Performance Comparison of Ant Colony Algorithms for the Scheduling of Steel Production Lines.
GECCO '15: Genetic and Evolutionary Computation Conference Madrid Spain July, 2015(2015)
Abstract
Swarm Intelligence metaheuristics, and among them Ant Colony Optimization (ACO), have been successfully applied worldwide to solve multiple examples of combinatorial NP-hard problems, giving good solutions in a reasonable period of time (an essential requirement in real life applications). Our company is dedicated to produce steel, being the biggest steelmaker in the World. The impact of a good sequencing in our daily production results is critical. Sequencing requires to take into consideration the most relevant parameters that influence along the material transformation process, being this a really difficult task to do manually or with other traditional methods. After the successful use of the Ant System (AS) algorithm to sequence several of our Galvanizing production lines, we have studied the potential benefit of moving from the classical AS algorithm to an Ant Colony System (ACS), a promising variation of the ACO metaheuristics family. In this paper, we present the work done in order to apply the ACO techniques to an industrial problem. More specifically, we exhibit a comparison between AS and ACS variants, the results obtained and the conclusions drawn from such comparison.
MoreTranslated text
Key words
ant colony algorithms,scheduling
AI Read Science
Must-Reading Tree
Example
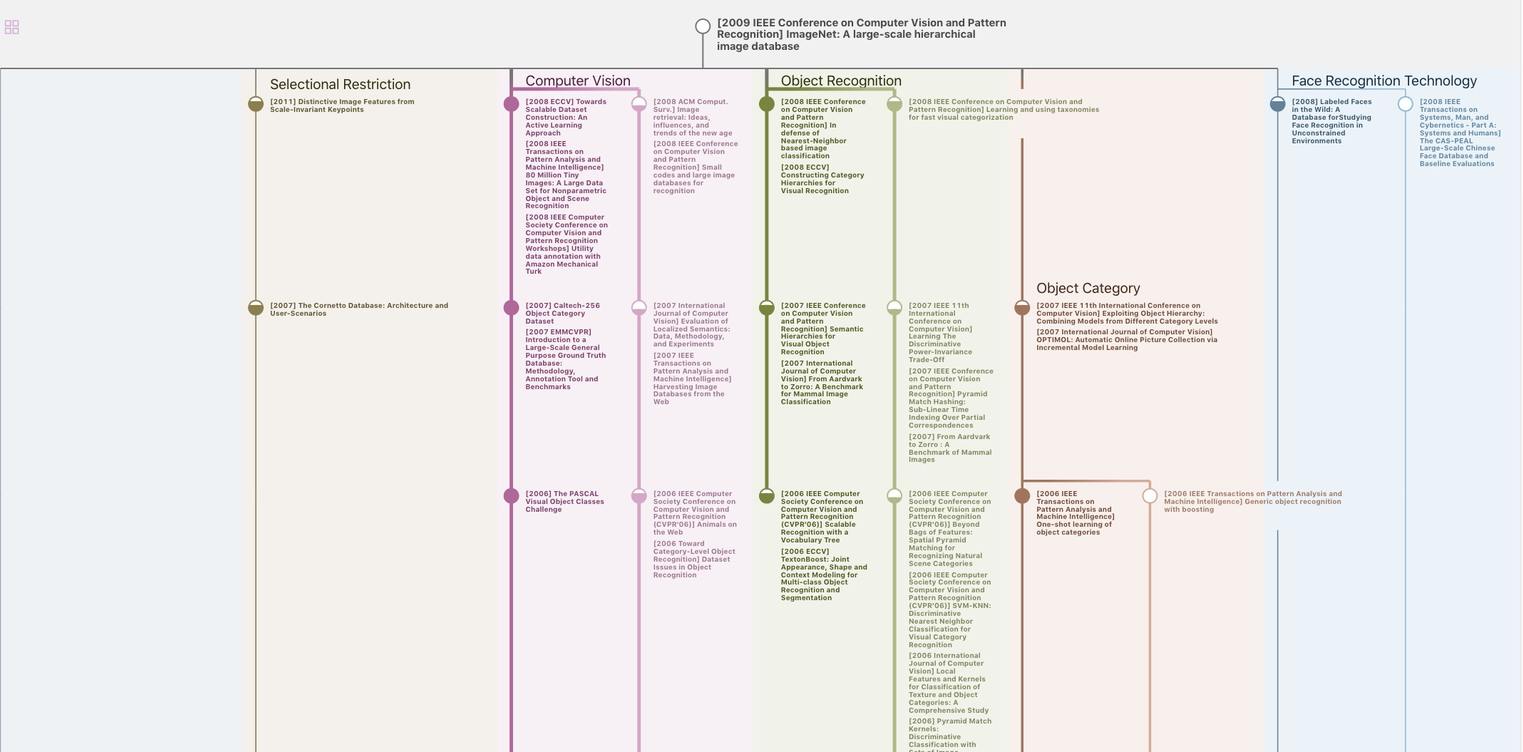
Generate MRT to find the research sequence of this paper
Chat Paper
Summary is being generated by the instructions you defined