Parallel Nonlinear Discriminant Feature Extraction for Face and Handwritten Digit Recognition.
BIOMETRIC RECOGNITION, CCBR 2015(2015)
摘要
For recognition tasks with large amounts of data, the nonlinear discriminant feature extraction technique often suffers from large computational burden. Although some nonlinear accelerating methods have been presented, how to greatly reduce computing time and simultaneously keep favorable recognition result is still challenging. In this paper, we introduce parallel computing into nonlinear subspace learning and build a parallel nonlinear discriminant feature extraction framework. We firstly design a random non-overlapping equal data division strategy to divide the whole training sample set into several subsets and assign each computational node a subset. Then we separately learn nonlinear discriminant subspaces from these subsets without mutual communications, and finally select the most appropriate subspace for classification. Under this framework, we propose a novel nonlinear subspace learning approach, i.e., parallel nonlinear discriminant analysis(PNDA). Experimental results on three public face and handwritten digit image databases demonstrate the efficiency and effectiveness of the proposed approach.
更多查看译文
关键词
Parallel nonlinear discriminant feature extraction framework,PNDA,Face and handwritten digit recognition
AI 理解论文
溯源树
样例
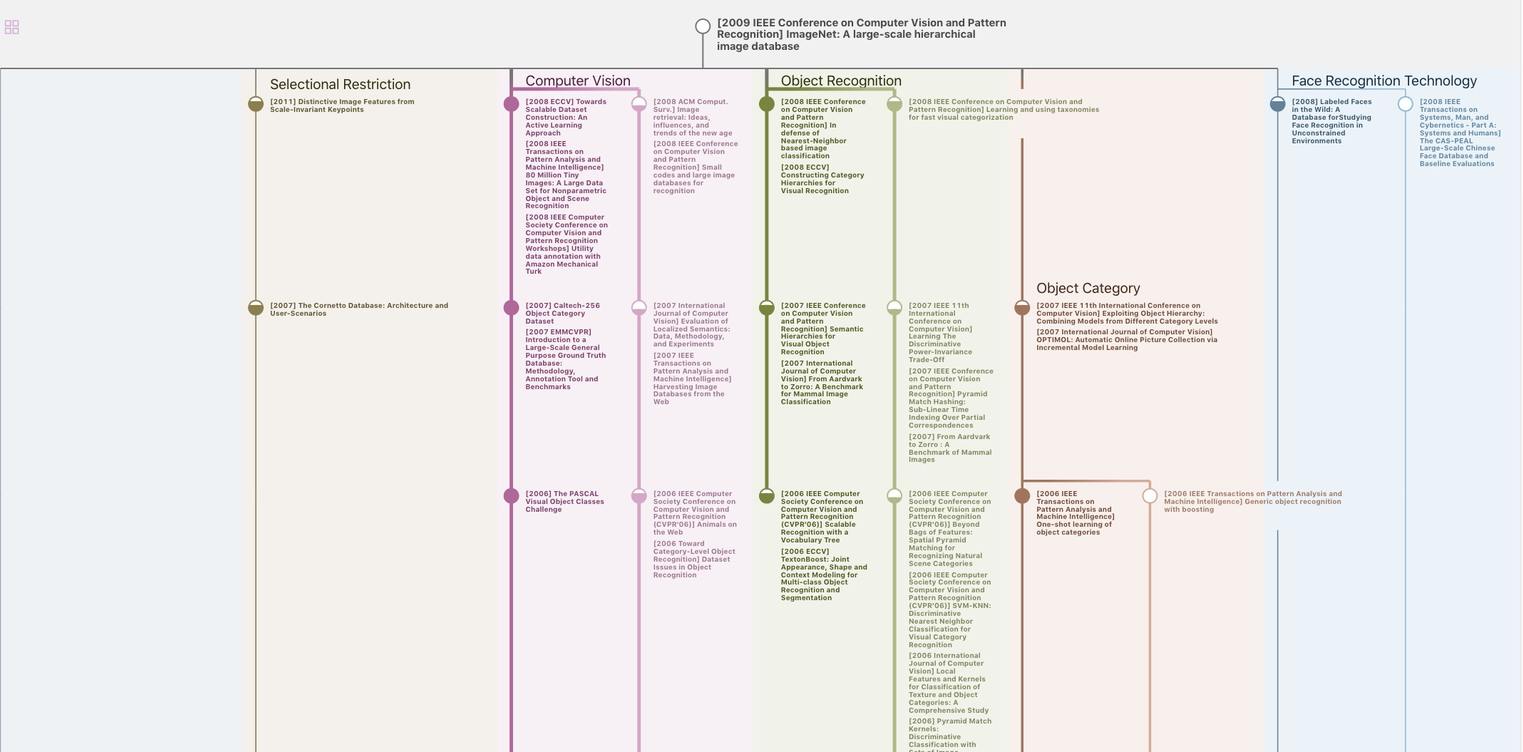
生成溯源树,研究论文发展脉络
Chat Paper
正在生成论文摘要