Integrating Physics-Based Prediction With Semantic Plan Execution Monitoring
2015 IEEE/RSJ INTERNATIONAL CONFERENCE ON INTELLIGENT ROBOTS AND SYSTEMS (IROS)(2015)
摘要
Real-world robotic systems have to perform reliably in uncertain and dynamic environments. State-of-the-art cognitive robotic systems use an abstract symbolic representation of the real world for high-level reasoning. Some aspects of the world, such as object dynamics, are inherently difficult to capture in an abstract symbolic form, yet they influence whether the executed action will succeed or fail. This paper presents an integrated system that uses a physics-based simulation to predict robot action results and durations, combined with a Hierarchical Task Network (HTN) planner and semantic execution monitoring. We describe a fully integrated system in which a Semantic Execution Monitor (SEM) uses information from the planning domain to perform functional imagination. Based on information obtained from functional imagination, the robot control system decides whether it is necessary to adapt the plan currently being executed. As a proof of concept, we demonstrate a PR2 able to carry tall objects on a tray without the objects toppling. Our approach achieves this by simulating robot and object dynamics. A validation shows that robot action results in simulation can be transferred to the real world. The system improves on state-of-the-art AI plan-based systems by feeding simulated prediction results back into the execution system.
更多查看译文
关键词
execution system,AI plan-based systems,object dynamic simulation,robot dynamic simulation,robot control system,functional imagination,SEM,semantic execution monitoring,HTN planner,hierarchical task network planner,robot action prediction,physics-based simulation,integrated system,abstract symbolic form,object dynamics,high-level reasoning,abstract symbolic representation,cognitive robotic systems,dynamic environment,uncertain environment,real-world robotic systems,semantic plan execution monitoring,physics-based prediction
AI 理解论文
溯源树
样例
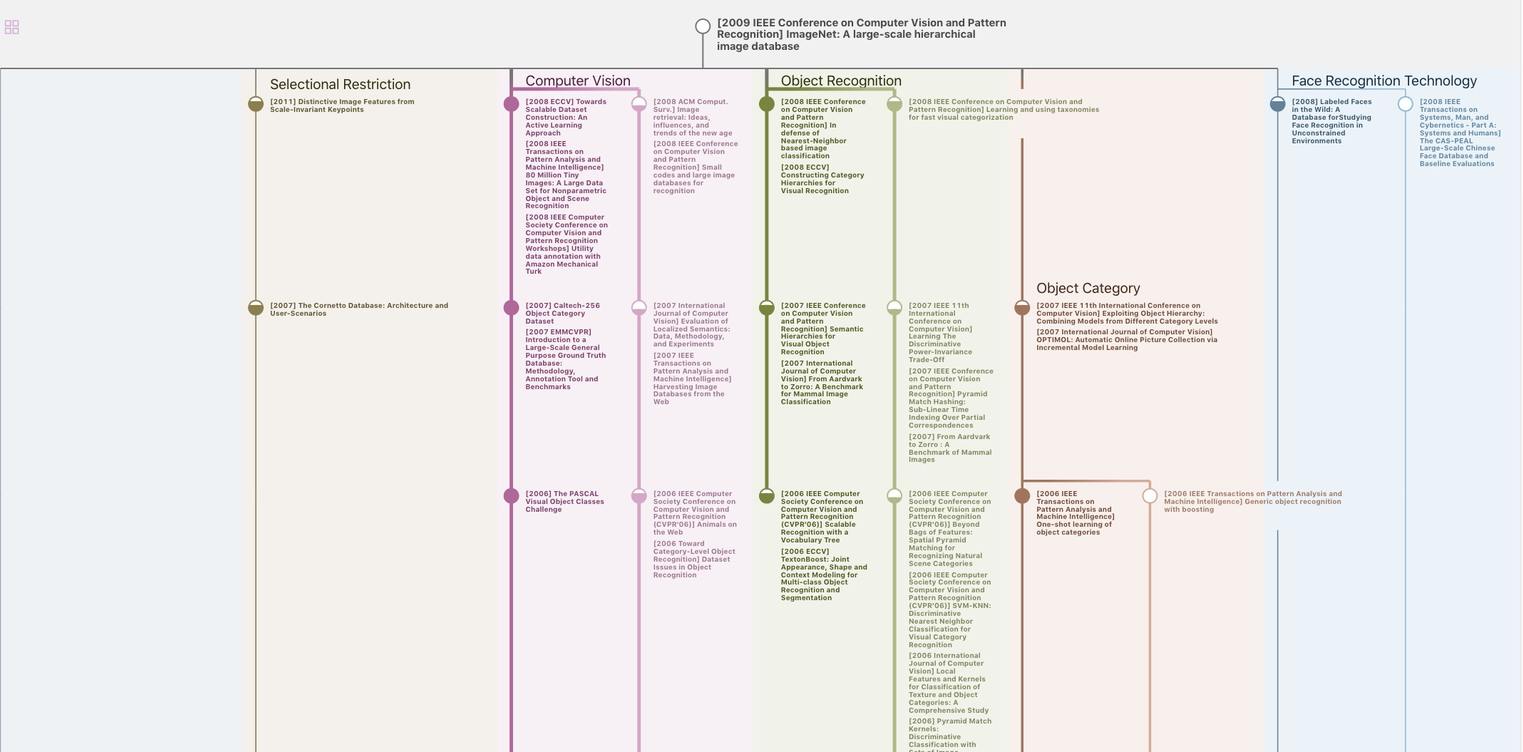
生成溯源树,研究论文发展脉络
Chat Paper
正在生成论文摘要