a standard phonological framework. The features in our model are inspired by OTu0027s Markedness and Faithfulness constraints. Fol- lowing the OT principle that such features in- dicate violations, we require their weights to be non-positive. We apply our model to a modified version of the Buckeye corpus (Pitt et al., 2007) in which the only phonological alternations are deletions of word-final /d/ and /t/ segments. The model sets a new state-of- the-art for this corpus for word segmentation, identification of underlying forms, and identi- fication of /d/ and /t/ deletions. We also show that the OT-inspired sign constraints on fea- ture weights are crucial for accurate identifi- cation of deleted /d/s; without them our model posits approximately 10 times more deleted underlying /d/s than appear in the manually annotated data.
更多查看译文
关键词
word segmentation,phonology,feature weights,sign
AI 理解论文
溯源树
样例
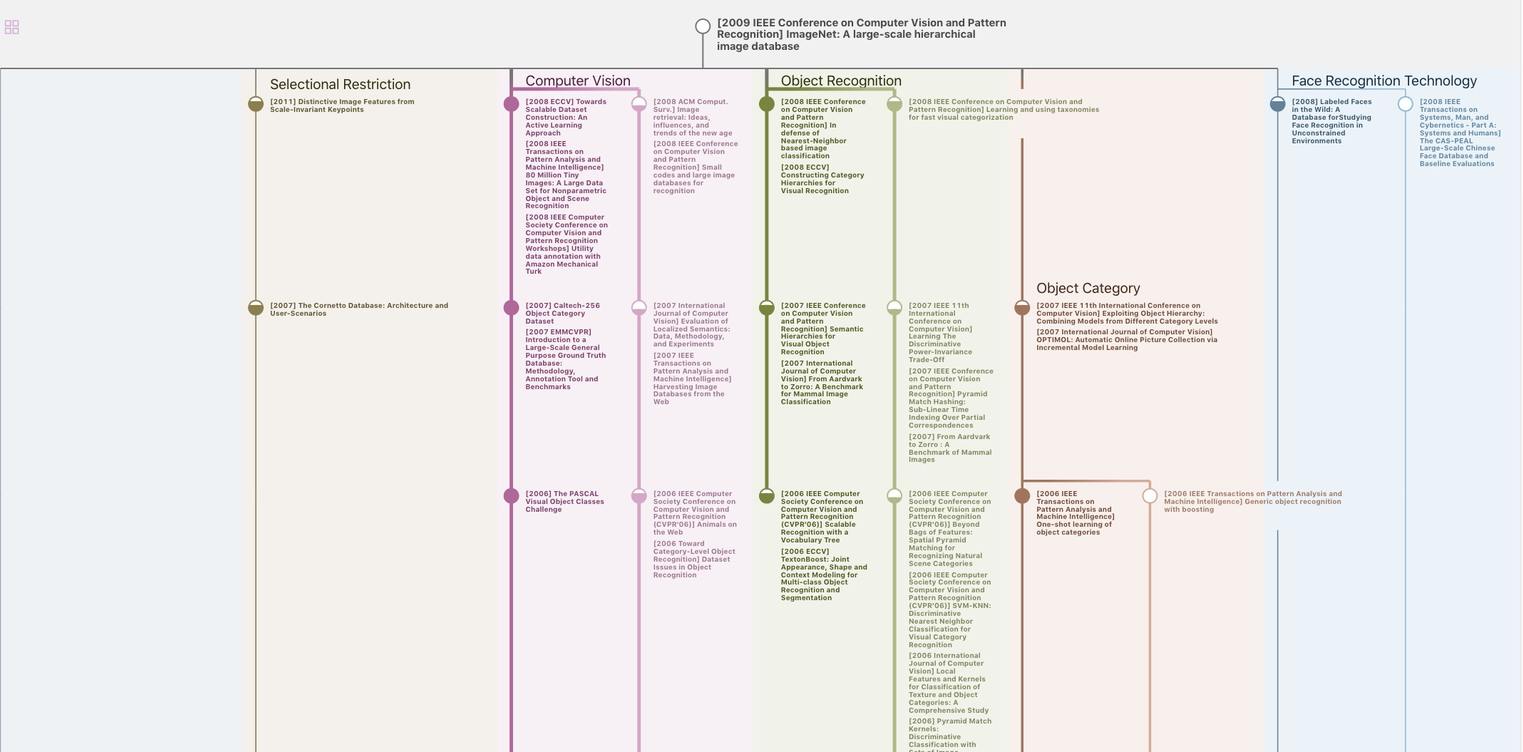
生成溯源树,研究论文发展脉络
Chat Paper
正在生成论文摘要