Texture Characterization Via Improved Deterministicwalks On Image-Generated Complex Network
2015 IEEE International Conference on Image Processing (ICIP)(2015)
摘要
This work presents a novel approach for texture based image description, by representing an image as a complex network and applying an enhanced deterministic partially self-avoiding walks algorithm on a transformed image generated from the network's statistics, focusing on extraction of walk shape to build a feature vector to competently characterize texture. A significant improvement to the deterministic walks method proposed in this work is to create a complex network from an image and perform walks on the network's nodes' degrees, instead of on the intensity of the original image's pixels, as usual. Another meaningful innovation is in the way information is acquired from the walks: instead of using walk sizes or demanding fractal dimension computations, the proposed method derives shape information from a walk direction histogram. Experiments using the proposed method for texture classification on several widespread datasets show that the proposed method not only manages to reduce feature vector size but also improves correct classification rates compared to other state-of-the-art methods.
更多查看译文
关键词
Texture characterization,complex networks,deterministic walks
AI 理解论文
溯源树
样例
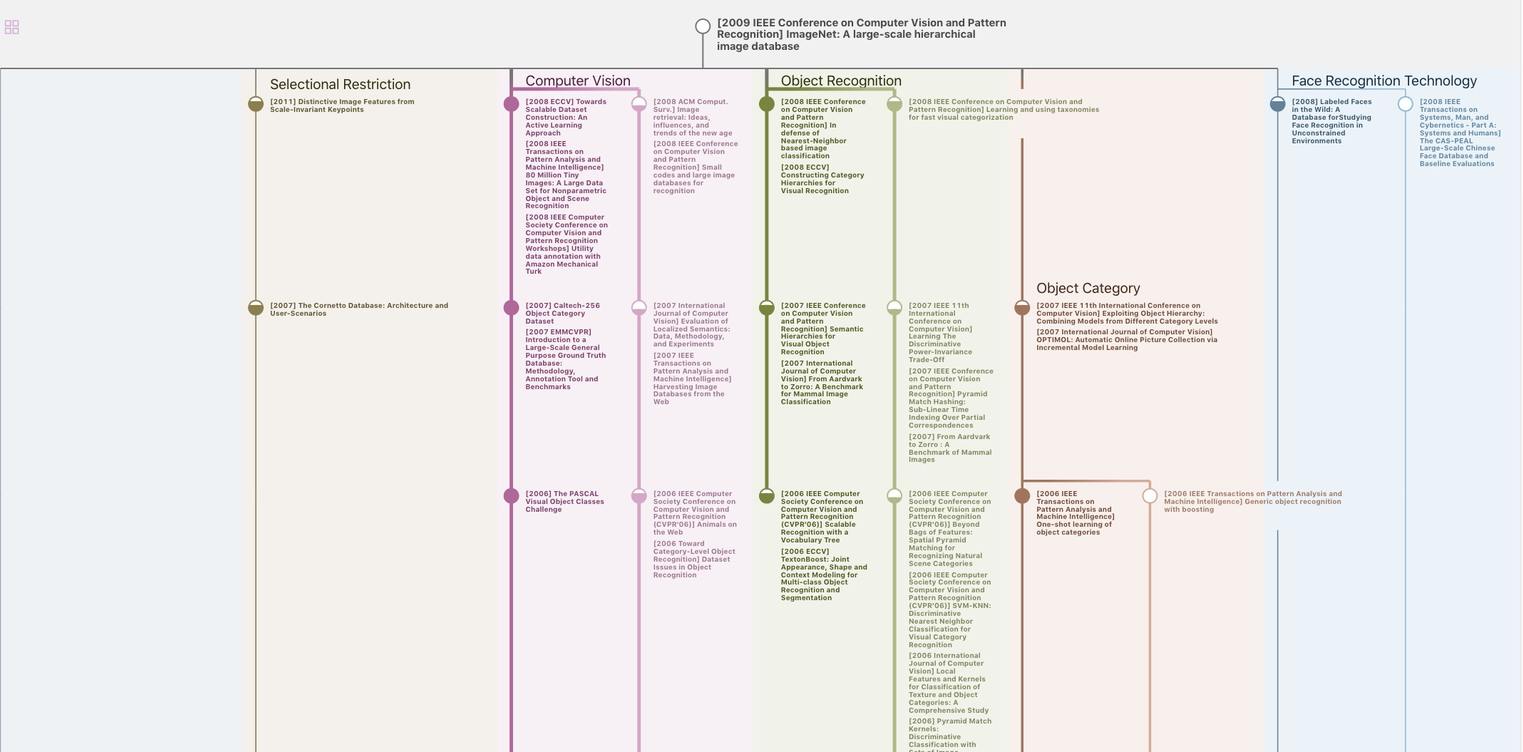
生成溯源树,研究论文发展脉络
Chat Paper
正在生成论文摘要